Data-Driven Multi-Objective Optimization: Analysis of Current Methods and Techniques
2023 IEEE CHILEAN Conference on Electrical, Electronics Engineering, Information and Communication Technologies (CHILECON)(2023)
摘要
Multi-objective optimization holds practical relevance, as the majority of real-world problems involve multiple conflicting objectives. This study aims to analyze data-driven multi-objective optimization methods, focusing on key aspects such as the employed algorithms, incorporation of machine learning techniques as surrogate models, number of evaluated objectives, consideration of model interpretability, and metrics used to evaluate the performance of the algorithms. This research distinguishes itself by providing a review and analysis of dataguided multi-objective optimization algorithms over the last six years. The results offer valuable guidance to the scientific community seeking to address complex problems using datadriven multi-objective optimization algorithms.
更多查看译文
关键词
data-driven algorithms,interpretability,machine learning,multi-objective optimization,surrogate model
AI 理解论文
溯源树
样例
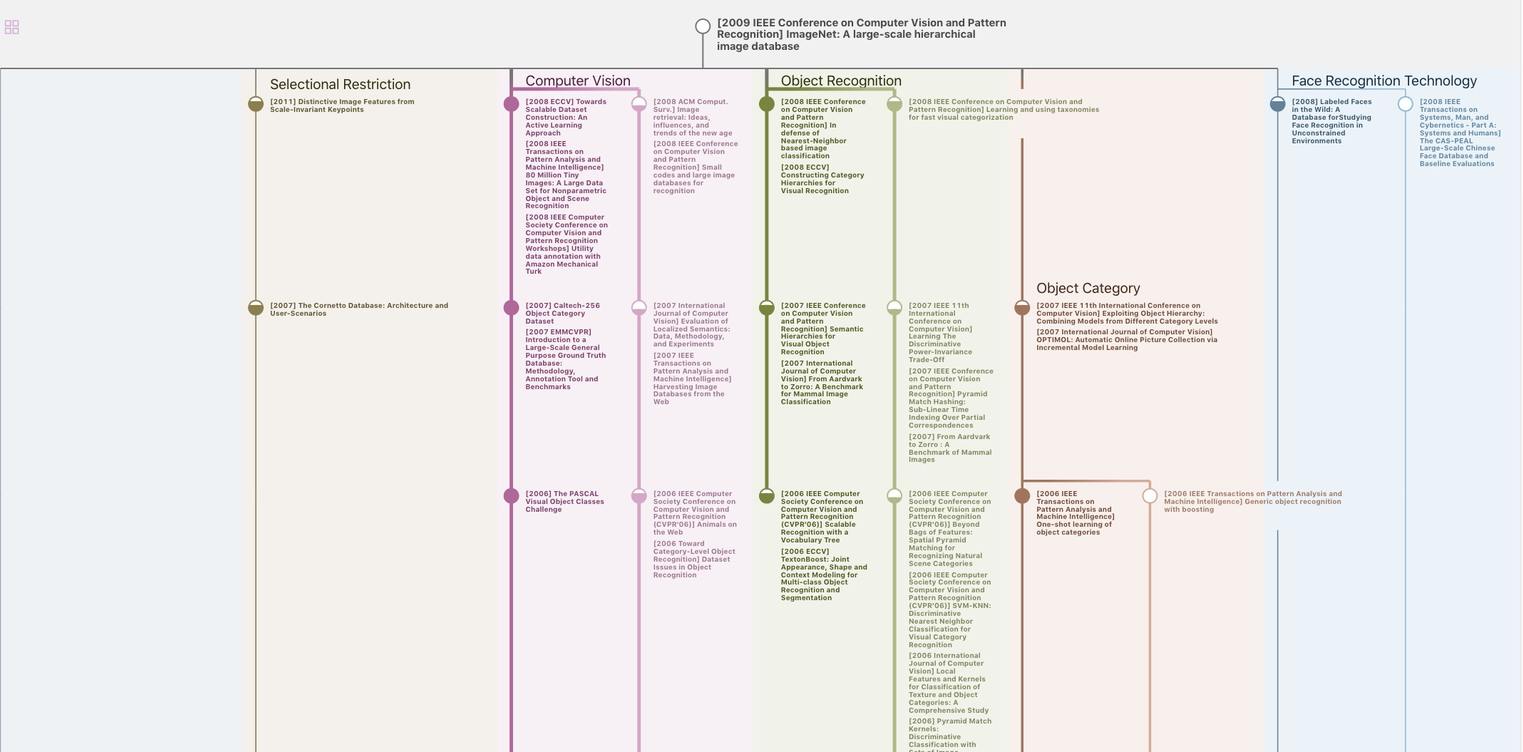
生成溯源树,研究论文发展脉络
Chat Paper
正在生成论文摘要