RUBICON: a framework for designing efficient deep learning-based genomic basecallers
Genome Biology(2024)
摘要
Nanopore sequencing generates noisy electrical signals that need to be converted into a standard string of DNA nucleotide bases using a computational step called basecalling. The performance of basecalling has critical implications for all later steps in genome analysis. Therefore, there is a need to reduce the computation and memory cost of basecalling while maintaining accuracy. We present RUBICON, a framework to develop efficient hardware-optimized basecallers. We demonstrate the effectiveness of RUBICON by developing RUBICALL, the first hardware-optimized mixed-precision basecaller that performs efficient basecalling, outperforming the state-of-the-art basecallers. We believe RUBICON offers a promising path to develop future hardware-optimized basecallers.
更多查看译文
关键词
Genomics sequencing,Basecalling,Hardware acceleration,Machine learning,Deep neural network
AI 理解论文
溯源树
样例
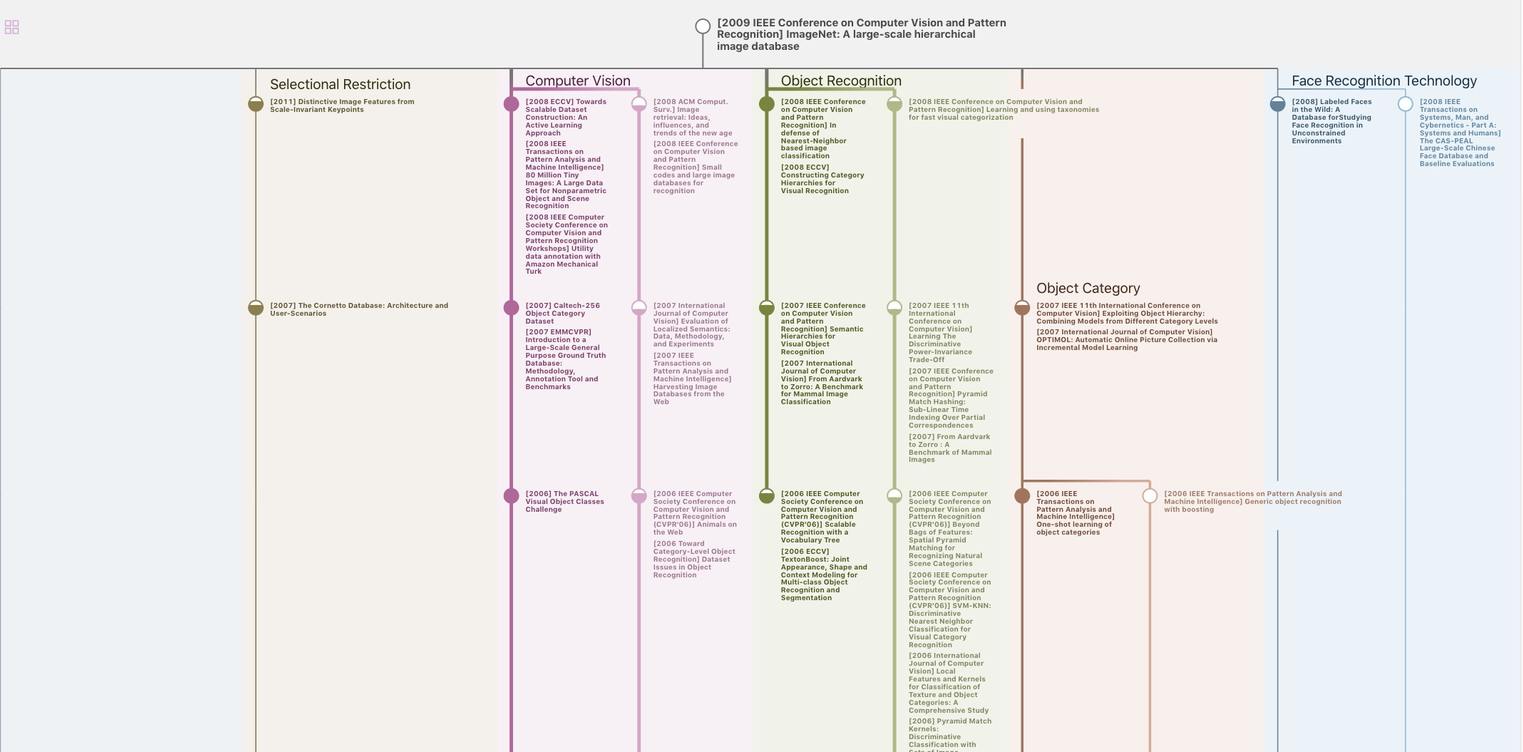
生成溯源树,研究论文发展脉络
Chat Paper
正在生成论文摘要