Impact of Uncertainties in Land Surface Processes on Subseasonal Predictability of Heat Waves Onset Over the Yangtze River Valley
JOURNAL OF GEOPHYSICAL RESEARCH-ATMOSPHERES(2024)
摘要
Land surface processes are strongly associated with heat waves (HWs). However, how the uncertainties in land surface processes owing to inaccurate physical parameters influence subseasonal HW predictions has rarely been explored. To examine the impact of parameter errors of land surface processes on the uncertainty of subseasonal HW predictions, five strong and long-lasting HW events over the middle and lower reaches of the Yangtze River are investigated. Based on the Weather Research and Forecasting model, the conditional nonlinear optimal perturbation related to parameters (CNOP-P) approach is employed to address the aforementioned issues. Numerical results demonstrate that the CNOP-P type errors of physical parameters cause large prediction errors for five HW event onsets. Two types of CNOP-Ps are obtained for HW events, called the type-1 CNOP-P and the type-2 CNOP-P. The type-1 (type-2) CNOP-P causes an approximately 3 degrees C (2 degrees C) warm (cold) bias during the HW period. Surface sensible and latent heat flux errors, especially flux exchange between vegetation canopy and canopy air, provide considerable uncertainty in subseasonal HW predictions. The type-1 (type-2) CNOP-P exhibits an underestimation (overestimation) of transpiration. Furthermore, it should be noted that the type-1 CNOP-P results in a substantial difference in soil moisture, a phenomenon that is demonstrated to be challenging to observe in the type-2 CNOP-P. The results indicate that understanding vegetation-atmosphere dynamics is crucial for improving subseasonal HW predictions. Jointly lowering soil-atmosphere and vegetation-atmosphere uncertainty can notably improve subseasonal HW prediction skills. Land surface processes play critical roles in subseasonal heat wave (HW) predictions. In this study, a rarely explored problem is examined: how uncertainties in land surface processes (i.e., model parameters) influence subseasonal HW predictions. The conditional nonlinear optimal perturbation related to parameters (CNOP-P) approach is employed to identify parameter errors causing the largest prediction errors. Five strong and long-lasting HW events over the middle and lower reaches of the Yangtze River (MLYR) are evaluated by the Weather Research and Forecasting model. There are two types of CNOP-Ps of HW events, named type-1 and type-2 CNOP-P. The type-1 CNOP-P overestimates the temperature, whereas the type-2 CNOP-P underestimates it. Both types of CNOP-Ps lead to notable forecast uncertainties. Surface sensible heat flux and latent heat flux errors are the main causes leading to large uncertainties in subseasonal HW predictions. Vegetation transpiration is important in the two types of CNOP-Ps. In contrast to the type-2 CNOP-P, the type-1 CNOP-P also underestimates soil evaporation, which results in soil moisture deficits. Low soil moisture conditions are critical for exacerbating HWs. This study indicates that accurately describing the soil-vegetation-atmosphere feedback processes is an effective way to improve the subseasonal prediction of severe HWs over the MLYR. The uncertainties in the physical parameters of the land surface process cause considerable errors in subseasonal heat wave (HW) predictionsThe combined effects of vegetation transpiration and soil evaporation are crucial for predictions of HW onset and evolutionThe conditional nonlinear optimal perturbation related to parameters method can be used to explore the subseasonal predictability of HWs
更多查看译文
关键词
extreme events,heat waves,CNOP-P,land surface,subseasonal,model uncertainties
AI 理解论文
溯源树
样例
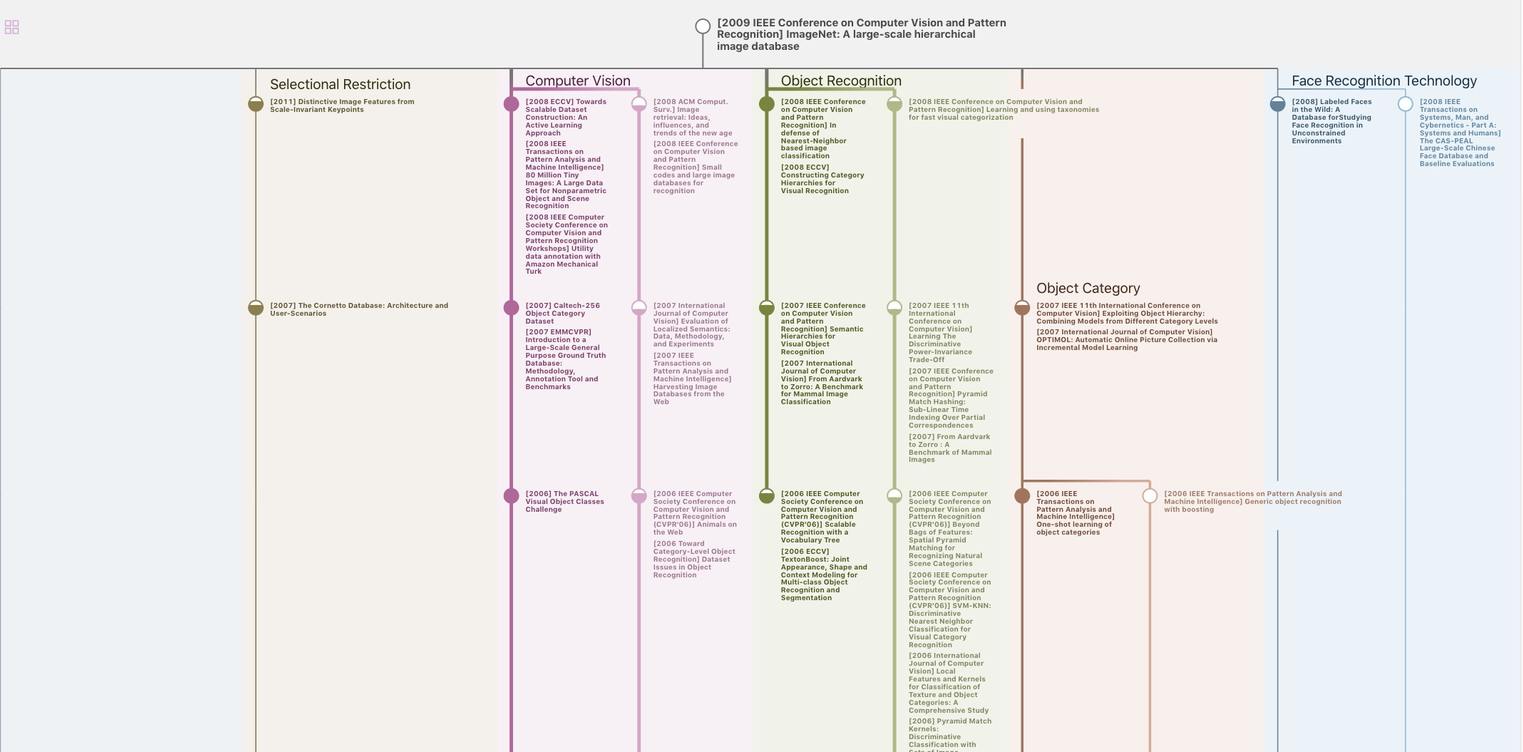
生成溯源树,研究论文发展脉络
Chat Paper
正在生成论文摘要