Emulated Disalignment: Safety Alignment for Large Language Models May Backfire!
arxiv(2024)
摘要
Large language models (LLMs) need to undergo safety alignment to ensure safe
conversations with humans. However, in this work, we introduce an
inference-time attack framework, demonstrating that safety alignment can also
unintentionally facilitate harmful outcomes under adversarial manipulation.
This framework, named Emulated Disalignment (ED), adversely combines a pair of
open-source pre-trained and safety-aligned language models in the output space
to produce a harmful language model without any training. Our experiments with
ED across three datasets and four model families (Llama-1, Llama-2, Mistral,
and Alpaca) show that ED doubles the harmfulness of pre-trained models and
outperforms strong baselines, achieving the highest harmful rate in 43 out of
48 evaluation subsets by a large margin. Crucially, our findings highlight the
importance of reevaluating the practice of open-sourcing language models even
after safety alignment.
更多查看译文
AI 理解论文
溯源树
样例
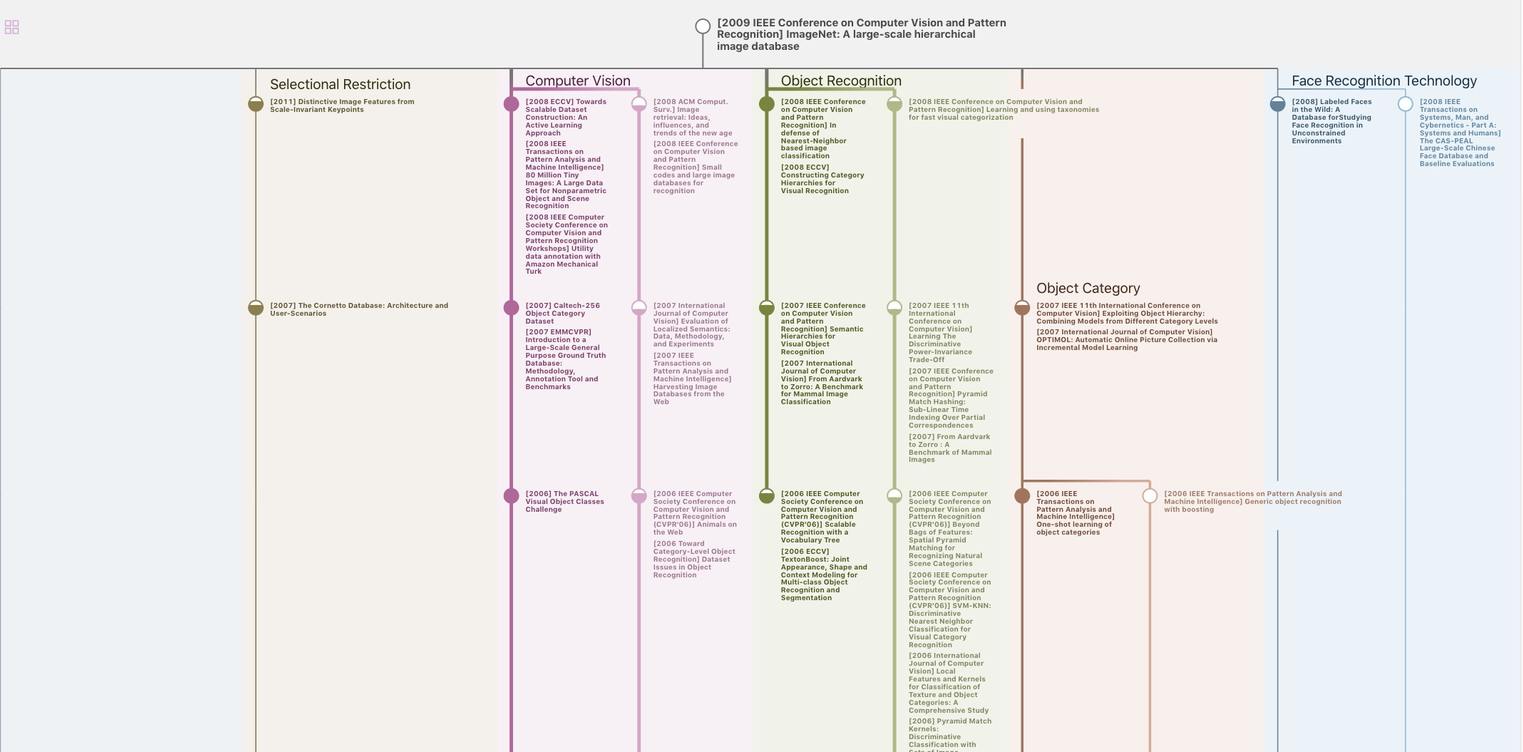
生成溯源树,研究论文发展脉络
Chat Paper
正在生成论文摘要