Artificial Intelligence-Enabled Crack Length Estimation From Acoustic Emission Signal Signatures
JOURNAL OF NONDESTRUCTIVE EVALUATION, DIAGNOSTICS AND PROGNOSTICS OF ENGINEERING SYSTEMS(2024)
摘要
This article addresses the classification of fatigue crack length using artificial intelligence (AI) applied to acoustic emission (AE) signals. The AE signals were collected during fatigue testing of two specimen types. One specimen type had a 1-mm hole for crack initiation. The other specimen type had a 150-mu m wide slit of various lengths. Fatigue testing was performed under stress intensity factor control to moderate crack advancement. The slit specimen produced AE signals only from crack advancement at the slit tips, whereas the 1-mm hole specimens produced AE signals from both crack tip advancement and crack rubbing or clapping. The AE signals were captured with a piezoelectric wafer active sensor (PWAS) array connected to MISTRAS instrumentation and aewin software. The collected AE signals were preprocessed using time-of-flight filtering and denoising. Choi Williams transform converted time domain AE signals into spectrograms. To apply machine learning, the spectrogram images were used as input data for the training, validation, and testing of a GoogLeNet convolutional neural network (CNN). The CNN was trained to sort the AE signals into crack length classes. CNN performance enhancements, including synthetic data generation and class balancing, were developed. A three-class example with crack lengths of (i) 10-12 mm, (ii) 12-14 mm, and (iii) 14-16 mm is provided. Our AI approach was able to classify the AE signals into these three classes with 91% accuracy, thus proving that the AE signals contain sufficient information for crack estimation using an AI-enabled approach.
更多查看译文
关键词
acoustic emission,damage classification,diagnostic feature extraction,elastic wave,prognosis,sensors,ultrasonics
AI 理解论文
溯源树
样例
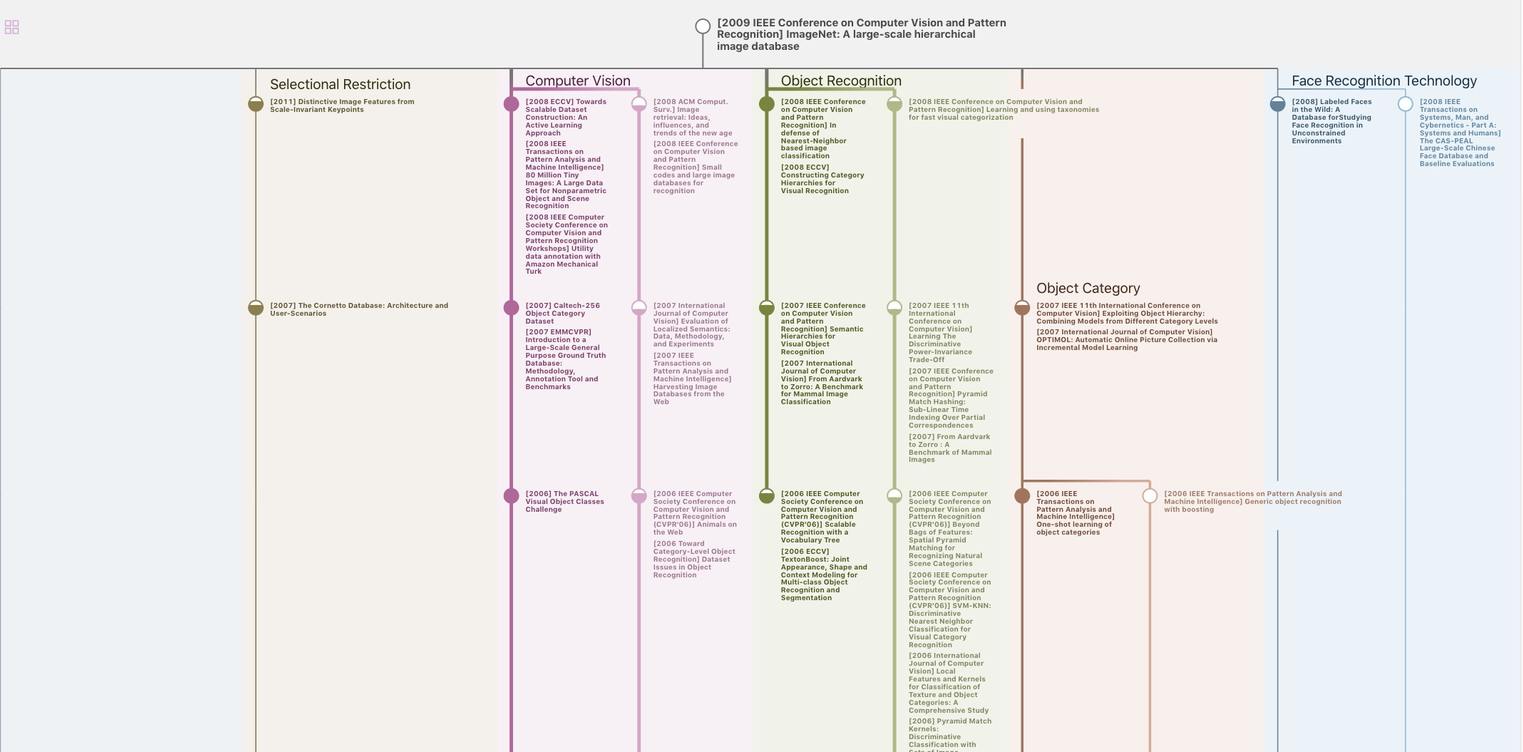
生成溯源树,研究论文发展脉络
Chat Paper
正在生成论文摘要