Harnessing heterogeneity in behavioural research using computational social science
BEHAVIOURAL PUBLIC POLICY(2023)
摘要
Similarly to other domains of the social sciences, behavioural science has grappled with a crisis concerning the effect sizes of research findings. Different solutions have been provided to answer this challenge. This paper will discuss analytical strategies developed in the context of computational social science, namely causal tree and forest, that will benefit behavioural scientists in harnessing heterogeneity of treatment effects in RCTs. As a mixture of theoretical and data-driven approaches, these techniques are well suited to exploit the rich information provided by large studies conducted using RCTs. We discuss the characteristics of these methods and their methodological rationale and provide simulations to illustrate their use. We simulate two scenarios of RCTs-generated data and explore the heterogeneity of treatment effects using causal tree and causal forest methods. Furthermore, we outlined a potential theoretical use of these techniques to enrich behavioural science ecological validity by introducing the notion of behavioural niche.
更多查看译文
关键词
treatment effect,heterogeneity,computational social science,causal forest,behavioural niches
AI 理解论文
溯源树
样例
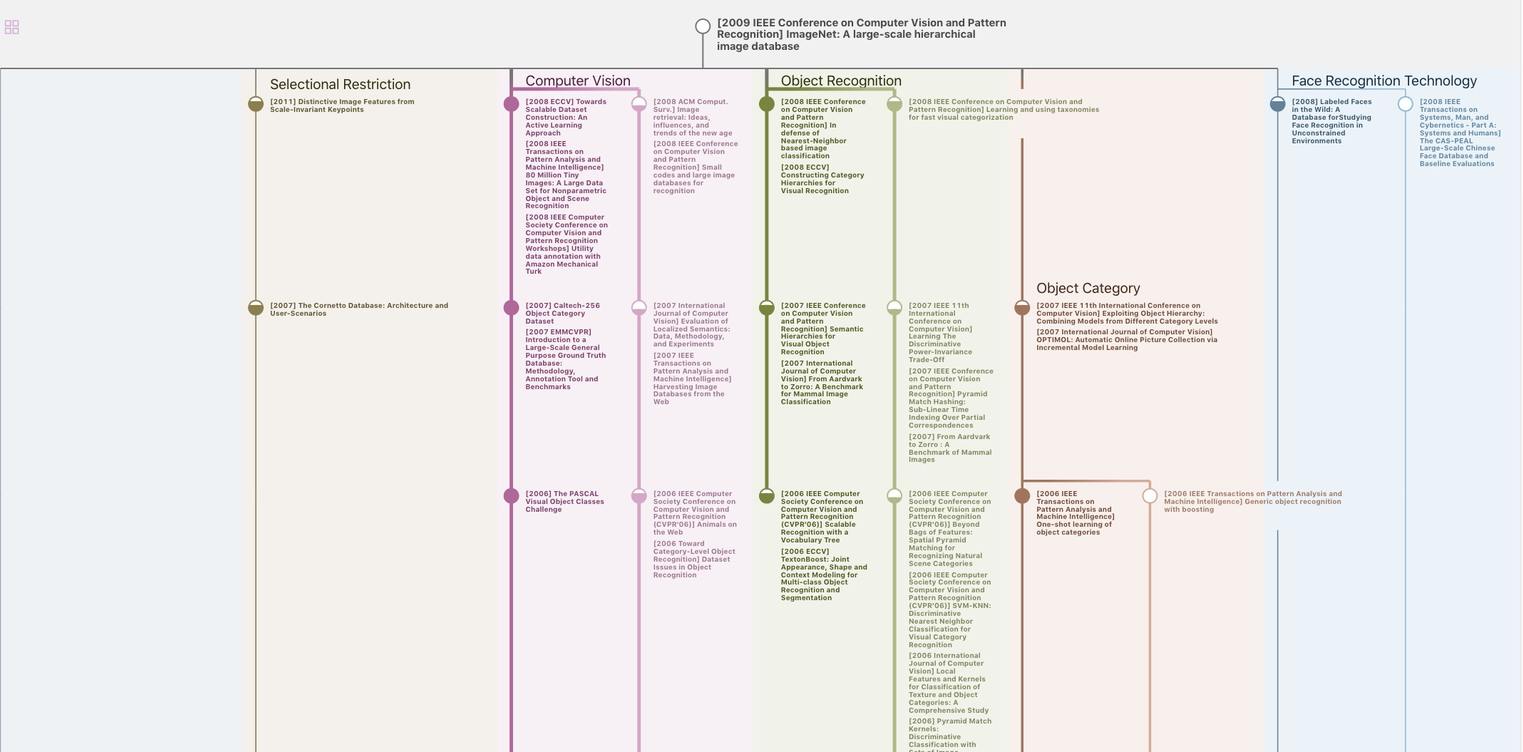
生成溯源树,研究论文发展脉络
Chat Paper
正在生成论文摘要