PMDAGS: Predicting Mirna-disease Associations with Graph Nonlinear Diffusion Convolution Network and Similarities.
IEEE/ACM transactions on computational biology and bioinformatics(2024)
摘要
Many studies have proven that microRNAs (miRNAs) can participate in a wide range of biological processes and can be considered as potential noninvasive biomarkers for disease diagnosis and prognosis. However, it is well-established that identifying potential miRNA-disease associations through wet-lab experimental methods is expensive and time-consuming. Therefore, many computational methods have been developed to reduce the cost of identifying miRNA-disease associations, ultimately enhancing the efficiency of disease diagnosis and treatment. In this study, we also introduced a new computational method called PMDAGS, which predicts miRNA-disease associations by utilizing graph nonlinear diffusion convolution network and similarities. PMDAGS first calculates miRNA functional similarity and disease functional similarity based on miRNA-target interactions and disease-gene associations, respectively. Additionally, we compute Gaussian Interaction Profile (GIP) kernel similarities of miRNAs and diseases based on the known miRNA-disease associations. The final miRNA similarity is obtained by fusing the miRNA GIP kernel similarity to fill in the zero values of miRNA functional similarity. Similarly, based on disease functional similarity and GIP kernel similarity, we obtain the final disease similarity using the same principle. Next, we construct the initial feature of each miRNA (disease) by concatenating its final similarity vector with its known association vector. Based on the known miRNA-disease association network and the initial feature vector of each node, we further apply nonlinear diffusion graph convolution network model to extract the feature embedding vectors. Finally, we concatenate the feature embedding vectors of miRNA and disease and input them into a multi-layer perceptron to generate the low-dimensional embedding of miRNA-disease pairs, which is then fed into a sigmoid classifier to identify potential miRNA-disease associations. To evaluate the prediction performance of our method and compare it with other computational methods, we conduct 5-fold cross validation (5CV), 10-fold cross validation (10CV), and global leave-one-out cross validation (GLOOCV) on HMDD v2.0 and HMDD v3.2. The evaluation metrics includes the area under the receiver operating characteristic curve (AUC), area under the precision-recall curve (AUPR), accuracy, precision, recall, and F1-score. PMDAGS achieves AUCs of 0.9222, 0.9228, and 0.9221 under 5CV, 10CV and GLOOCV on HMDD v2.0, respectively. In addition, PMDAGS also achieves AUC values of 0.9366, 0.9377, and 0.9376 under 5CV, 10CV and GLOOCV on HMDD v3.2, respectively. According to the experimental results, we can conclude that PMDAGS outperforms other compared methods. Additionally, the case studies also demonstrate that PMDAGS can effectively predict miRNA-disease associations and provide assistance in disease diagnosis and treatment.
更多查看译文
关键词
miRNA,Disease,miRNA-disease associations,Similarity,Graph nonlinear diffusion convolution network
AI 理解论文
溯源树
样例
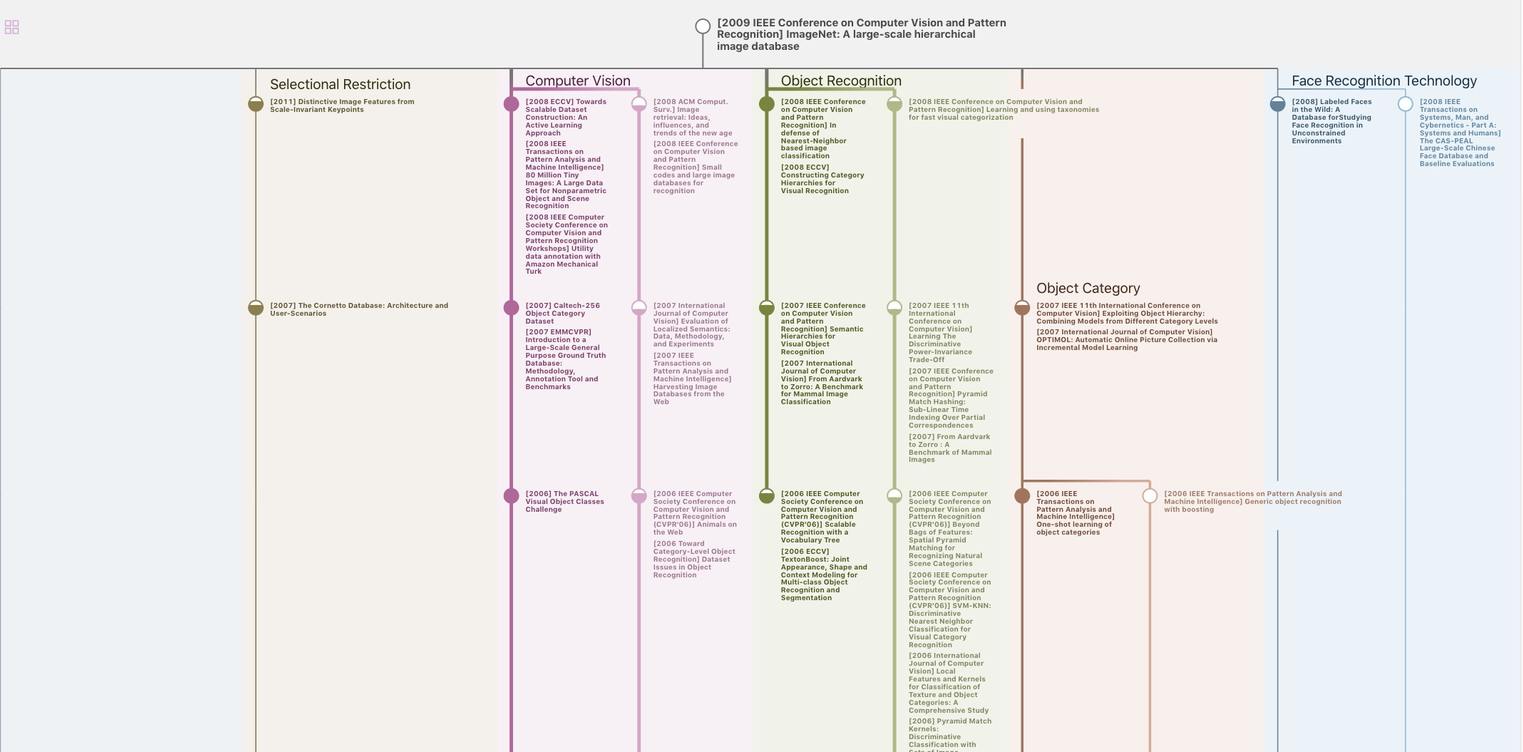
生成溯源树,研究论文发展脉络
Chat Paper
正在生成论文摘要