Open-Science Guidance for Qualitative Research: An Empirically Validated Approach for De-Identifying Sensitive Narrative Data
ADVANCES IN METHODS AND PRACTICES IN PSYCHOLOGICAL SCIENCE(2023)
摘要
The open-science movement seeks to make research more transparent and accessible. To that end, researchers are increasingly expected to share de-identified data with other scholars for review, reanalysis, and reuse. In psychology, open-science practices have been explored primarily within the context of quantitative data, but demands to share qualitative data are becoming more prevalent. Narrative data are far more challenging to de-identify fully, and because qualitative methods are often used in studies with marginalized, minoritized, and/or traumatized populations, data sharing may pose substantial risks for participants if their information can be later reidentified. To date, there has been little guidance in the literature on how to de-identify qualitative data. To address this gap, we developed a methodological framework for remediating sensitive narrative data. This multiphase process is modeled on common qualitative-coding strategies. The first phase includes consultations with diverse stakeholders and sources to understand reidentifiability risks and data-sharing concerns. The second phase outlines an iterative process for recognizing potentially identifiable information and constructing individualized remediation strategies through group review and consensus. The third phase includes multiple strategies for assessing the validity of the de-identification analyses (i.e., whether the remediated transcripts adequately protect participants' privacy). We applied this framework to a set of 32 qualitative interviews with sexual-assault survivors. We provide case examples of how blurring and redaction techniques can be used to protect names, dates, locations, trauma histories, help-seeking experiences, and other information about dyadic interactions.
更多查看译文
关键词
open science,data sharing,data archiving,qualitative interview,de-identification,dyadic data
AI 理解论文
溯源树
样例
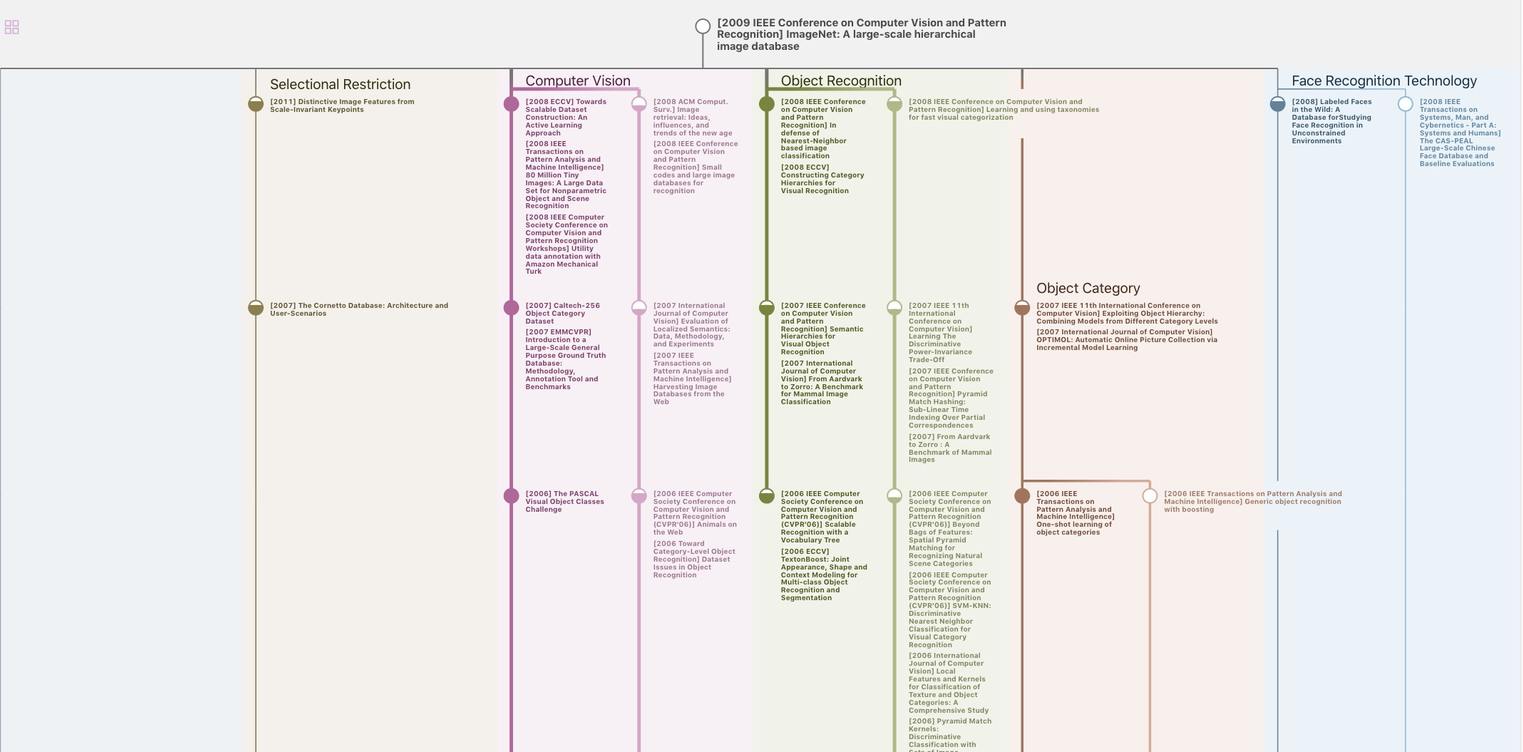
生成溯源树,研究论文发展脉络
Chat Paper
正在生成论文摘要