ROSE Doesn't Do That: Boosting the Safety of Instruction-Tuned Large Language Models with Reverse Prompt Contrastive Decoding
CoRR(2024)
摘要
With the development of instruction-tuned large language models (LLMs),
improving the safety of LLMs has become more critical. However, the current
approaches for aligning the LLMs output with expected safety usually require
substantial training efforts, e.g., high-quality safety data and expensive
computational resources, which are costly and inefficient. To this end, we
present reverse prompt contrastive decoding (ROSE), a simple-yet-effective
method to directly boost the safety of existing instruction-tuned LLMs without
any additional training. The principle of ROSE is to improve the probability of
desired safe output via suppressing the undesired output induced by the
carefully-designed reverse prompts. Experiments on 6 safety and 2
general-purpose tasks show that, our ROSE not only brings consistent and
significant safety improvements (up to +13.8
instruction-tuned LLMs, but also benefits the general-purpose ability of LLMs.
In-depth analyses explore the underlying mechanism of ROSE, and reveal when and
where to use it.
更多查看译文
AI 理解论文
溯源树
样例
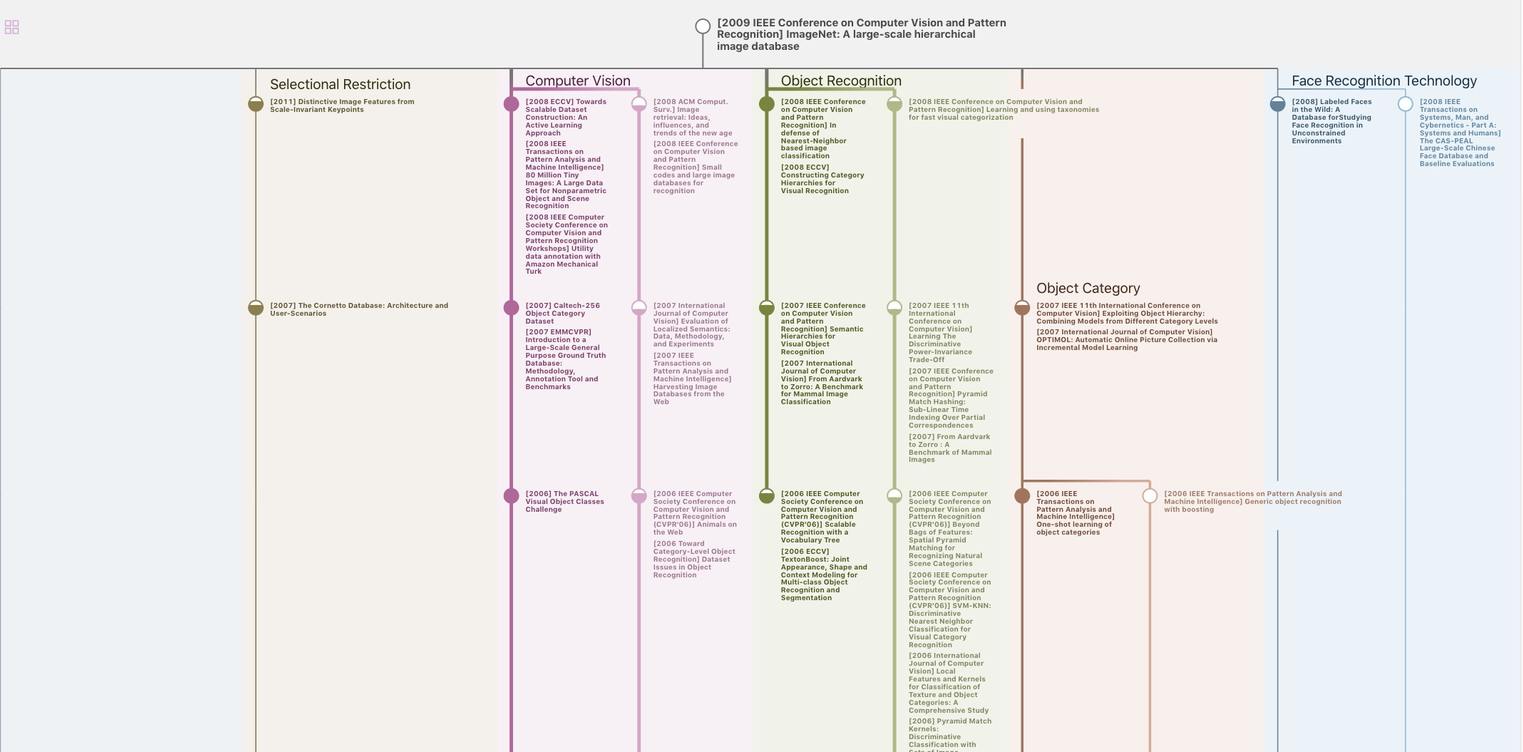
生成溯源树,研究论文发展脉络
Chat Paper
正在生成论文摘要