A hybrid CNN-vision transformer structure for remote sensing scene classification
REMOTE SENSING LETTERS(2024)
摘要
Vision Transformers (ViTs) have become one of the main architectures in deep learning with the self-attention mechanism, and are becoming an alternative to Convolutional Neural Networks (CNNs) for remote sensing scene classification tasks. However, the earlier self-attention layer of ViTs focuses on local features rather than global features, and the deeper self-attention layer focuses on global features but ignores the different impact of different frequency information. This will greatly increase the training and computational cost due to the quadratic complexity of the self-attention mechanism on the long sequence representation. In this paper, we propose a hybrid CNN - vision transformer structure (HCVNet), which uses convolutional layers to replace the earlier self-attention layers, and a novel Frequency Multi-head Self Attention (F-MSA) mechanism to replace the deeper self-attention layers. Specifically, F-MSA is a dual-stream structure that reduces the computational cost and improves the classification performance by encoding the high/low frequency information separately. In addition, a Semantic-aware Localization (SaL) module is introduced, which can guide the selection of crop by learning prior knowledge, avoiding the issue of pure background sampling. Our method performed an accuracy value of 97.20 +/- 0.02% on the Aerial Image Dataset and 93.89 +/- 0.03% on the NWPU-RESISC45 Dataset, with low complexity costs.
更多查看译文
关键词
Remote sensing image,scene classification,swin-transformer,convolutional neural network
AI 理解论文
溯源树
样例
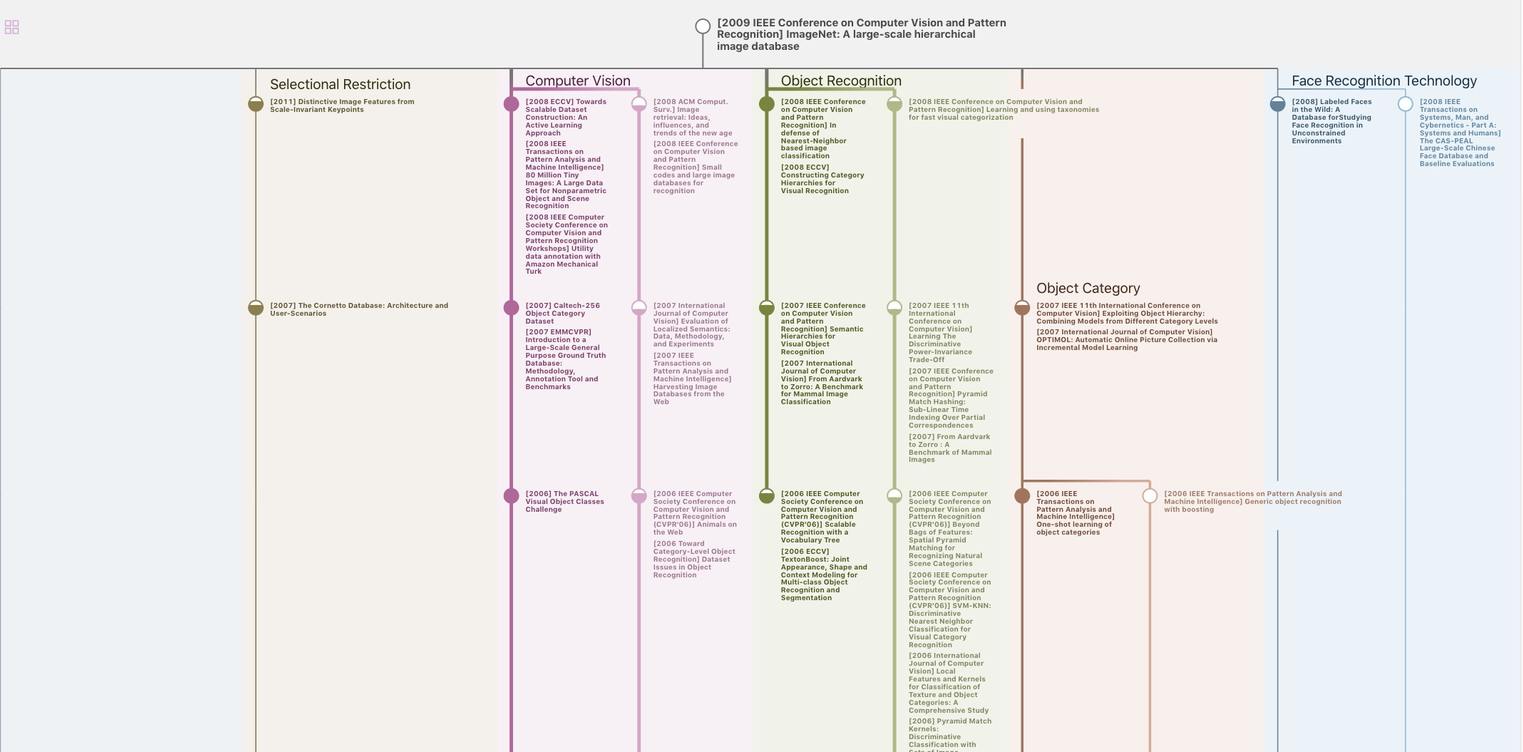
生成溯源树,研究论文发展脉络
Chat Paper
正在生成论文摘要