Deep cross-modal subspace clustering with Contrastive Neighbour Embedding
NEUROCOMPUTING(2024)
摘要
Deep cross -modal clustering has been developing rapidly and attracted considerable attention in recent years. It aims to pursue a consistent subspace from different modalities with deep neural networks and achieves remarkable clustering performance. However, most existing methods do not simultaneously consider the inherently diverse information of each modality and the neighbour geometric structure over cross -modal data, which inevitably degrades the cluster structure revealed by the common subspace. In this paper, we propose a novel method named Deep Cross -Modal Subspace Clustering with Contrastive Neighbour Embedding (DCSC-CNE) to address the above challenge. DCSC-CNE maintains the inherent independence of each modality while concurrently uncovering consistent information across diverse modalities. In addition, we introduce a contrastive neighbour graph in the proposed deep cross -modal subspace clustering framework by performing contrastive learning between positive and negative samples, to highlight the underlying neighbour geometry of the original data and learn discriminative latent (subspace) representations. In this way, DCSC-CNE integrates the consistent-inherent learning and the contrastive neighbour embedding into a unified deep learning framework. Experimental results demonstrate that the proposed method can significantly improve the cross -modal subspace clustering performance compared with state -of -the -art methods on six benchmark datasets.
更多查看译文
关键词
Cross-modal clustering,Contrastive learning,Consistent learning
AI 理解论文
溯源树
样例
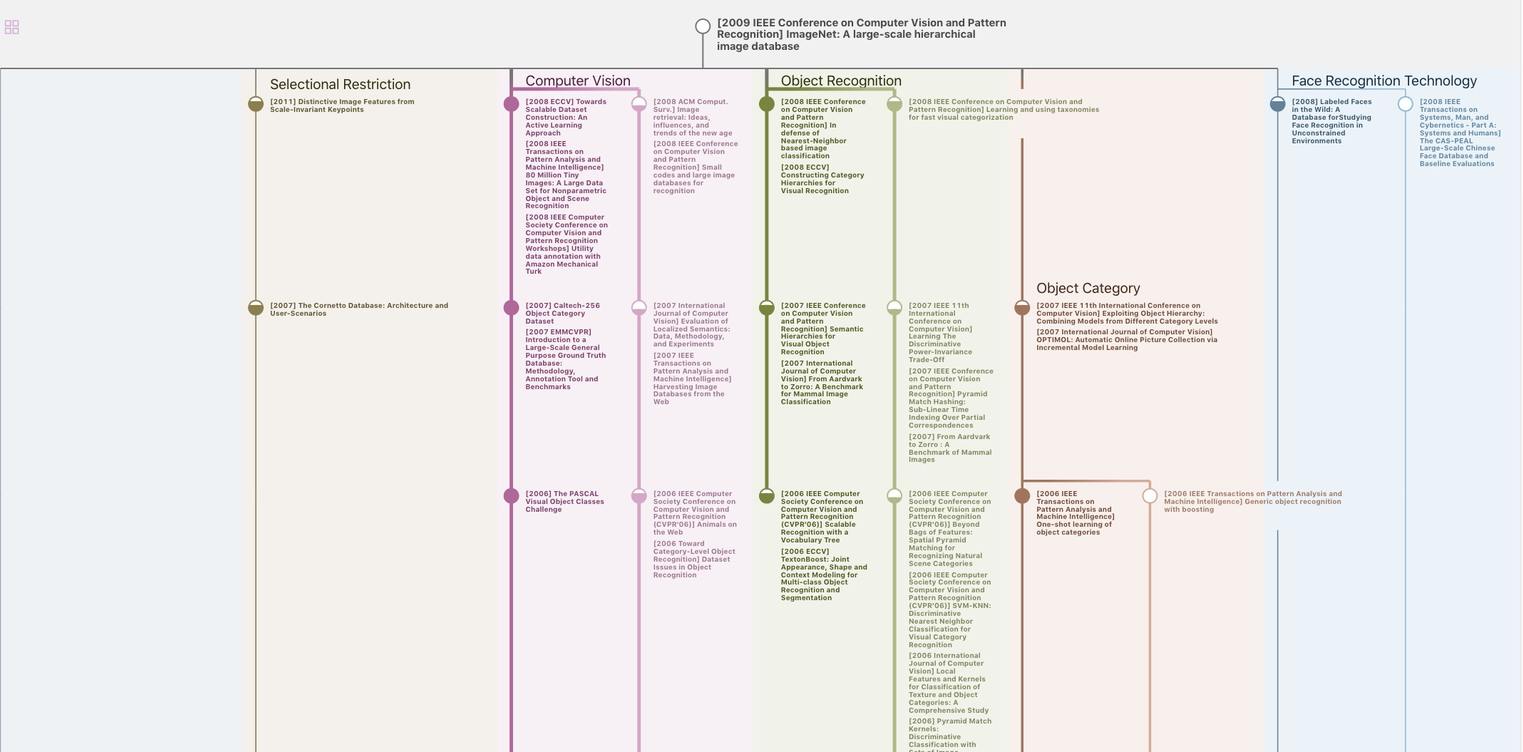
生成溯源树,研究论文发展脉络
Chat Paper
正在生成论文摘要