Attention-Based Deep Learning Framework for Action Recognition in a Dark Environment
HUMAN-CENTRIC COMPUTING AND INFORMATION SCIENCES(2024)
摘要
Human interactions and action recognition (HIAR) are critical tasks in video analytics and understanding. While HIAR has garnered significant attention in the visible spectrum, other modalities, such as infrared (IR), have posed significant challenges in achieving accurate action recognition (AR) due to hazy surface textures and inappropriate features. Current mainstream approaches typically consider either action or contextual information to recognize human action and interactions in the IR spectrum. We propose a convolutional block attention-based action and interaction recognition network (AIR-Net) that reflects both action, contextual features and can be utilized in the dark, as well as visually light environments in a broad range of applications such as surveillance, security, and healthcare. The proposed method is mainly categorized into three steps. Firstly, the video stream is passed through pre-processing, which transforms the IR input data into a sequence of frames. Next, the action features are extracted, aided by a backbone model followed by a convolutional block attention module, while contextual features are extracted using a fine-tuned InceptionV3 network. Finally, a two-stream bidirectional long short-term memory (BiLSTM) network is applied to acquire temporal patterns individually. These sequence patterns are intelligently fused and forwarded to the associated unistream BiLSTM, which learns expedient information for action and interaction recognition. A comprehensive ablation study over two benchmark datasets (InfAR and NTU-RGB+D) demonstrates that our proposed method outperforms state-of-the-art methods. Specifically, the recognition performance is marginally improved on InfAR by 2.5% and achieved 80.94% on NTU-RGB+D.
更多查看译文
关键词
Human Action Recognition,Infrared View Action Recognition,Action Recognition in Low Light,Activity,Recognition,Cognitive Computing in Our Daily Lives,Video Processing,Action Recognition at Night,Human-Machine Interaction
AI 理解论文
溯源树
样例
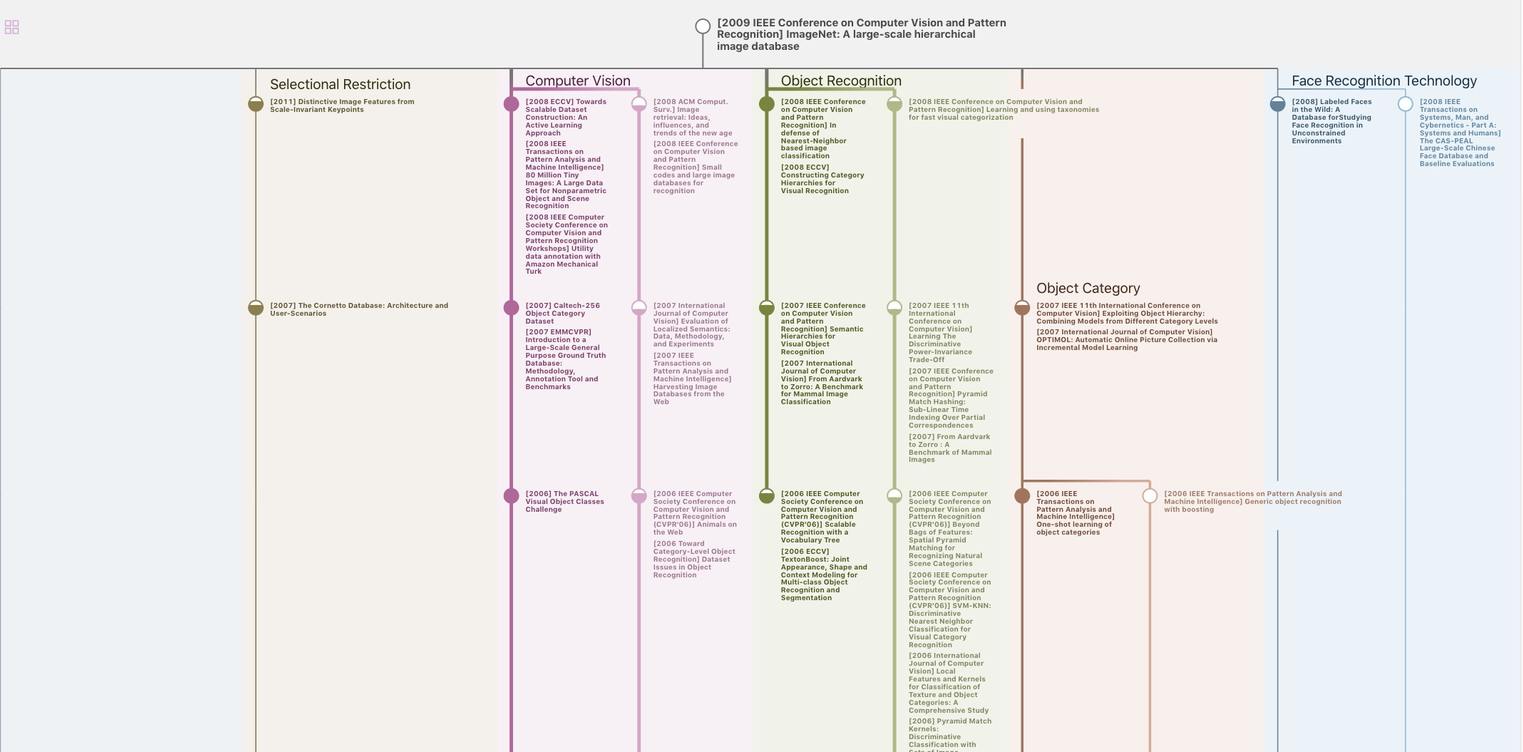
生成溯源树,研究论文发展脉络
Chat Paper
正在生成论文摘要