Generative Kaleidoscopic Networks
CoRR(2024)
摘要
We discovered that the Deep ReLU networks (or Multilayer Perceptron
architecture) demonstrate an 'over-generalization' phenomenon. That is, the
output values for the inputs that were not seen during training are mapped
close to the output range that were observed during the learning process. In
other words, the MLP learns a many-to-one mapping and this effect is more
prominent as we increase the number of layers or depth of the MLP. We utilize
this property of Deep ReLU networks to design a dataset kaleidoscope, termed as
'Generative Kaleidoscopic Networks'. Briefly, if we learn a MLP to map from
input x∈ℝ^D to itself f_𝒩(x)→ x, the
'Kaleidoscopic sampling' procedure starts with a random input noise
z∈ℝ^D and recursively applies f_𝒩(⋯
f_𝒩(z)⋯ ). After a burn-in period duration, we start observing
samples from the input distribution and we found that deeper the MLP, higher is
the quality of samples recovered. Scope: We observed this phenomenon to various
degrees for the other deep learning architectures like CNNs, Transformers
U-Nets and we are currently investigating them further.
更多查看译文
AI 理解论文
溯源树
样例
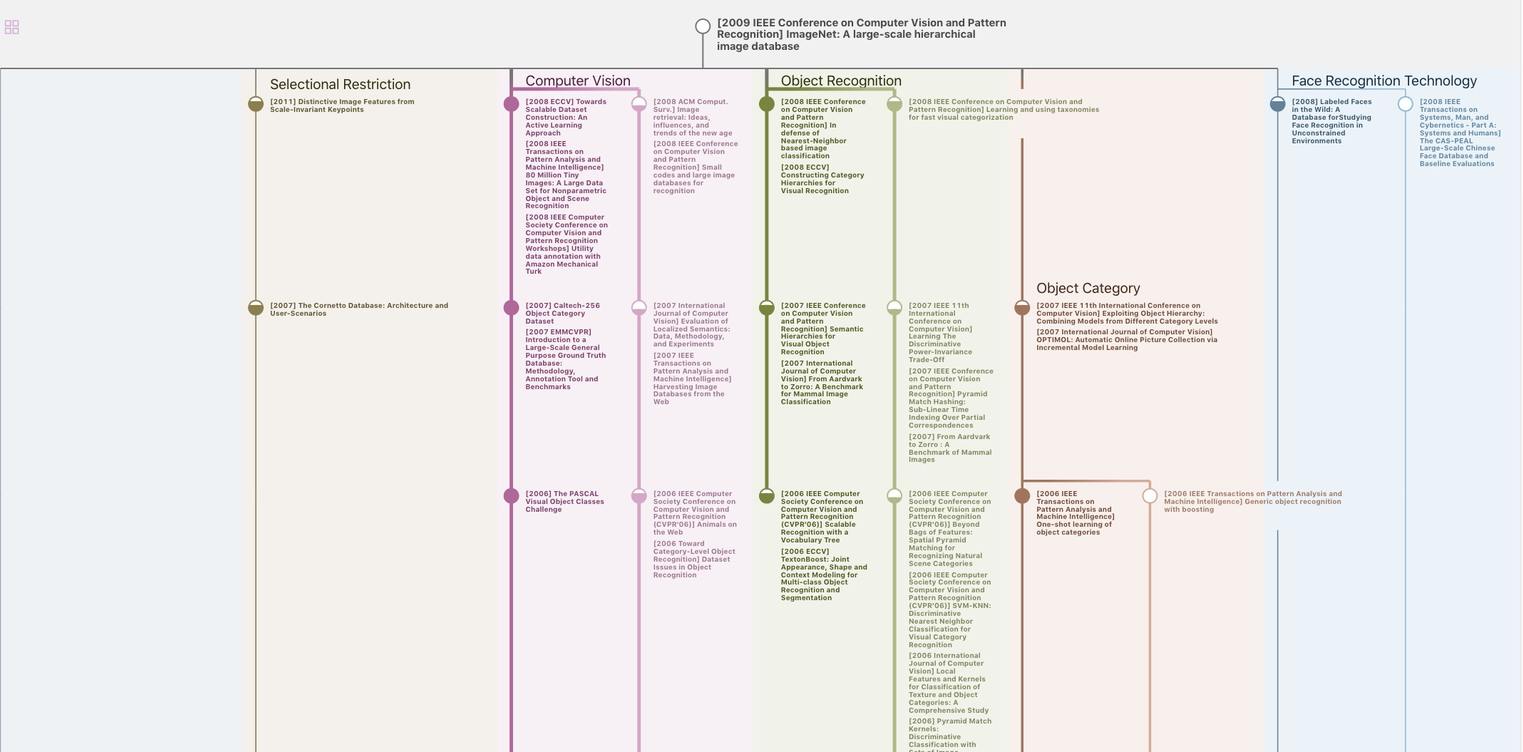
生成溯源树,研究论文发展脉络
Chat Paper
正在生成论文摘要