Easy as ABCs: Unifying Boltzmann Q-Learning and Counterfactual Regret Minimization
CoRR(2024)
摘要
We propose ABCs (Adaptive Branching through Child stationarity), a
best-of-both-worlds algorithm combining Boltzmann Q-learning (BQL), a classic
reinforcement learning algorithm for single-agent domains, and counterfactual
regret minimization (CFR), a central algorithm for learning in multi-agent
domains. ABCs adaptively chooses what fraction of the environment to explore
each iteration by measuring the stationarity of the environment's reward and
transition dynamics. In Markov decision processes, ABCs converges to the
optimal policy with at most an O(A) factor slowdown compared to BQL, where A is
the number of actions in the environment. In two-player zero-sum games, ABCs is
guaranteed to converge to a Nash equilibrium (assuming access to a perfect
oracle for detecting stationarity), while BQL has no such guarantees.
Empirically, ABCs demonstrates strong performance when benchmarked across
environments drawn from the OpenSpiel game library and OpenAI Gym and exceeds
all prior methods in environments which are neither fully stationary nor fully
nonstationary.
更多查看译文
AI 理解论文
溯源树
样例
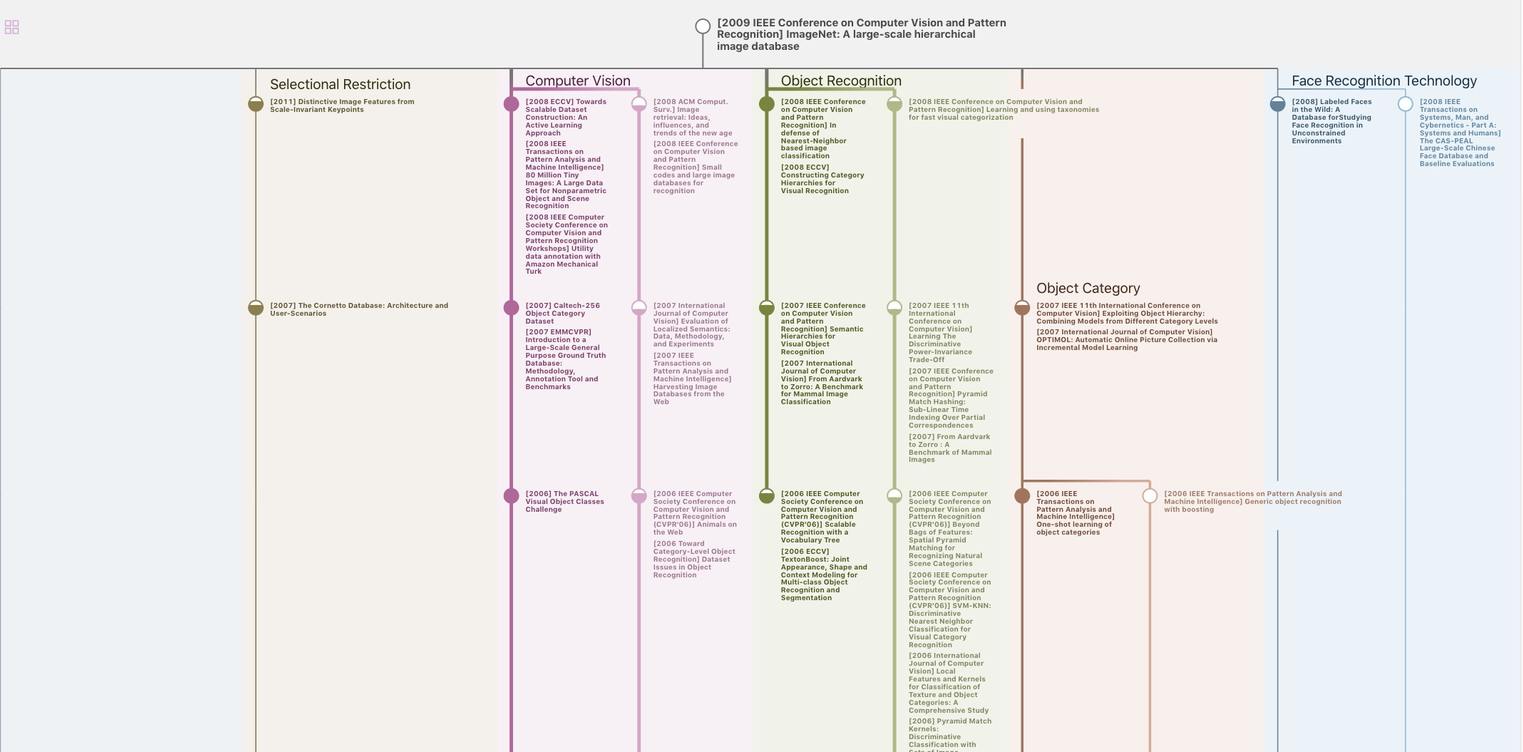
生成溯源树,研究论文发展脉络
Chat Paper
正在生成论文摘要