Tuning Machine Learning to Address Process Mining Requirements
IEEE ACCESS(2024)
摘要
Machine learning models are routinely integrated into process mining pipelines to carry out tasks like data transformation, noise reduction, anomaly detection, classification, and prediction. Often, the design of such models is based on some ad-hoc assumptions about the corresponding data distributions, which are not necessarily in accordance with the non-parametric distributions typically observed with process data. Moreover, mainstream machine-learning approaches tend to ignore the challenges posed by concurrency in operational processes. Data encoding is a key element to smooth the mismatch between these assumptions but its potential is poorly exploited. In this paper, we argue that a deeper understanding of the challenges associated with training machine learning models on process data is essential for establishing a robust integration of process mining and machine learning. Our analysis aims to lay the groundwork for a methodology that aligns machine learning with process mining requirements. We encourage further research in this direction to advance the field and effectively address these critical issues.
更多查看译文
关键词
Process mining,machine learning,non-parametric distribution,concurrency,non-stationary,zero-shot learning,encoding,training
AI 理解论文
溯源树
样例
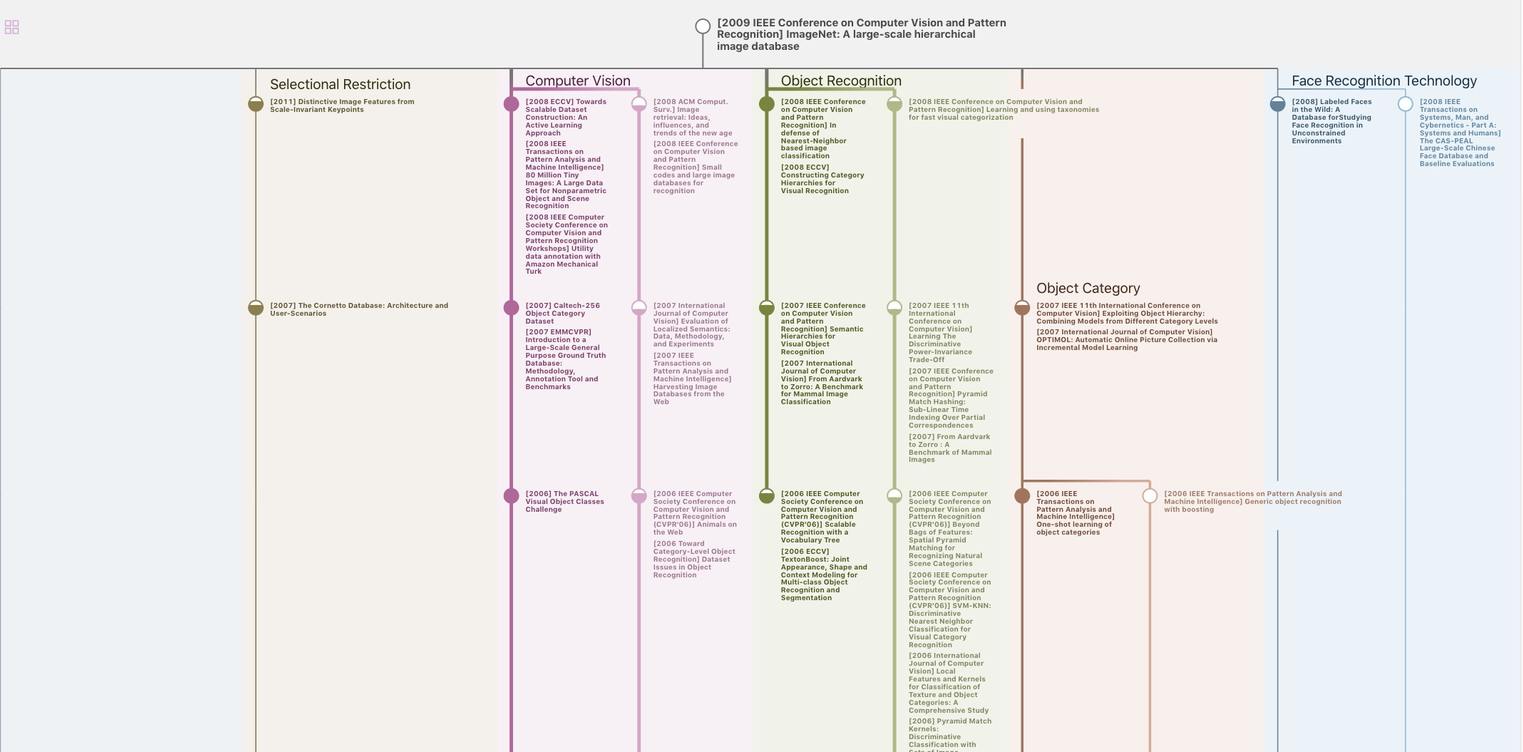
生成溯源树,研究论文发展脉络
Chat Paper
正在生成论文摘要