Gradient synchronization for multivariate functional data, with application to brain connectivity
JOURNAL OF THE ROYAL STATISTICAL SOCIETY SERIES B-STATISTICAL METHODOLOGY(2024)
摘要
Quantifying the association between components of multivariate random curves is of general interest and is a ubiquitous and basic problem that can be addressed with functional data analysis. An important application is the problem of assessing functional connectivity based on functional magnetic resonance imaging (fMRI), where one aims to determine the similarity of fMRI time courses that are recorded on anatomically separated brain regions. In the functional brain connectivity literature, the static temporal Pearson correlation has been the prevailing measure for functional connectivity. However, recent research has revealed temporally changing patterns of functional connectivity, leading to the study of dynamic functional connectivity. This motivates new similarity measures for pairs of random curves that reflect the dynamic features of functional similarity. Specifically, we introduce gradient synchronization measures in a general setting. These similarity measures are based on the concordance and discordance of the gradients between paired smooth random functions. Asymptotic normality of the proposed estimates is obtained under regularity conditions. We illustrate the proposed synchronization measures via simulations and an application to resting-state fMRI signals from the Alzheimer's Disease Neuroimaging Initiative and they are found to improve discrimination between subjects with different disease status.
更多查看译文
关键词
Alzheimer's disease,concordance,fMRI,functional connectivity,functional data analysis,Pearson correlation
AI 理解论文
溯源树
样例
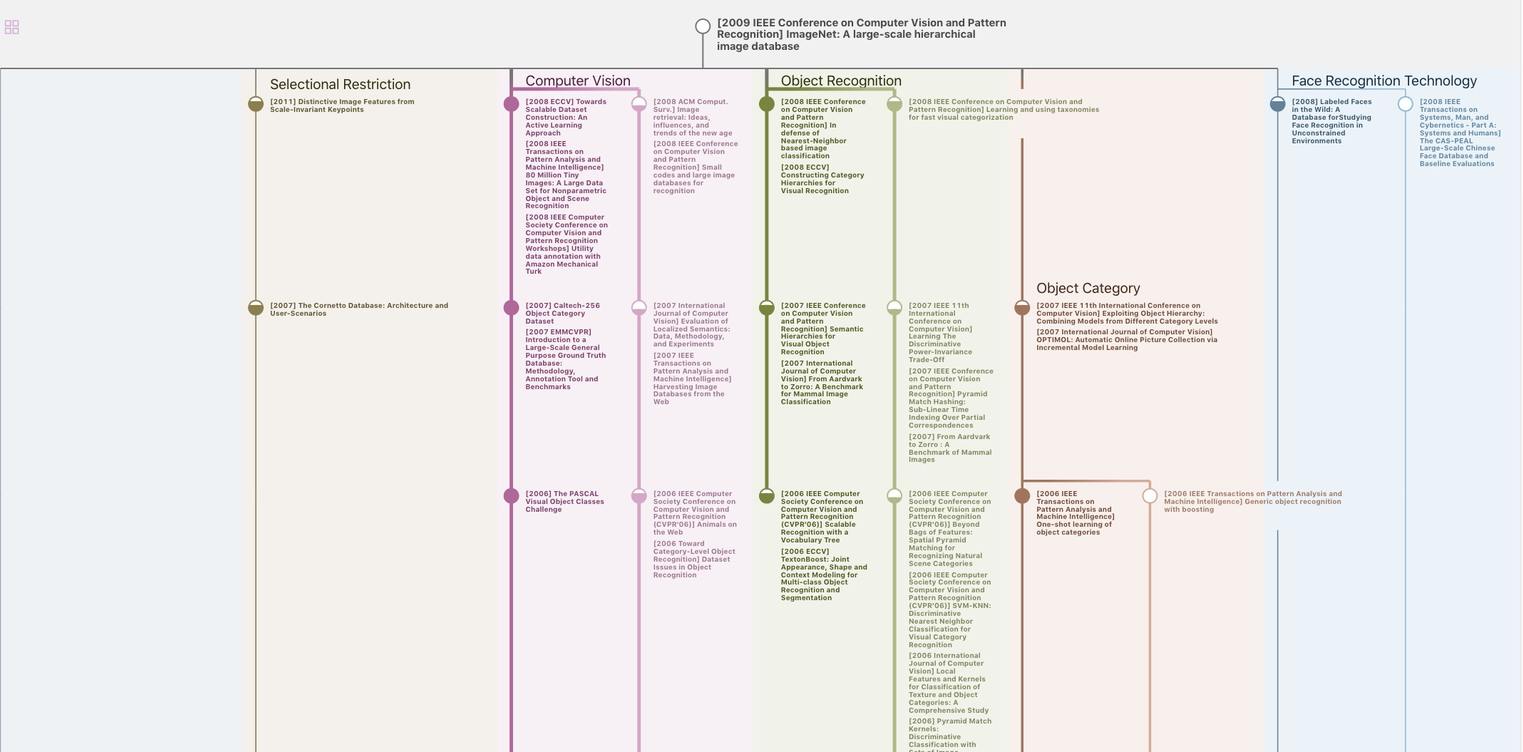
生成溯源树,研究论文发展脉络
Chat Paper
正在生成论文摘要