Multivariate lesion symptom mapping for predicting trajectories of recovery from aphasia
BRAIN COMMUNICATIONS(2023)
摘要
Individuals with post-stroke aphasia tend to recover their language to some extent; however, it remains challenging to reliably predict the nature and extent of recovery that will occur in the long term. The aim of this study was to quantitatively predict language outcomes in the first year of recovery from aphasia across multiple domains of language and at multiple timepoints post-stroke. We recruited 217 patients with aphasia following acute left hemisphere ischaemic or haemorrhagic stroke and evaluated their speech and language function using the Quick Aphasia Battery acutely and then acquired longitudinal follow-up data at up to three timepoints post-stroke: 1 month (n = 102), 3 months (n = 98) and 1 year (n = 74). We used support vector regression to predict language outcomes at each timepoint using acute clinical imaging data, demographic variables and initial aphasia severity as input. We found that similar to 60% of the variance in long-term (1 year) aphasia severity could be predicted using these models, with detailed information about lesion location importantly contributing to these predictions. Predictions at the 1- and 3-month timepoints were somewhat less accurate based on lesion location alone, but reached comparable accuracy to predictions at the 1-year timepoint when initial aphasia severity was included in the models. Specific subdomains of language besides overall severity were predicted with varying but often similar degrees of accuracy. Our findings demonstrate the feasibility of using support vector regression models with leave-one-out cross-validation to make personalized predictions about long-term recovery from aphasia and provide a valuable neuroanatomical baseline upon which to build future models incorporating information beyond neuroanatomical and demographic predictors. Levy et al. use machine learning to predict language outcomes across the first year of recovery from aphasia post-stroke, explaining nearly two-thirds of the variance in language outcomes and additionally demonstrating the importance of lesion location in making good predictions. See aphasia-friendly version of findings within! Graphical Abstract
更多查看译文
关键词
aphasia,stroke
AI 理解论文
溯源树
样例
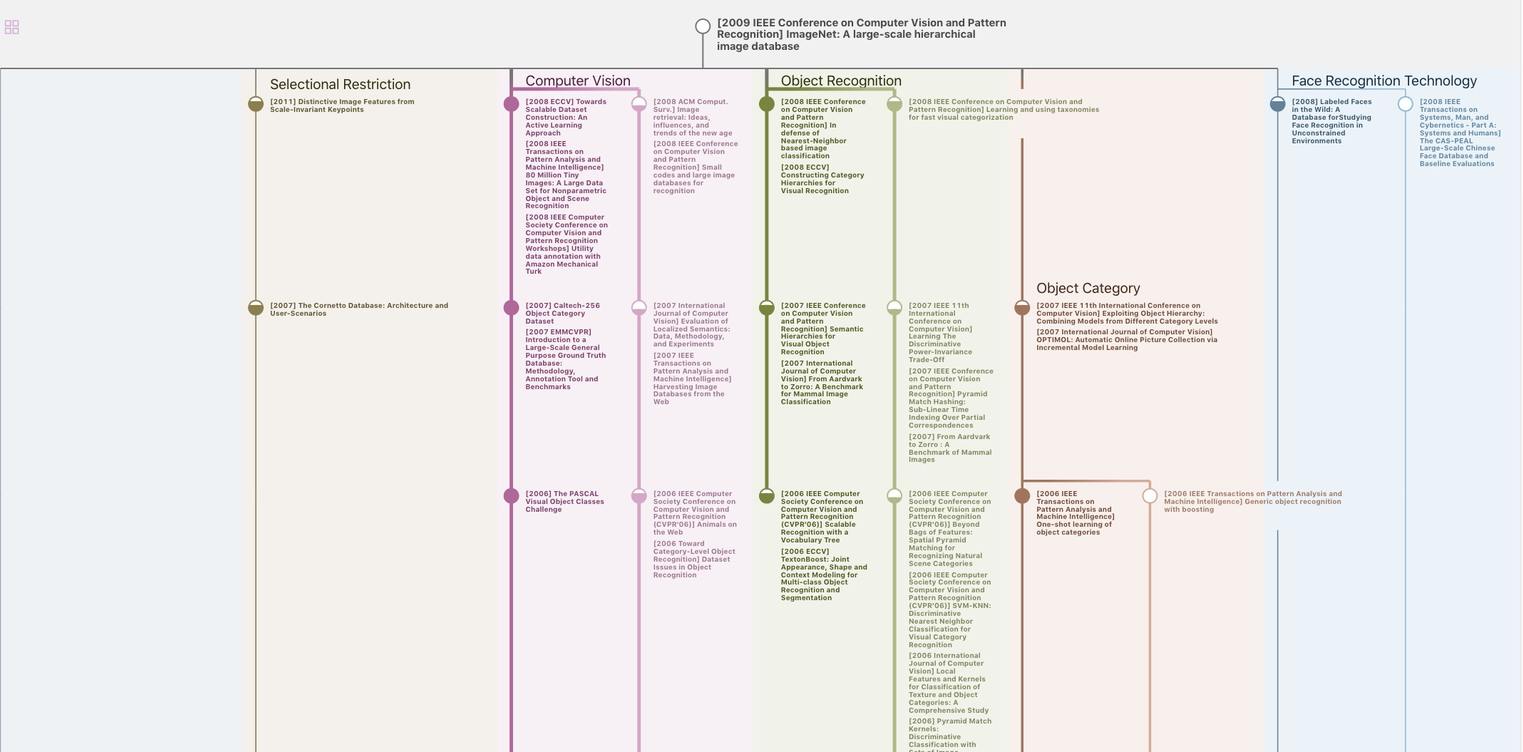
生成溯源树,研究论文发展脉络
Chat Paper
正在生成论文摘要