Double Deep Q Networks Reinforcement Learning based Controller for Grid-Connected 7-Level PUC Inverter
2024 4th International Conference on Smart Grid and Renewable Energy (SGRE)(2024)
摘要
The Packed-U-Cell (PUC) topology has been widely employed as a single DC-source multilevel inverter (MLI) in various applications, with different control techniques. In grid-connected applications, the two main control objectives are the minimization of the total harmonic distortion (THD%) of the generated current and the regulation of the flying capacitor voltage at its reference value. This paper proposes a reinforcement learning-based control (RLC) using the off-policy value function-based double deep Q networks (DDQN) reinforcement learning algorithm for a grid-tied single-phase 7-level PUC inverter. The agent consists of two DQNs that estimate the value function and determine the control action at each time step. The reinforcement learning-based control using DDQN (RLC-DDQN) is trained by interacting with the PUC7 system on three different pairs of current and voltage references. In the testing phase, the trained agent was tested on different operating conditions to validate its performance. The presented results show that the current THD has been kept below 3% for different reference signals from 4A to 11A, whereas the maximum deviation of the capacitor voltage from its volts reference value is 0.5V. The trained RLC-DDQN algorithm shows a high dynamic response under disturbed operating conditions (variations in the reference signals including the grid current, the DC-source voltage, and the voltage-current phase-shift). Moreover, the robustness of the trained agent when applied on systems with different component design (capacitance and inductance variations) is verified.
更多查看译文
关键词
Double Deep Q Networks,Grid Connection,Multilevel Inverter,Packed-U-Cell,Reinforcement Learning
AI 理解论文
溯源树
样例
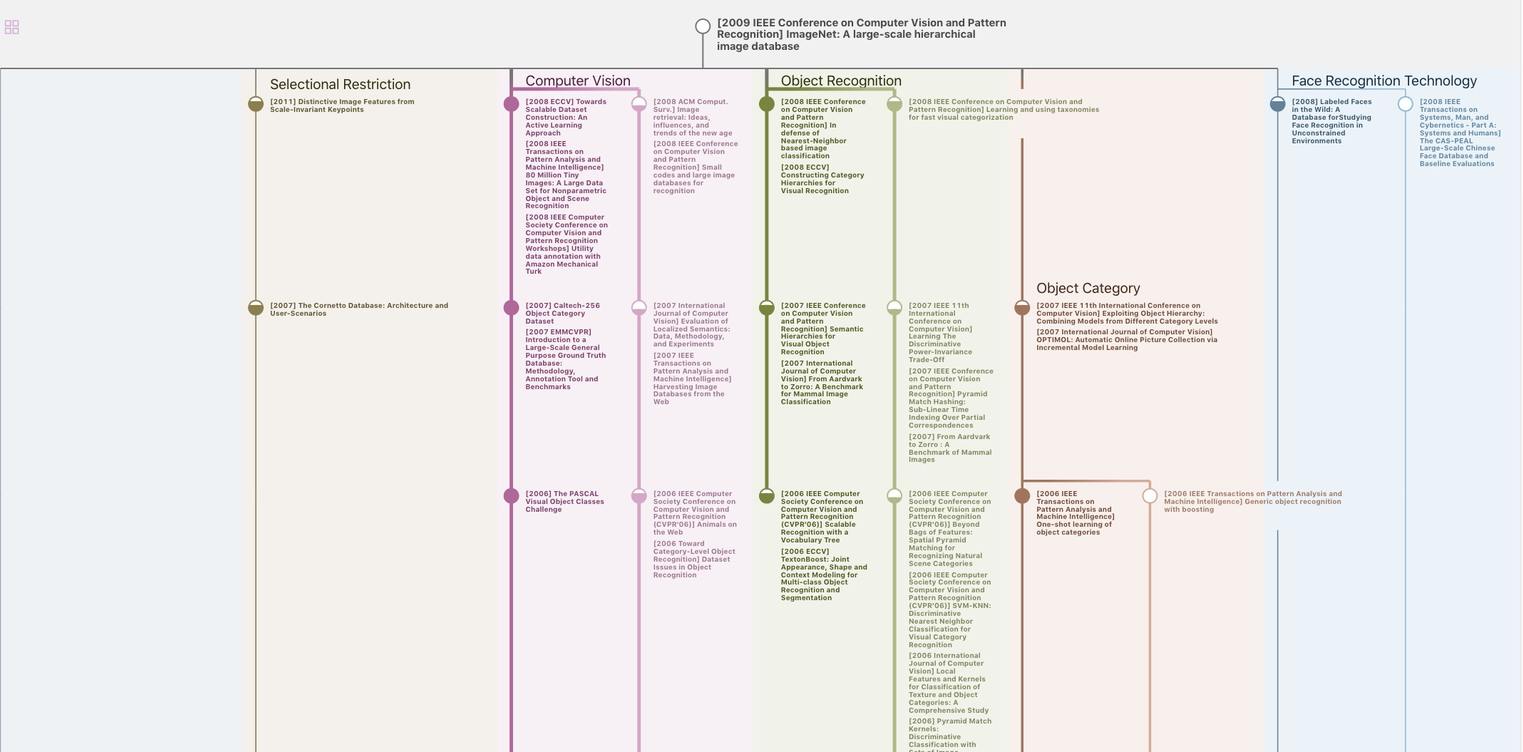
生成溯源树,研究论文发展脉络
Chat Paper
正在生成论文摘要