Cosmological multifield emulator
arxiv(2024)
摘要
We demonstrate the use of deep network to learn the distribution of data from
state-of-the-art hydrodynamic simulations of the CAMELS project. To this end,
we train a generative adversarial network to generate images composed of three
different channels that represent gas density (Mgas), neutral hydrogen density
(HI), and magnetic field amplitudes (B). We consider an unconstrained model and
another scenario where the model is conditioned on the matter density
Ω_ m and the amplitude of density fluctuations σ_8. We
find that the generated images exhibit great quality which is on a par with
that of data, visually. Quantitatively, we find that our model generates maps
whose statistical properties, quantified by probability distribution function
of pixel values and auto-power spectra, agree reasonably well with those of the
real maps. Moreover, the cross-correlations between fields in all maps produced
by the emulator are in good agreement with those of the real images, which
indicates that our model generates instances whose maps in all three channels
describe the same physical region. Furthermore, a CNN regressor, which has been
trained to extract Ω_ m and σ_8 from CAMELS multifield
dataset, recovers the cosmology from the maps generated by our conditional
model, achieving R^2 = 0.96 and 0.83 corresponding to Ω_ m and
σ_8 respectively. This further demonstrates the great capability of
the model to mimic CAMELS data. Our model can be useful for generating data
that are required to analyze the information from upcoming multi-wavelength
cosmological surveys.
更多查看译文
AI 理解论文
溯源树
样例
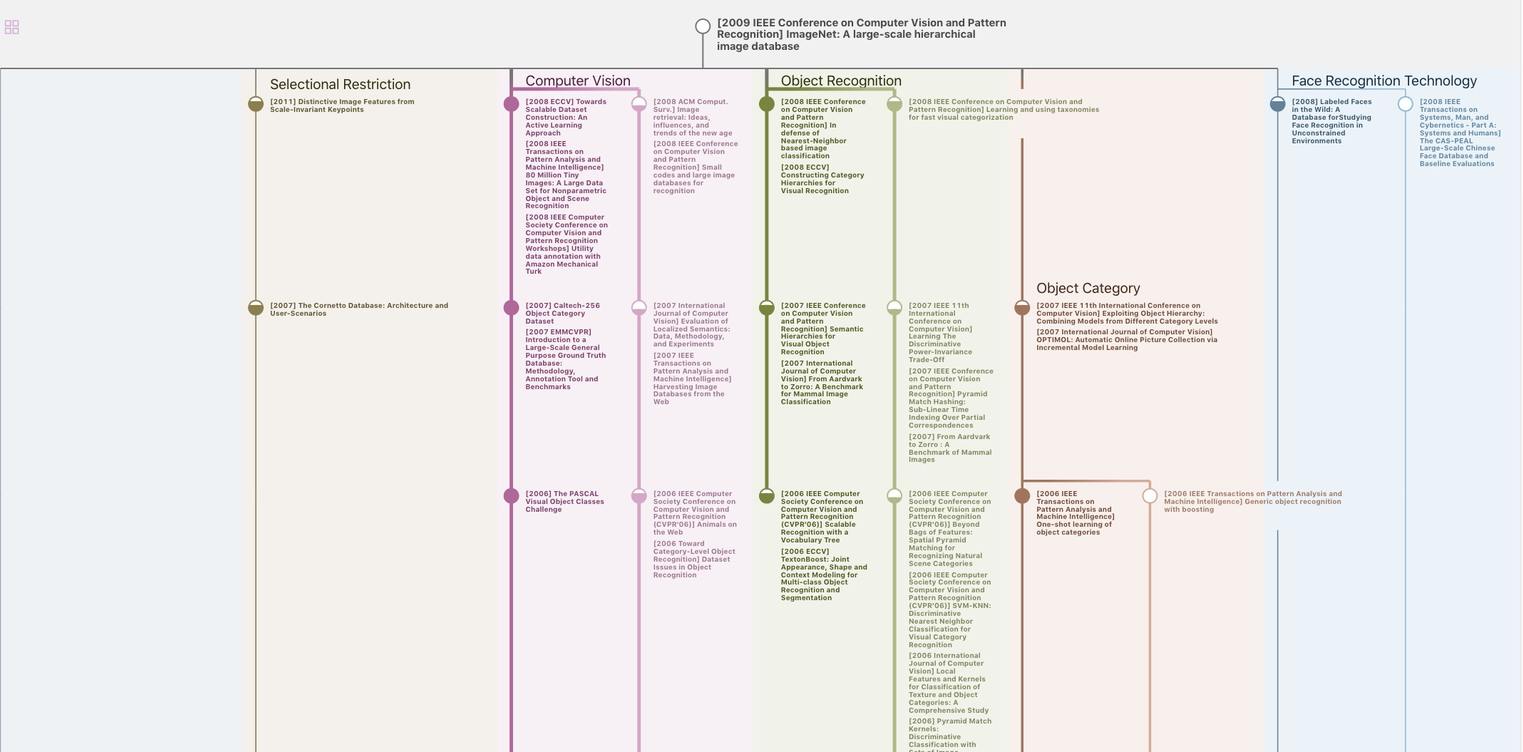
生成溯源树,研究论文发展脉络
Chat Paper
正在生成论文摘要