Goal-Conditioned Offline Reinforcement Learning via Metric Learning
CoRR(2024)
摘要
In this work, we address the problem of learning optimal behavior from
sub-optimal datasets in the context of goal-conditioned offline reinforcement
learning. To do so, we propose a novel way of approximating the optimal value
function for goal-conditioned offline RL problems under sparse rewards,
symmetric and deterministic actions. We study a property for representations to
recover optimality and propose a new optimization objective that leads to such
property. We use the learned value function to guide the learning of a policy
in an actor-critic fashion, a method we name MetricRL. Experimentally, we show
how our method consistently outperforms other offline RL baselines in learning
from sub-optimal offline datasets. Moreover, we show the effectiveness of our
method in dealing with high-dimensional observations and in multi-goal tasks.
更多查看译文
AI 理解论文
溯源树
样例
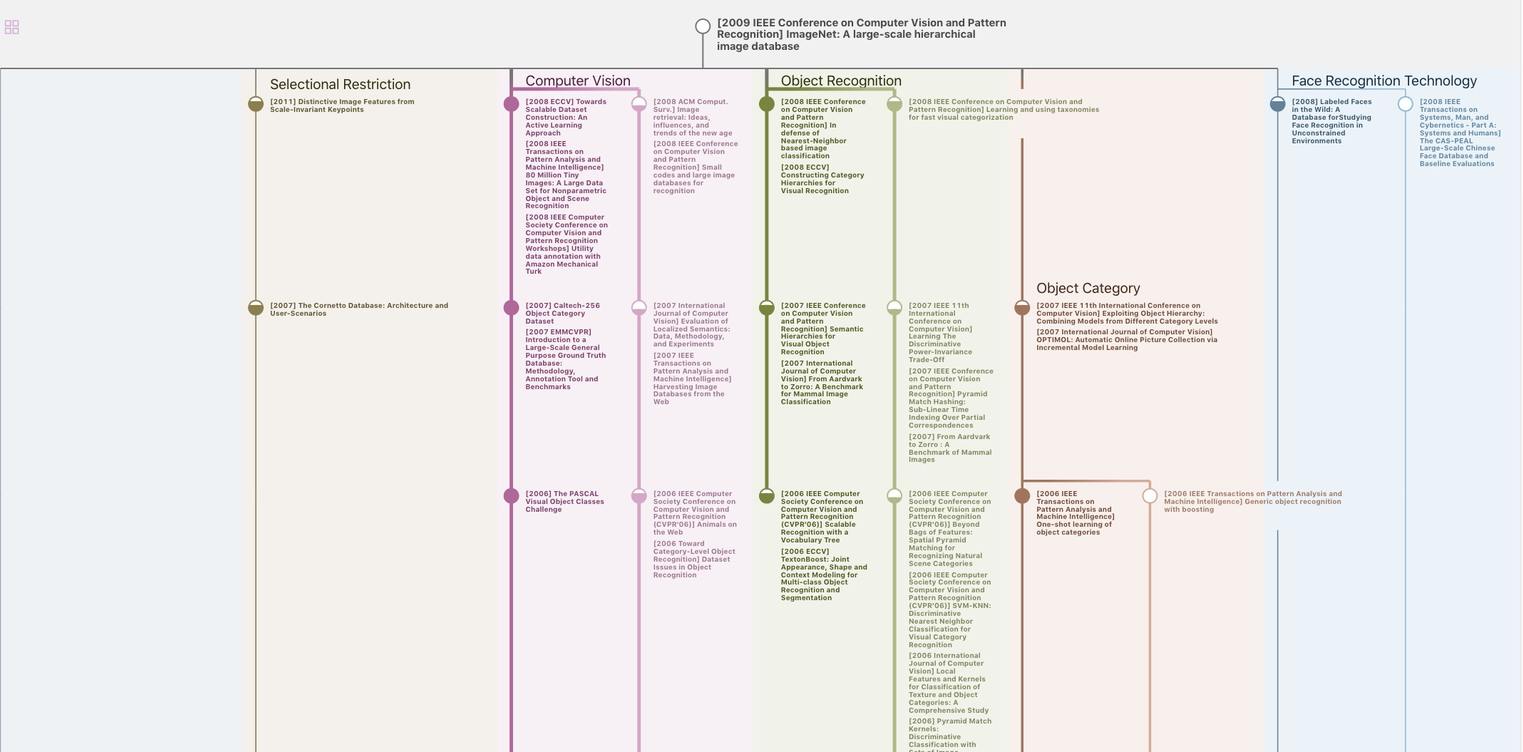
生成溯源树,研究论文发展脉络
Chat Paper
正在生成论文摘要