Integrative Classification Using Structural Equation Modeling of Homeostasis
Statistics in Biosciences(2024)
摘要
We consider binary classification in the high-dimensional setting, where the number of features is huge, and the number of observations is limited. We focus on the setting where features in one group have certain correlation structures that are not present in the other group. This is particularly relevant in early detection of diseases where subjects develop from a normal or homeostatic state to a diseased condition. Linear discriminant analysis (with a link function) and classification based on regularized regression or machine learning have been used as methods for this problem and related variable selection. However, most methods do not account for the correlation structures of variables within groups. While the diseased group may demonstrate abundant diversity and no clear structure, achieving higher accuracy in classification requires considering the correlation structures in the control group with homeostasis. In this paper, we develop a structural equation modeling approach to characterize the correlation structures of homeostasis, and the parameters are estimated using only the data from one group. The structural equation models are not applicable to the data from the other group, and the classification specificity and sensitivity are determined by choosing the confidence intervals of the estimated parameters. We use a real multi-platform genomics dataset to illustrate the methods, and we demonstrate that our approach performs well compared to statistical learning methods such as regularized logistic regression models.
更多查看译文
关键词
Cancer diagnosis,Classification,Covariance structure,Integrative analysis,Genomic data,Structural equation model
AI 理解论文
溯源树
样例
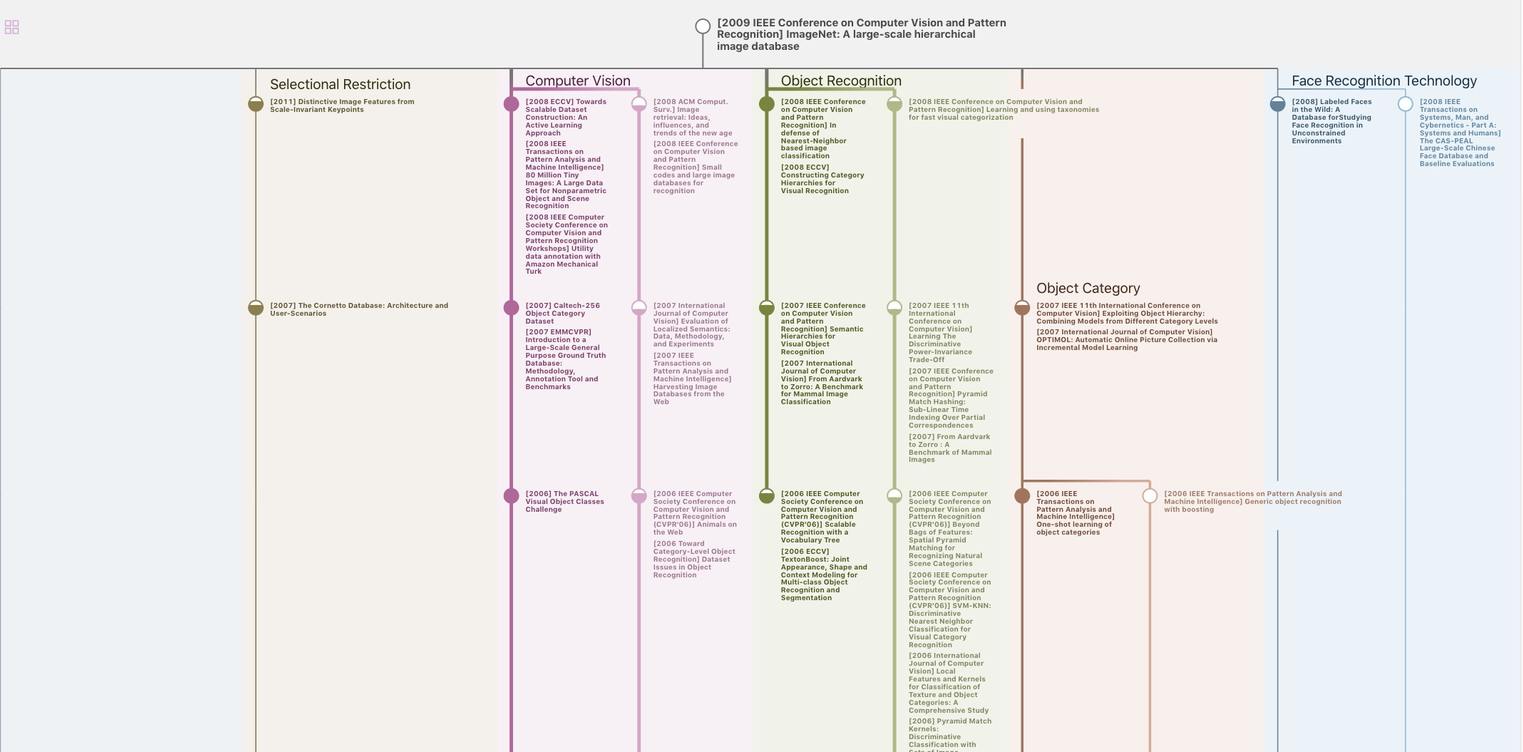
生成溯源树,研究论文发展脉络
Chat Paper
正在生成论文摘要