Scalable Light-weight Anomaly Detection for Data of Individual Smart Meters
2024 4th International Conference on Smart Grid and Renewable Energy (SGRE)(2024)
摘要
With the rise in attacks on power grid networks, traditional cybersecurity mechanisms alone prove inadequate, necessitating the integration of novel security tools. This paper proposes a scalable anomaly detection framework for individual smart meters using deep neural networks (DNNs). The proposed framework leverages clustering to improve performance over different load profiles. It utilizes lightweight DNNs for load forecasting and anomaly detection. In this work, the system’s scalability is prioritized, aligning with the requirements of large-scale smart grid implementations. The proposed framework is scalable and easily deployable, addressing factors influencing the success of anomaly detection. The anomaly detection model achieved an accuracy of over 84% when tested on synthesized False Data Injection (FDI) attacks.
更多查看译文
关键词
Smart meters,Anomaly detection,Deep learning
AI 理解论文
溯源树
样例
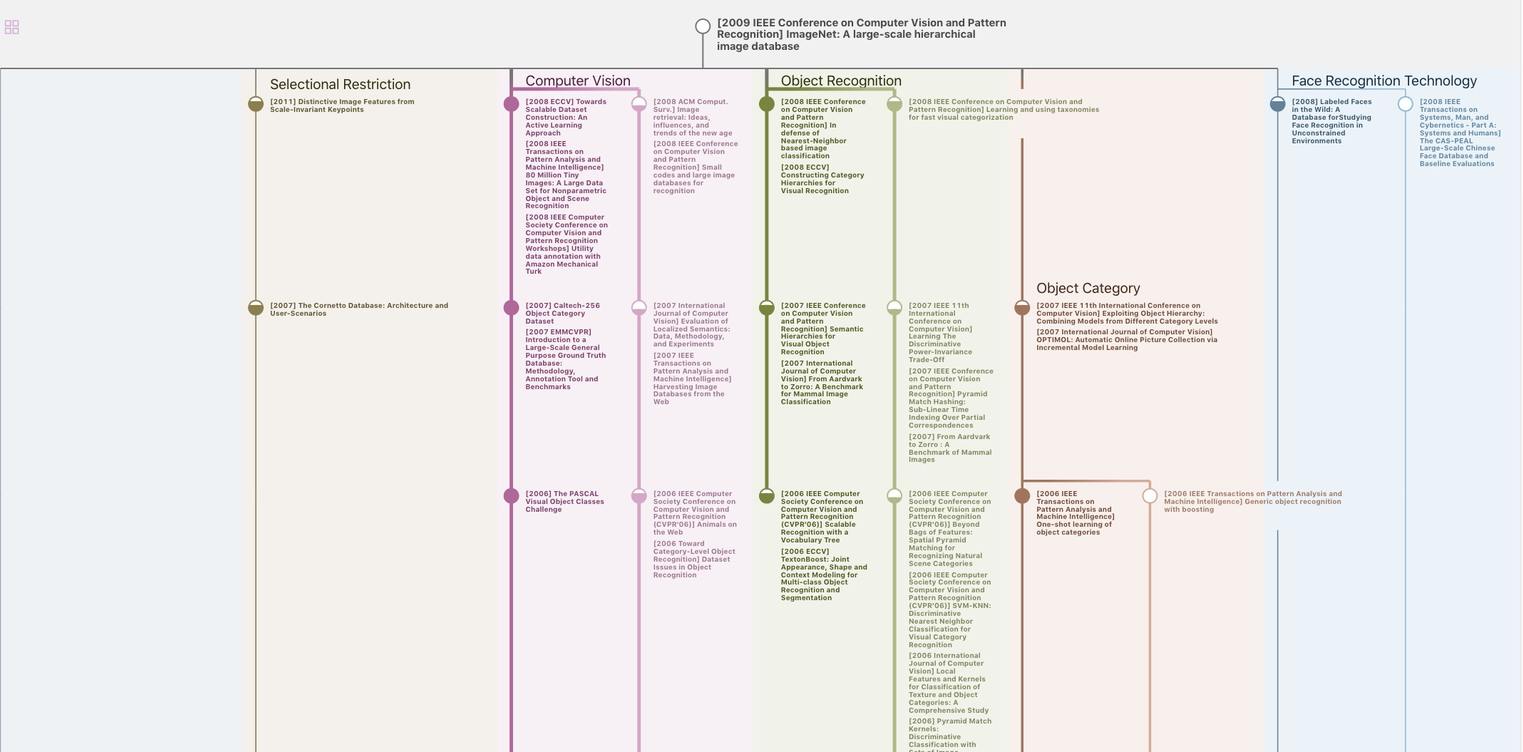
生成溯源树,研究论文发展脉络
Chat Paper
正在生成论文摘要