Improving the Quality of Unstained Transmission Electron Microscope Images Using Deep Learning
2023 Cross Strait Radio Science and Wireless Technology Conference (CSRSWTC)(2023)
摘要
In the field of biomedical science, Transmission Electron Microscopy (TEM) is one of the essential tools for studying cellular structure and function. Traditional TEM image acquisition requires a heavy metal staining process, and unstained TEM images often suffer from issues such as low contrast, noise, and blurriness, which limit the observation of image resolution and details. We propose a workflow that utilizes deep learning to enhance the quality of unstained transmission electron microscope images, aiming to achieve high-quality images without the need for traditional staining processes. The proposed deep learning-based method provides an effective and efficient solution for enhancing the quality of unstained TEM images. This has significant practical implications for further investigations into cellular structures and biological functions, as well as improving the accuracy and reliability of TEM image analysis.
更多查看译文
关键词
deep learning,transmission electron microscopy,medical image processing,convolutional neural network,generative adversarial network
AI 理解论文
溯源树
样例
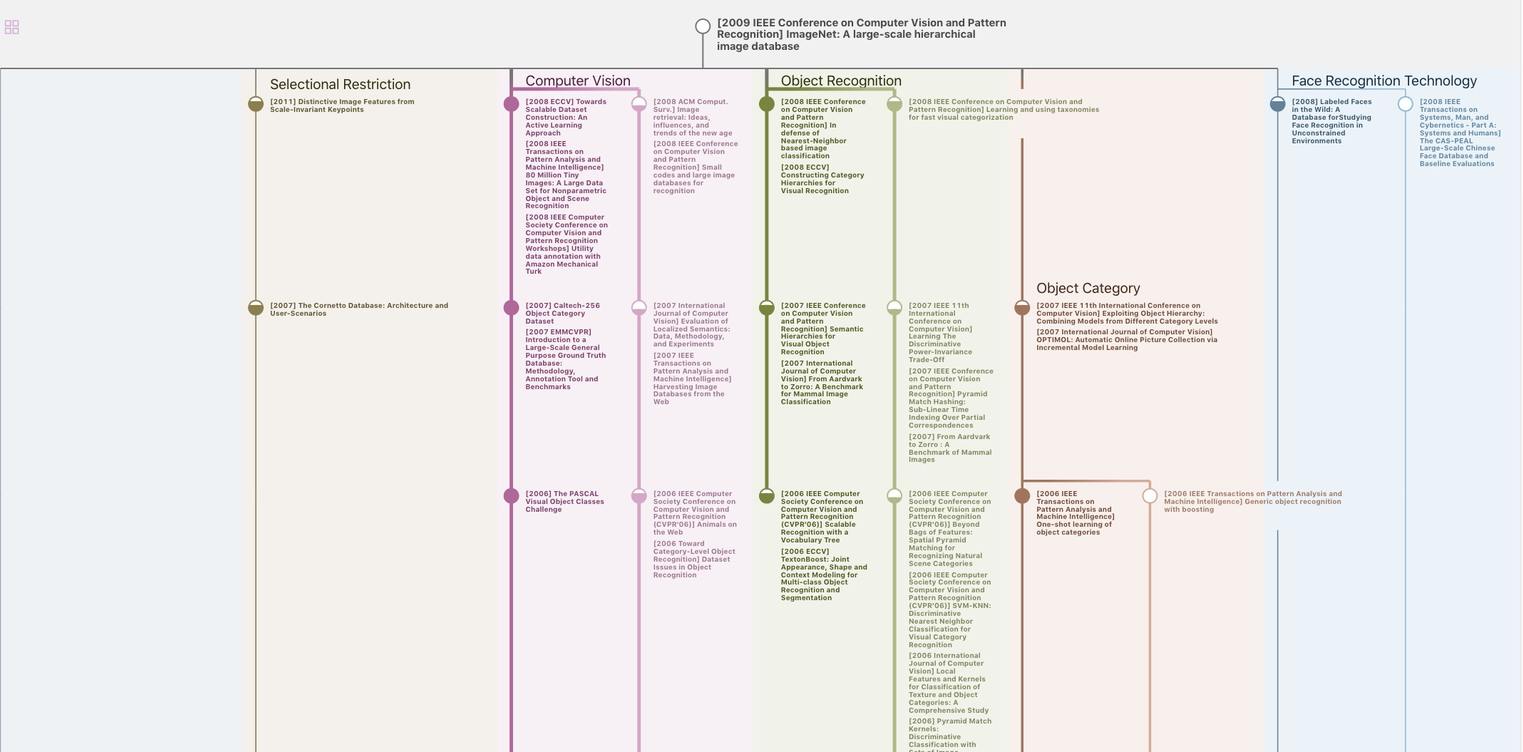
生成溯源树,研究论文发展脉络
Chat Paper
正在生成论文摘要