Individualized treatment rule characterization via a value function surrogate
BIOMETRICS(2024)
摘要
Precision medicine is a promising framework for generating evidence to improve health and health care. Yet, a gap persists between the ever-growing number of statistical precision medicine strategies for evidence generation and implementation in real-world clinical settings, and the strategies for closing this gap will likely be context-dependent. In this paper, we consider the specific context of partial compliance to wound management among patients with peripheral artery disease. Using a Gaussian process surrogate for the value function, we show the feasibility of using Bayesian optimization to learn optimal individualized treatment rules. Further, we expand beyond the common precision medicine task of learning an optimal individualized treatment rule to the characterization of classes of individualized treatment rules and show how those findings can be translated into clinical contexts.
更多查看译文
关键词
Gaussian process,individualized treatment rule,precision medicine,surrogate model,translational science
AI 理解论文
溯源树
样例
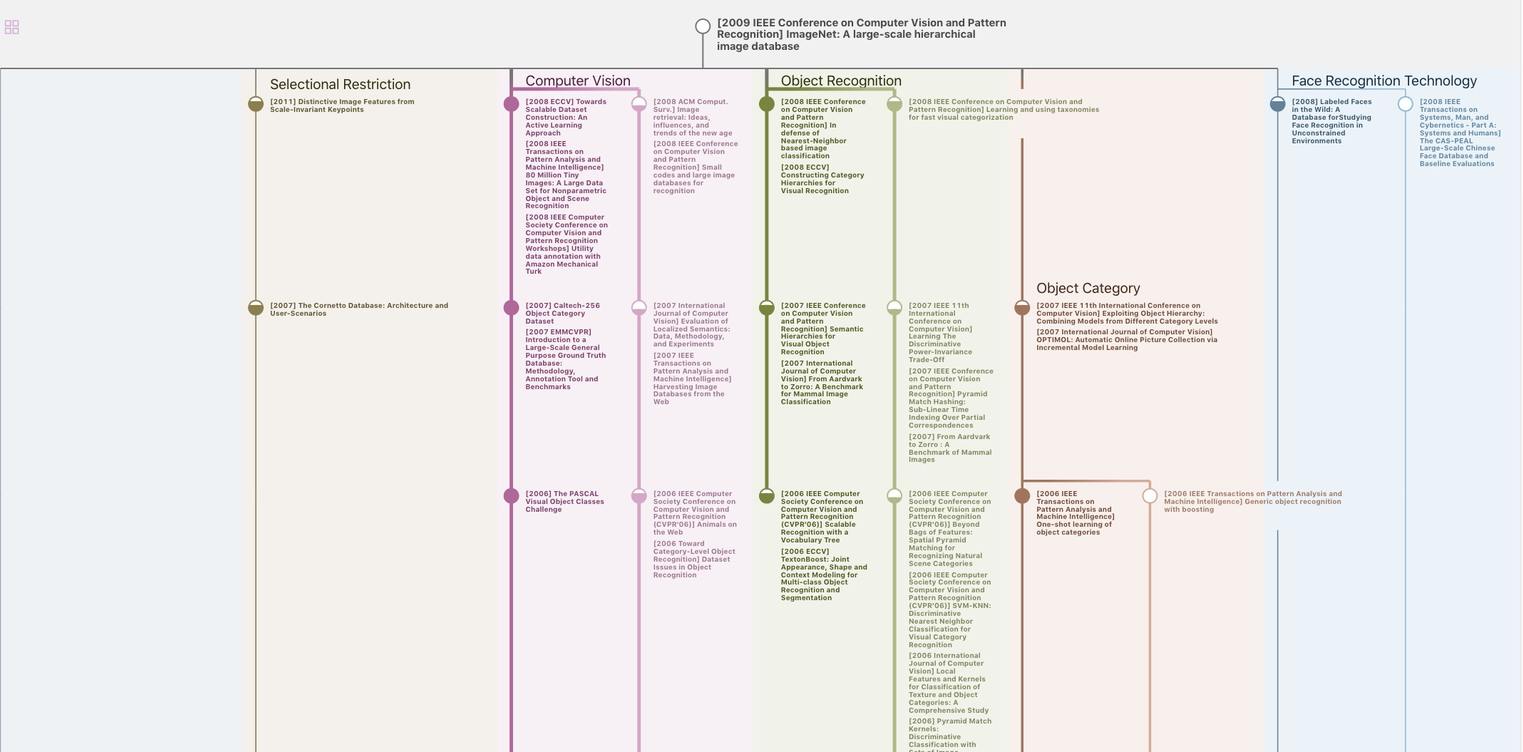
生成溯源树,研究论文发展脉络
Chat Paper
正在生成论文摘要