Scalable high-dimensional Bayesian varying coefficient models with unknown within-subject covariance
JOURNAL OF MACHINE LEARNING RESEARCH(2023)
摘要
Nonparametric varying coefficient (NVC) models are useful for modeling time-varying effects on responses that are measured repeatedly for the same subjects. When the number of covariates is moderate or large, it is desirable to perform variable selection from the varying coefficient functions. However, existing methods for variable selection in NVC models either fail to account for within-subject correlations or require the practitioner to specify a parametric form for the correlation structure. In this paper, we introduce the nonparametric varying coefficient spike-and-slab lasso (NVC-SSL) for Bayesian high-dimensional NVC models. Through the introduction of functional random effects, our method allows for flexible modeling of within-subject correlations without needing to specify a parametric covariance function. We further propose several scalable optimization and Markov chain Monte Carlo (MCMC) algorithms. For variable selection, we propose an Expectation Conditional Maximization (ECM) algorithm to rapidly obtain maximum a posteriori (MAP) estimates. Our ECM algorithm scales linearly in the total number of observations N and the number of covariates p. For uncertainty quantification, we introduce an approximate MCMC algorithm that also scales linearly in both N and p. We demonstrate the scalability, variable selection performance, and inferential capabilities of our method through simulations and a real data application. These algorithms are implemented in the publicly available R package NVCSSL on the Comprehensive R Archive Network.
更多查看译文
关键词
functional random effects,spike-and-slab group lasso,variable selection,varying coefficient model,within-subject covariance
AI 理解论文
溯源树
样例
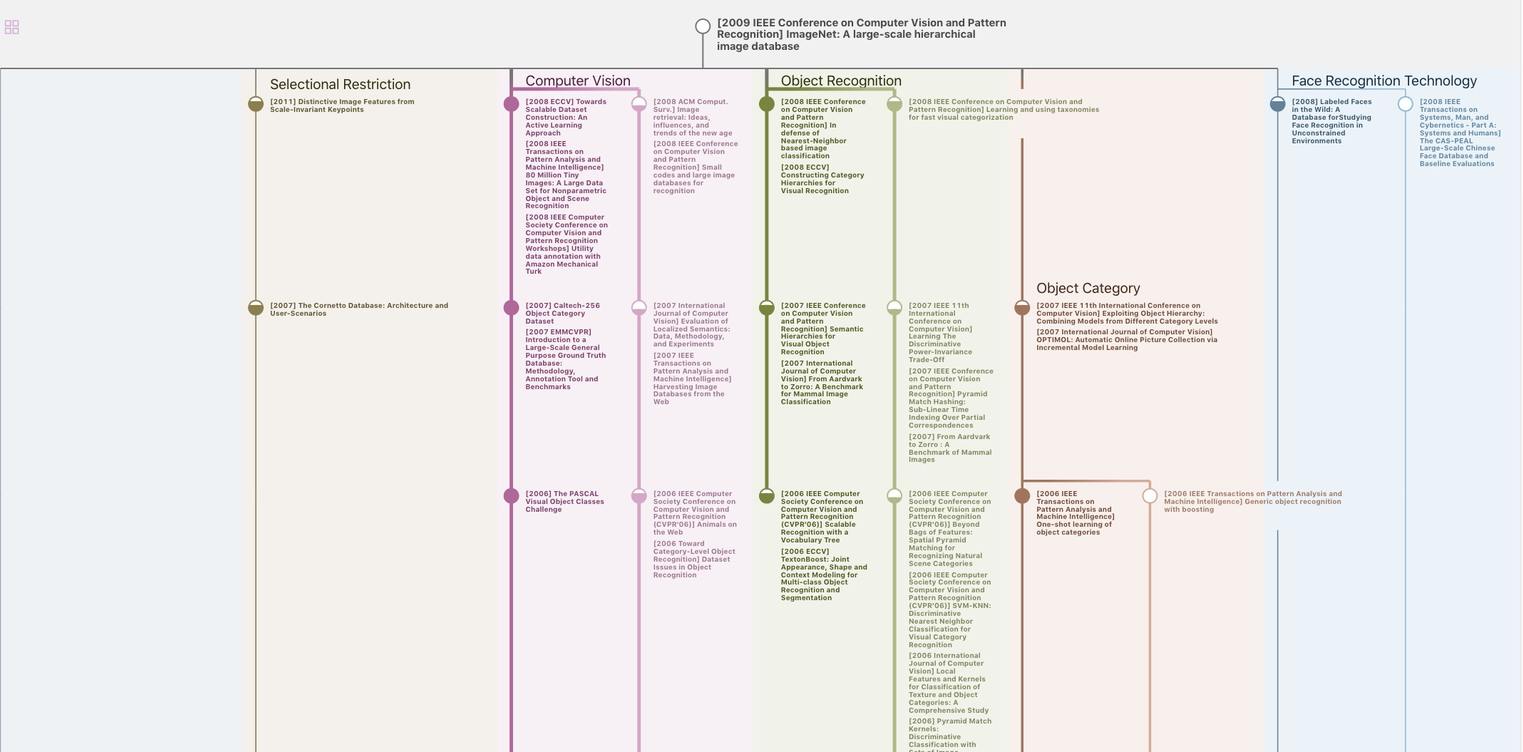
生成溯源树,研究论文发展脉络
Chat Paper
正在生成论文摘要