Infusing structural assumptions into dimension reduction for single-cell RNA sequencing data to identify small gene sets
biorxiv(2024)
摘要
Dimension reduction greatly facilitates the exploration of cellular heterogeneity in single-cell RNA sequencing data. While most of such approaches are data-driven, it can be useful to incorporate biologically plausible assumptions about the underlying structure or the experimental design. We propose the boosting autoencoder (BAE) approach, which combines the advantages of unsupervised deep learning for dimension reduction and boosting for formalizing assumptions. Specifically, our approach selects small sets of genes that explain latent dimensions. As illustrative applications, we explore the diversity of neural cell identities and temporal patterns of embryonic development.
### Competing Interest Statement
The authors have declared no competing interest.
更多查看译文
AI 理解论文
溯源树
样例
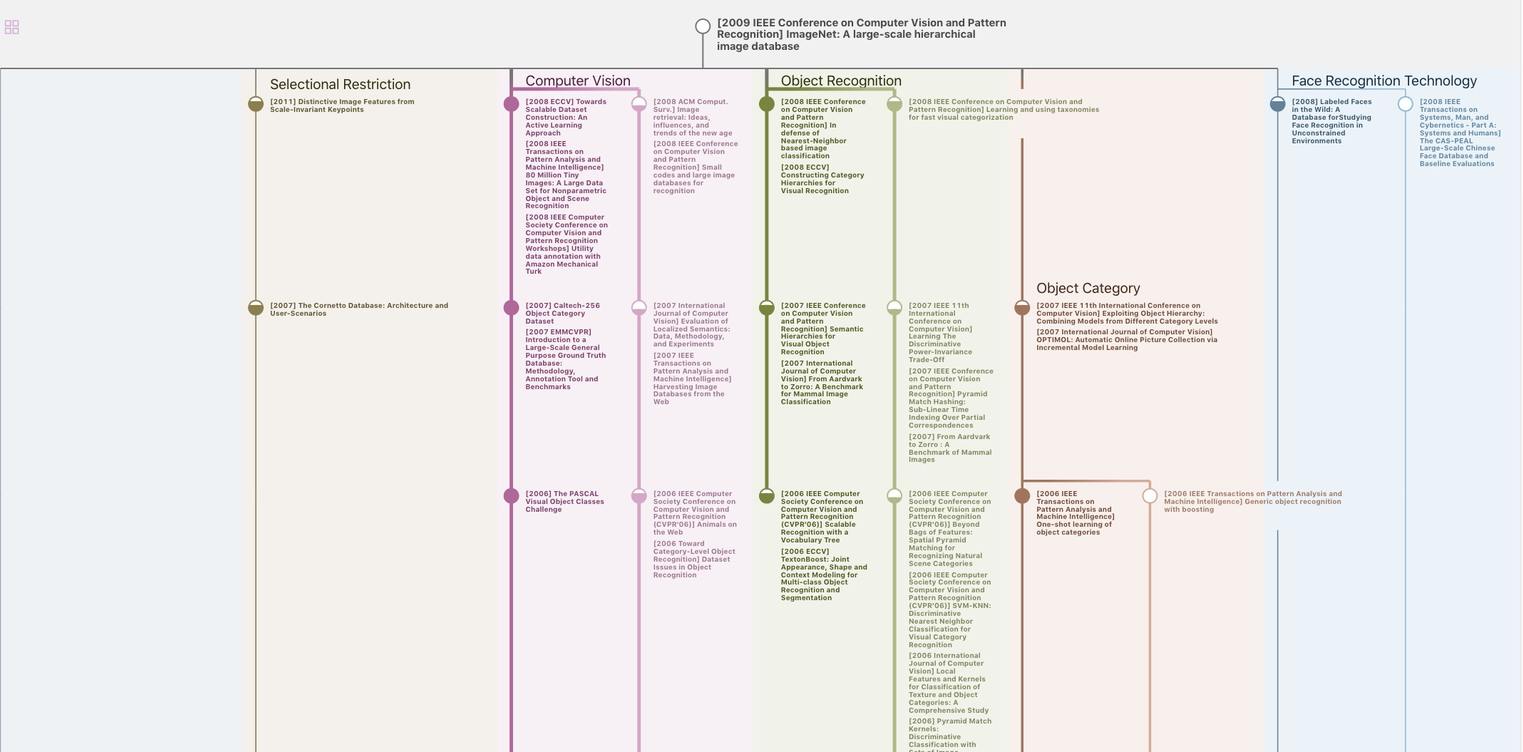
生成溯源树,研究论文发展脉络
Chat Paper
正在生成论文摘要