Prediction models of suicide and non-fatal suicide attempt after discharge from a psychiatric inpatient stay: A machine learning approach on nationwide Danish registers
ACTA PSYCHIATRICA SCANDINAVICA(2023)
摘要
Introduction: To develop machine learning models capable of predicting suicide and non-fatal suicide attempt as separate outcomes in the first 30 days after discharge from a psychiatric inpatient stay.Methods:Prospective cohort study using nationwide Danish registry data. We included individuals who were 18 years or older, and all discharges from psychiatric hospitalizations in Denmark from 1995 to 2018. We trained predictive models using 10-fold cross validation on 80% of the data and did testing on the remaining 20%.Results: The best model for predicting non-fatal suicide attempt was an ensemble of predictions from gradient boosting (XGBoost) and categorical boosting (catBoost). The ROC-AUC for predicting suicide attempt was 0.85 (95% CI: 0.84-0.85). At a risk threshold of 4.36%, positive predictive value (PPV) was 11.0% and sensitivity was 47.2%. The best model for predicting suicide was an ensemble of predictions from random forest, XGBoost and catBoost. For suicide, the ROC-AUC was 0.71 (95% CI: 0.70-0.73). At a risk threshold of 0.15%, PPV was 0.34% and sensitivity was 56.0%. The most contributing predictors differed when predicting suicide and suicide attempt, indicating that separate models are needed. The ensemble model was fair across sex and age, and more so than the penalized logistic regression model.Conclusions: We achieved good performance for predicting suicide attempts and demonstrated a clinical application of ensemble models. Our results indicate a difference in predictive performance for models predicting suicide and suicide attempt, respectively. Thus, we recommend that suicide and suicide attempt are treated as two separate endpoints, in particular for clinical application. We demonstrated that the ensemble model is fairer across sex and age compared with a penalized logistic regression, and therefore we recommend the use of well-tested ensembles despite a more complex explainability.
更多查看译文
关键词
machine learning,prediction,psychiatry,suicide,suicide attempt
AI 理解论文
溯源树
样例
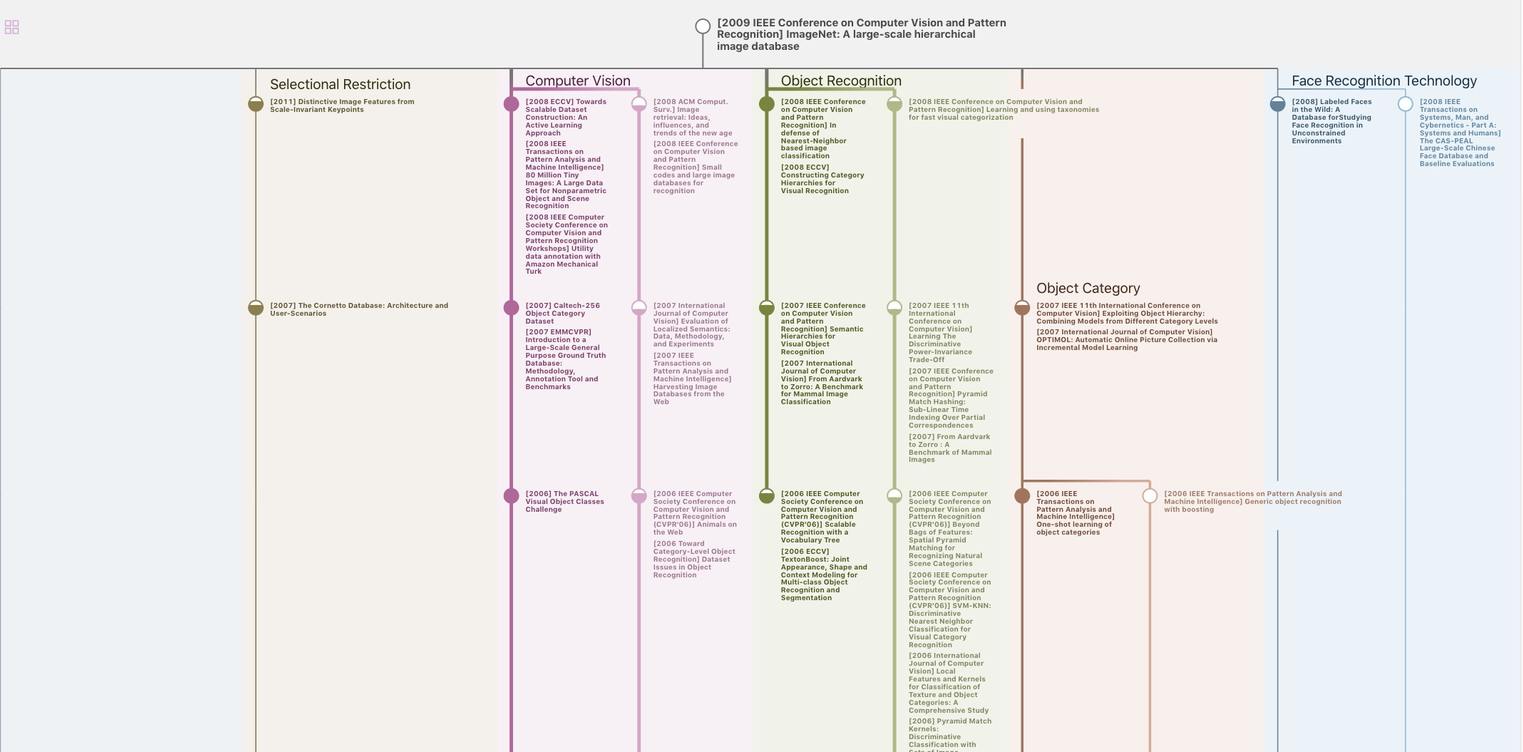
生成溯源树,研究论文发展脉络
Chat Paper
正在生成论文摘要