Learning Robust Skills for Tightly Coordinated Arms in Contact-Rich Tasks
IEEE ROBOTICS AND AUTOMATION LETTERS(2024)
摘要
Multi-arm coordination has been receiving increasing attention in industry and our daily life. However, empowering arms to skillfully manipulate a common object within contact-rich tasks remains a daunting challenge. In this letter, we introduce a novel learning-based framework for arms to manipulate the object skillfully while maintaining tight coordination, which not only avoids dynamic modeling for keeping tight coordination but also eliminates the necessity to pre-program task-specific skills for handling complex contacts. To the best of our knowledge, our study is the first to concentrate on tightly coordinated arms in contact-rich tasks. Specifically, we improve and utilize value-decomposed multi-agent policy gradient methods to simultaneously learn tight coordination skills and task-specific skills. Contrasting with previous work on multi-arm skill learning, our approach efficiently learns skills without integrating human skills. Furthermore, we evaluate our approach through two challenging dual-arm assembly tasks and the performance exhibits promising robustness and generalizability.
更多查看译文
关键词
Task analysis,Force,Reinforcement learning,Impedance,Fans,End effectors,Computational modeling,Cooperating Robots,Reinforcement Learning,Dual Arm Manipulation,Assembly
AI 理解论文
溯源树
样例
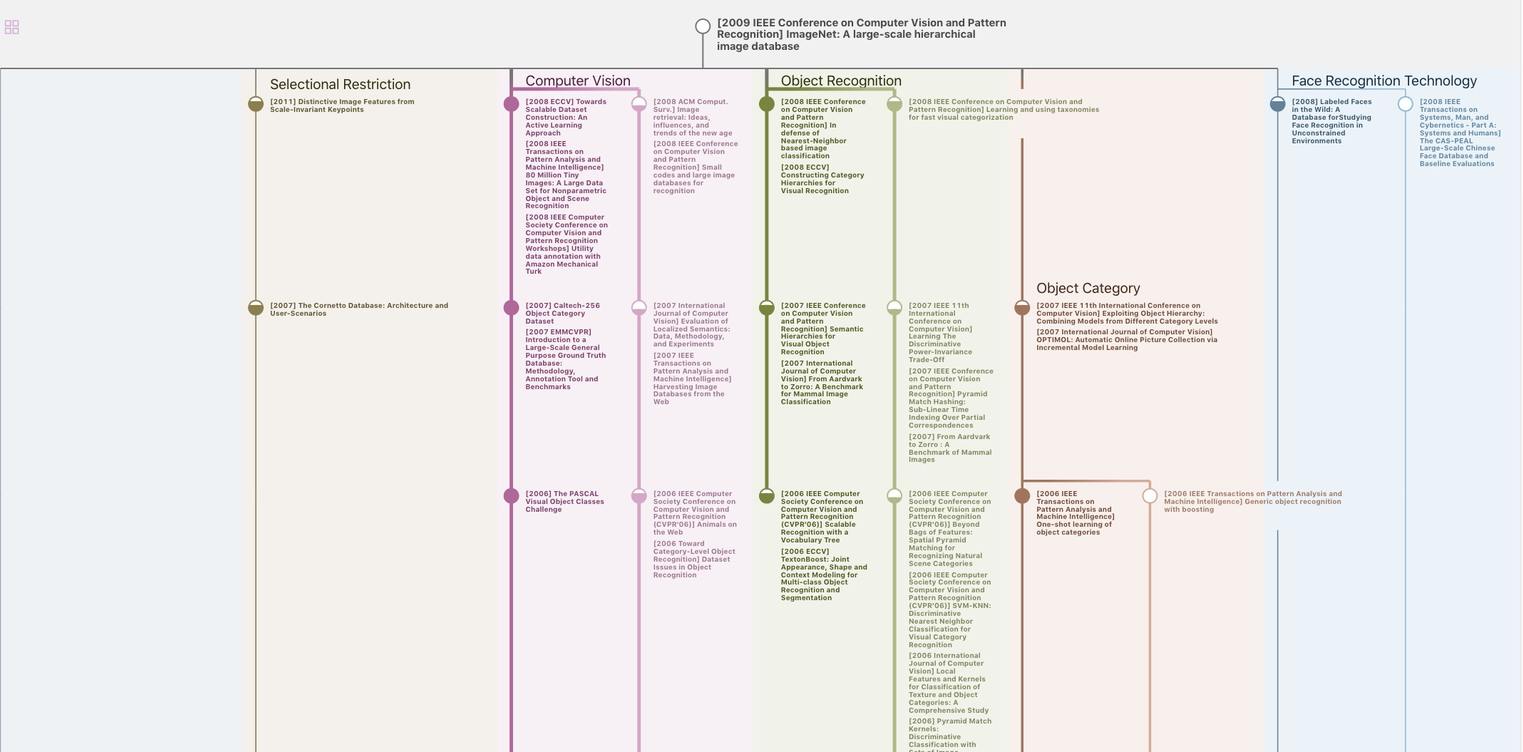
生成溯源树,研究论文发展脉络
Chat Paper
正在生成论文摘要