An Efficient Support Vector Machine Algorithm Based Network Outlier Detection System
IEEE ACCESS(2024)
摘要
With the increase of cyber-attacks and security threats in the recent decade, it is necessary to safeguard sensitive data and provide robust protection to information systems and computer networks. In this paper, an anomaly-based network outlier detection system (NODS) is proposed and optimized to check and classify the incoming network traffic stream's behaviours that affect the computer networks. The proposed NODS has high classification efficiency. Network connection events classified as outliers are reported to the network admin to drop and block its packets. The NSL-KDD and CICIDS2017 intrusion datasets were employed to build the proposed system and test its detection capabilities. Sequential scenarios were implemented to optimize the system's effectiveness. Network features were normalized by min-max and Z-Score approaches, while the relevant features were selected individually by the principal component analysis (PCA) and correlated features selection (CFS) techniques. Support vector machine (SVM) and Gaussian Naive Bayes (GNB) algorithms are used to build the detection model, while the Genetic algorithm (GA) was employed to tune their control parameters. The obtained evaluation results proved that the proposed SVM based NODS is characterized by low false alarms and detection time as well as high classification accuracy. Furthermore, a comparative analysis was conducted with other existing techniques, and the results obtained demonstrate the effectiveness of the proposed SVM-IDS
更多查看译文
关键词
Outlier detection,NSL-KDD,CICIDS2017,features normalization,features selection,support vector machine,Gaussian Naive Bayes,genetic algorithm,RBF,tunning parameters
AI 理解论文
溯源树
样例
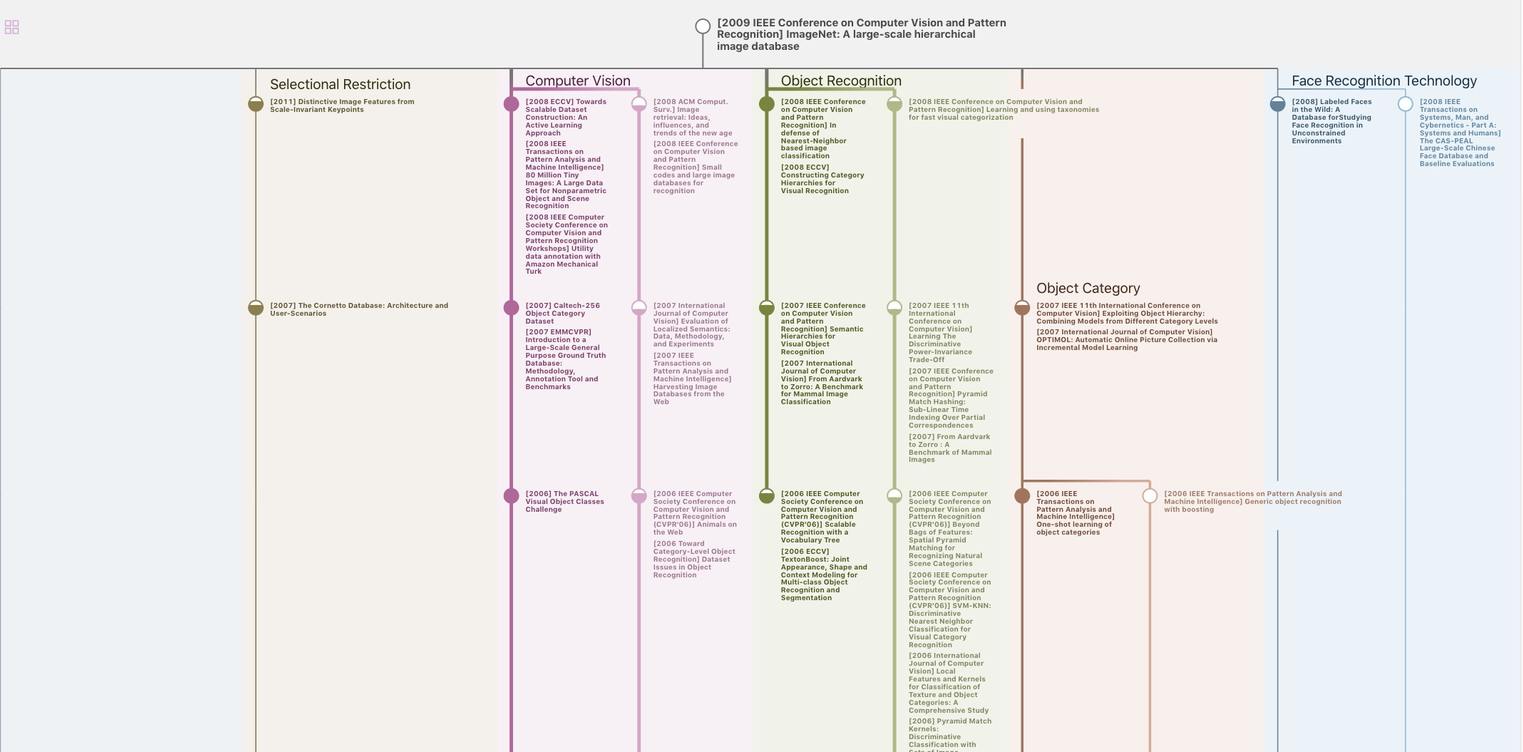
生成溯源树,研究论文发展脉络
Chat Paper
正在生成论文摘要