Exploring conditional pixel-independent generation in GAN inversion for image processing
Multimedia Tools and Applications(2024)
摘要
Image processing holds an indispensable role in various facets of our daily lives, professional undertakings, and educational pursuits, encompassing a gamut of tasks including image reconstruction, inpainting, super-resolution, colorization, and editing. In recent years, the advent of advanced models rooted in Generative Adversarial Networks (GANs) has showcased remarkable capabilities in the domain of image synthesis, catapulting the direct application of these cutting-edge models to image processing to the forefront of contemporary research. Within this context, GAN inversion, an emerging paradigm, assumes a pivotal role in the landscape of image processing tasks. This paper delves into the realm of image inversion based on the latent space of GAN models. In response to the inherent limitations of current GAN inversion methods, we introduce three innovations. Firstly, we depart from the conventional use of convolutional networks for generator implementation in existing GAN inversion techniques. Our approach employs generators entirely composed of fully connected layers, marking a significant departure from spatial convolutions and information propagation across pixels. Secondly, we leverage the distinct characteristic of generators engaged in conditional independent pixel synthesis. This feature is enhanced by fusing feature maps spanning contiguous strata of a feature pyramid network during the feature extraction process. Lastly, our framework offers a high degree of versatility, extending its applicability beyond image reconstruction to domains like image inpainting, super-resolution, and image colorization. Empirical results, based on the CelebFaces Attribute-HQ (CelebA-HQ) dataset, unequivocally demonstrate that GAN inversion, built on the principle of conditional independent pixel synthesis, yields superior reconstruction outcomes. Furthermore, it proves amenable to a plethora of tasks, including image inpainting, super-resolution, and image colorization. These advances open new vistas in image processing.
更多查看译文
关键词
Generative Adversarial Networks (GANs),Image generation,Latent space,Deep learning,Semantic similarity
AI 理解论文
溯源树
样例
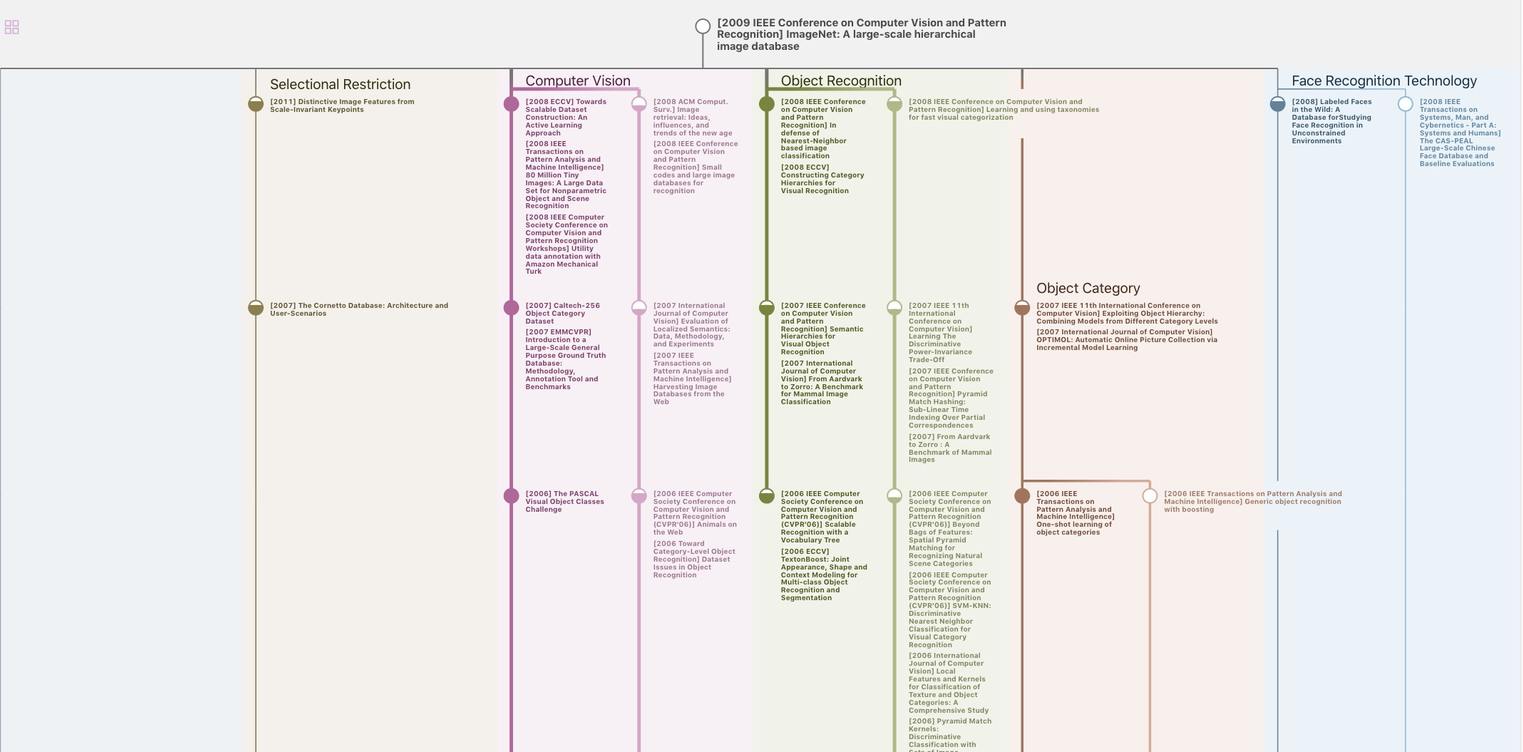
生成溯源树,研究论文发展脉络
Chat Paper
正在生成论文摘要