Reinforcement Learning-based Performance-aware Energy Management in 5G Base Stations.
International Conference on Communication Systems and Networks(2024)
摘要
Although 5G wireless networks are significantly energy efficient compared to their 4G predecessors in terms of data bits per kilowatts, the higher density of base stations needed to support the expected exponential growth in wireless traffic nullifies any accrued energy efficiency benefits and instead consumes significantly more energy than 4G. A variety of mechanisms exist to handle this challenge including but not limited to putting inactive radios into sleep mode and reducing power to unused antennas, among others. Yet, a systematic and dynamic approach that can leverage these mechanisms in a way that continues to deliver the desired quality of service to users and accounts for workload fluctuations while saving energy costs is still lacking. This poster presents our ideas based on reinforcement learning to address these challenges. These ideas are based on our prior success in applying such an approach in managing energy consumption of server resources in high performance computing clusters. In this poster we outline our training method used to train the algorithm that is independent of the user application and present work-in-progress that evaluates the proposed technique in the Colosseum 5G network emulator, which is a 5G testbed hosted at Northeastern University, USA.
更多查看译文
关键词
Base-station,HPC,RL,power optimization,RAPL,energy efficiency
AI 理解论文
溯源树
样例
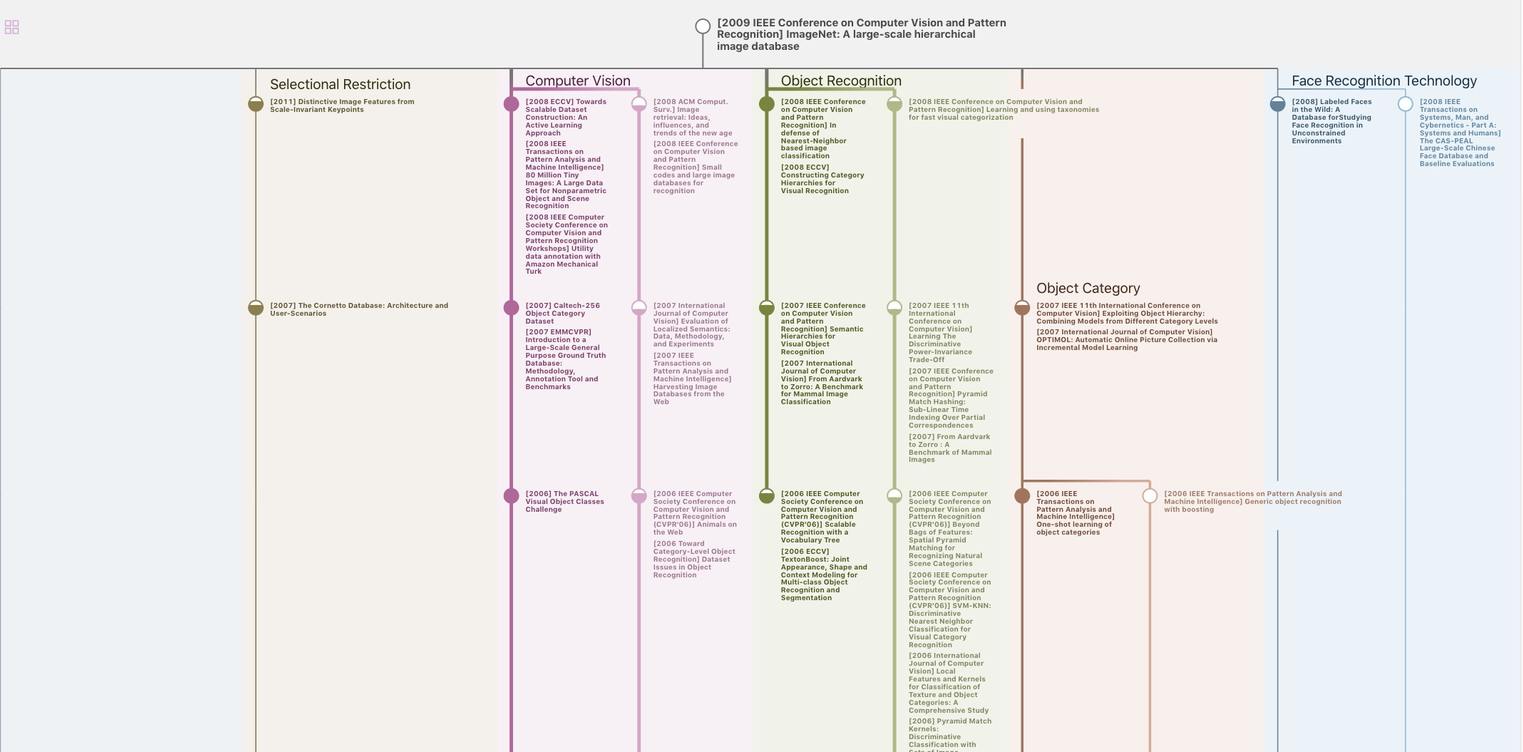
生成溯源树,研究论文发展脉络
Chat Paper
正在生成论文摘要