Autonomous Vehicle Patrolling Through Deep Reinforcement Learning: Learning to Communicate and Cooperate
CoRR(2024)
摘要
Autonomous vehicles are suited for continuous area patrolling problems.
Finding an optimal patrolling strategy can be challenging due to unknown
environmental factors, such as wind or landscape; or autonomous vehicles'
constraints, such as limited battery life or hardware failures. Importantly,
patrolling large areas often requires multiple agents to collectively
coordinate their actions. However, an optimal coordination strategy is often
non-trivial to be manually defined due to the complex nature of patrolling
environments. In this paper, we consider a patrolling problem with
environmental factors, agent limitations, and three typical cooperation
problems – collision avoidance, congestion avoidance, and patrolling target
negotiation. We propose a multi-agent reinforcement learning solution based on
a reinforced inter-agent learning (RIAL) method. With this approach, agents are
trained to develop their own communication protocol to cooperate during
patrolling where faults can and do occur. The solution is validated through
simulation experiments and is compared with several state-of-the-art patrolling
solutions from different perspectives, including the overall patrol
performance, the collision avoidance performance, the efficiency of battery
recharging strategies, and the overall fault tolerance.
更多查看译文
AI 理解论文
溯源树
样例
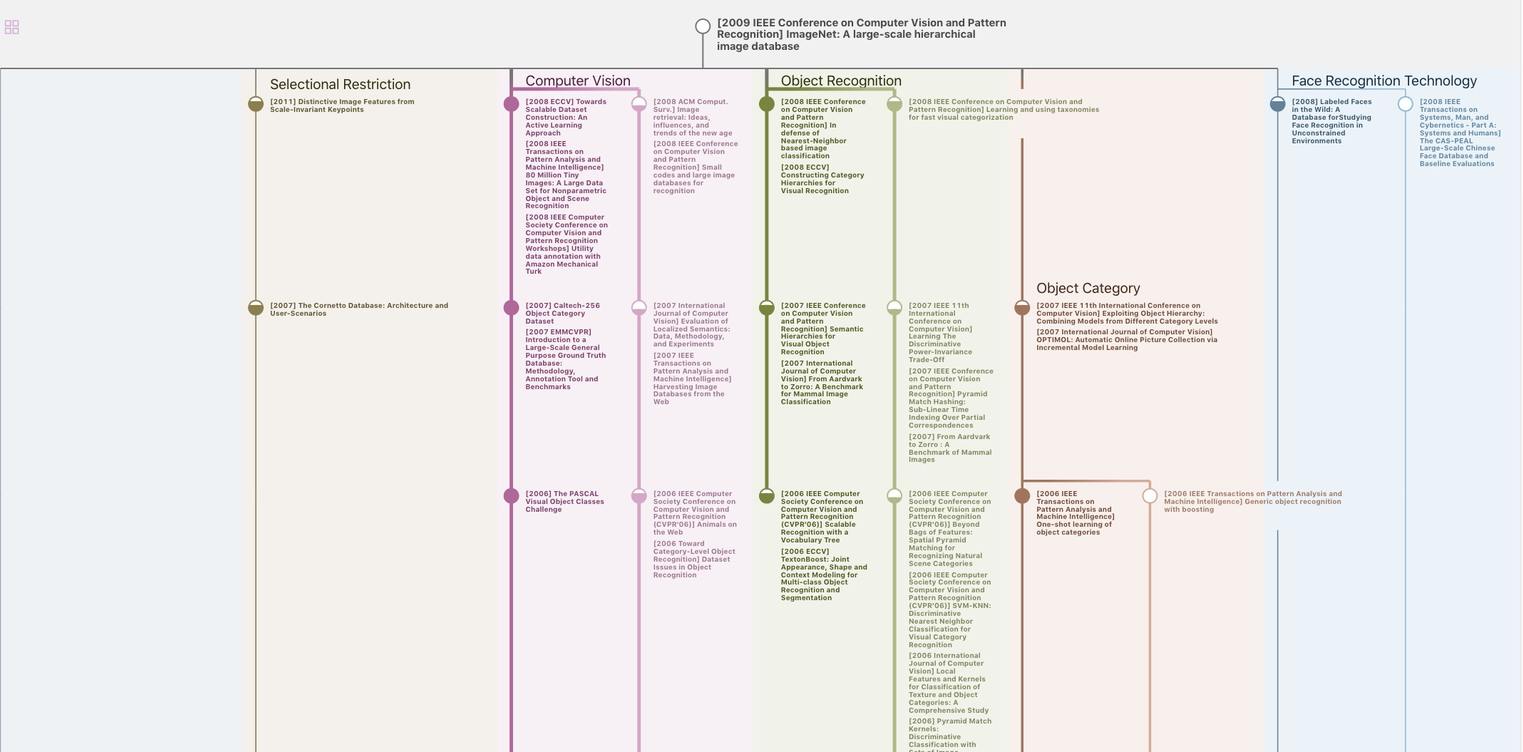
生成溯源树,研究论文发展脉络
Chat Paper
正在生成论文摘要