Hierarchical Prior-Based Super Resolution for Point Cloud Geometry Compression.
IEEE transactions on image processing : a publication of the IEEE Signal Processing Society(2024)
摘要
The Geometry-based Point Cloud Compression (G-PCC) has been developed by the Moving Picture Experts Group to compress point clouds efficiently. Nevertheless, in its lossy mode, the reconstructed point cloud by G-PCC often suffers from noticeable distortions due to naïve geometry quantization (i.e., grid downsampling). This paper proposes a hierarchical prior-based super resolution method for point cloud geometry compression. The content-dependent hierarchical prior is constructed at the encoder side, which enables coarse-to-fine super resolution of the point cloud geometry at the decoder side. A more accurate prior generally yields improved reconstruction performance, albeit at the cost of increased bits required to encode this piece of side information. Our experiments on the MPEG Cat1A dataset demonstrate substantial Bjøntegaard-delta bitrate savings, surpassing the performance of the octree-based and trisoup-based G-PCC v14. We provide our implementations for reproducible research at https://github.com/lidq92/mpeg-pcc-tmc13.
更多查看译文
关键词
Point cloud geometry compression,hierarchical prior,coarse-to-fine super resolution
AI 理解论文
溯源树
样例
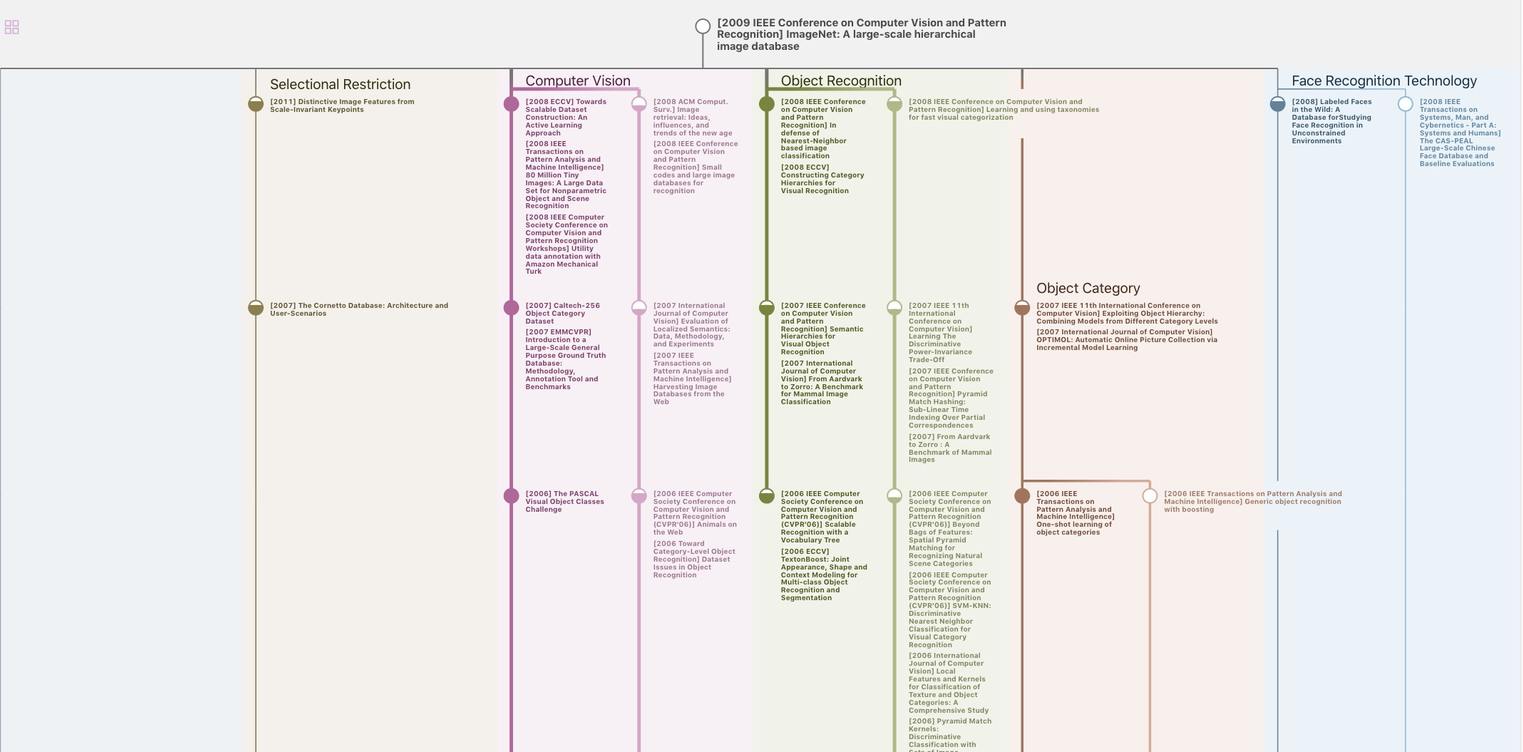
生成溯源树,研究论文发展脉络
Chat Paper
正在生成论文摘要