Decentralized convex optimization on time-varying networks with application to Wasserstein barycenters
COMPUTATIONAL MANAGEMENT SCIENCE(2024)
摘要
Inspired by recent advances in distributed algorithms for approximating Wasserstein barycenters, we propose a novel distributed algorithm for this problem. The main novelty is that we consider time-varying computational networks, which are motivated by examples when only a subset of sensors can observe each time step, and yet, the goal is to average signals (e.g., satellite pictures of some area) by approximating their barycenter. We embed this problem into a class of non-smooth dual-friendly distributed optimization problems over time-varying networks and develop a first-order method for this class. We prove non-asymptotic accelerated in the sense of Nesterov convergence rates and explicitly characterize their dependence on the parameters of the network and its dynamics. In the experiments, we demonstrate the efficiency of the proposed algorithm when applied to the Wasserstein barycenter problem.
更多查看译文
关键词
Distributed optimization,Dual oracle,Wasserstein barycenter,Time-varying networks,Consensus problem
AI 理解论文
溯源树
样例
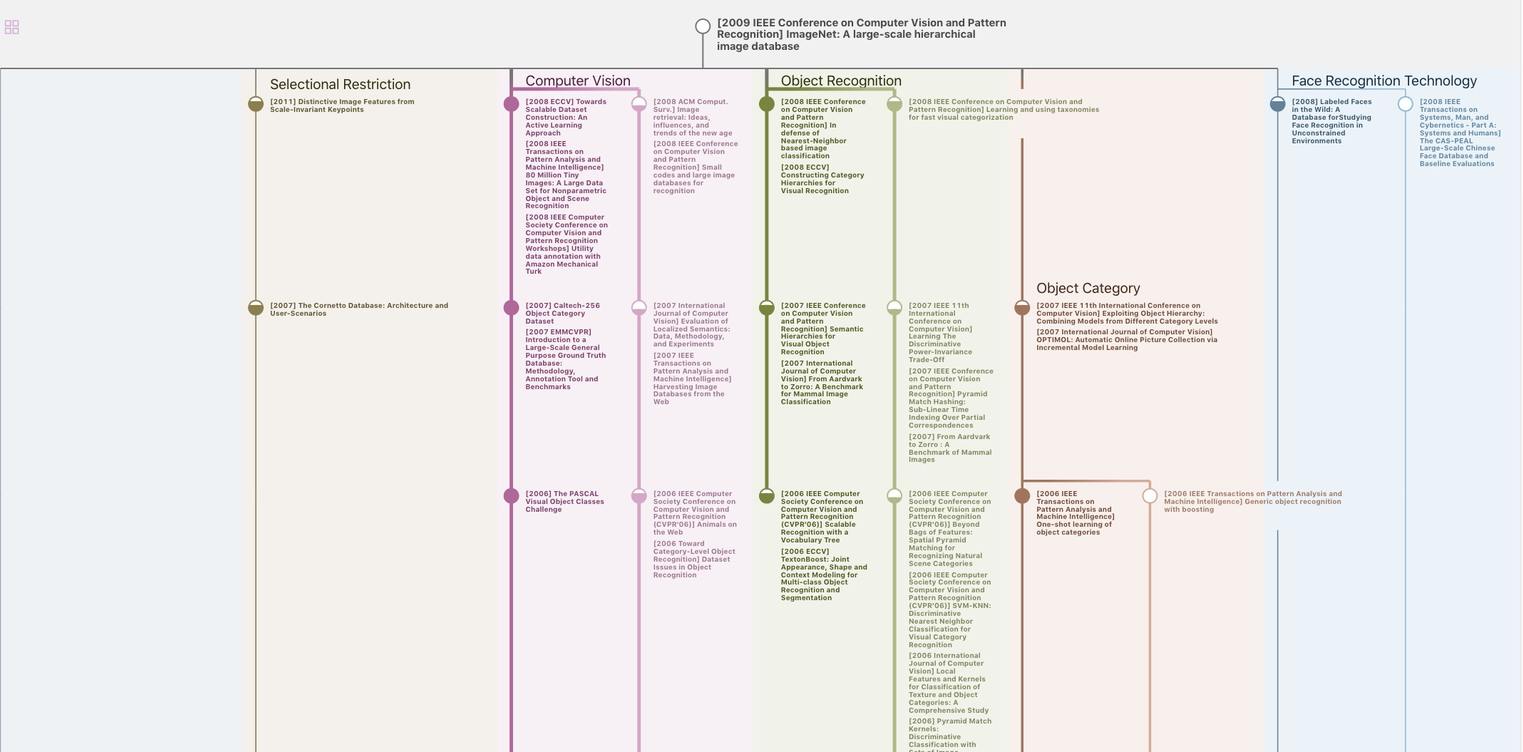
生成溯源树,研究论文发展脉络
Chat Paper
正在生成论文摘要