Bearing fault diagnosis via fusing small samples and training multi-state Siamese neural networks
NEUROCOMPUTING(2024)
摘要
Recently, deep learning techniques have been widely applied to fault diagnosis due to their outstanding feature extraction abilities. The success of deep -learning -based fault diagnosis methods is highly dependent on the quantity and quality of the training data. In practical scenarios, it is challenging to obtain sufficient high -quality training data for fault diagnosis tasks due to complex environments, which would affect the effectiveness of the deep learning methods. In this paper, a new fault diagnosis method is proposed for motor bearing fault diagnosis under small samples. The Siamese neural networks (SNNs) are employed to extract the fault features. A multi -stage training strategy is proposed to train the SNNs with the aim to prevent the training stagnation problem and handle the small sample problem. A multi -source feature fusion network is developed to make full use of the multi -source sensor data by fusing the extracted fault features for further fault diagnosis. The proposed method is applied to motor bearing fault diagnosis on two real -world datasets. Experimental results demonstrate the effectiveness and feasibility of the introduced method for motor bearing fault diagnosis under small samples.
更多查看译文
关键词
Fault diagnosis,Siamese neural networks,Small sample,Multi-source sensor,Motor rolling bearing
AI 理解论文
溯源树
样例
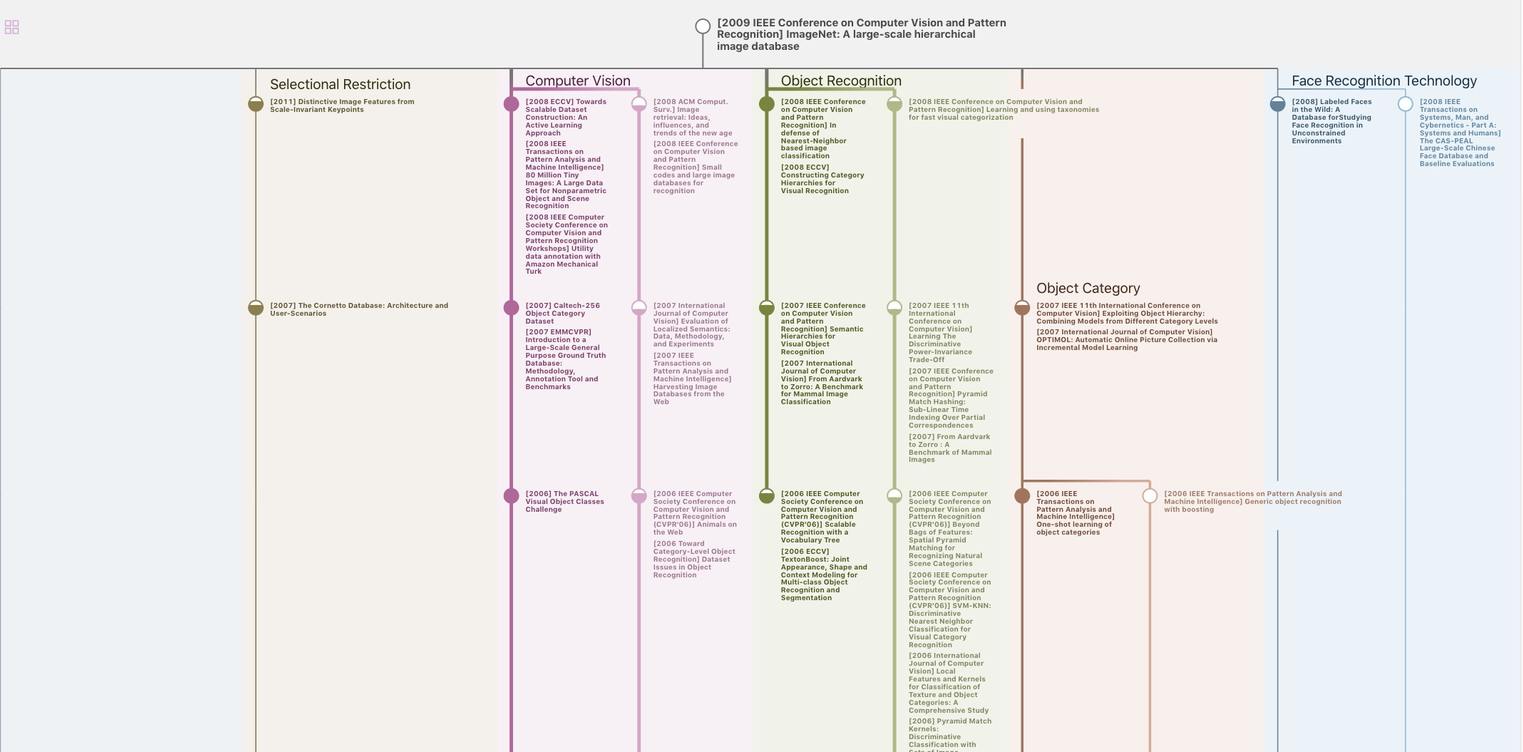
生成溯源树,研究论文发展脉络
Chat Paper
正在生成论文摘要