Operational modal analysis of under-determined system based on Bayesian CP decomposition (Translated)
MECHANICAL ENGINEERING JOURNAL(2024)
摘要
Modal parameters such as natural frequencies, modal shapes, and the damping ratio are useful to understand structural dynamics of mechanical systems. Modal parameters need to be estimated under operational conditions for use in structural health monitoring. Therefore, operational modal analysis (OMA) without input signals has been proposed to easily extract modal parameters under operational conditions. Recently, OMA for under-determined systems with more active modes than measurement outputs has been investigated to reduce the number of sensors. This study proposes the OMA framework for under-determined systems based on Bayesian CP (CANDECOMP/PARAFAC) decomposition of second-order statistical data. The proposed method enables us to extract the modal parameters from under-determined systems without tuning the number of active modes, because the rank of the tensor data corresponding to the number of active modes is automatically determined via Bayesian inference. The effectiveness of this method is demonstrated using artificial vibration data of a mass-spring system under operational and under-determined conditions.
更多查看译文
关键词
position,CANDECOMP/PARAFAC decomposition,Bayesian inference,Under-determined problem,Second-order blind identification
AI 理解论文
溯源树
样例
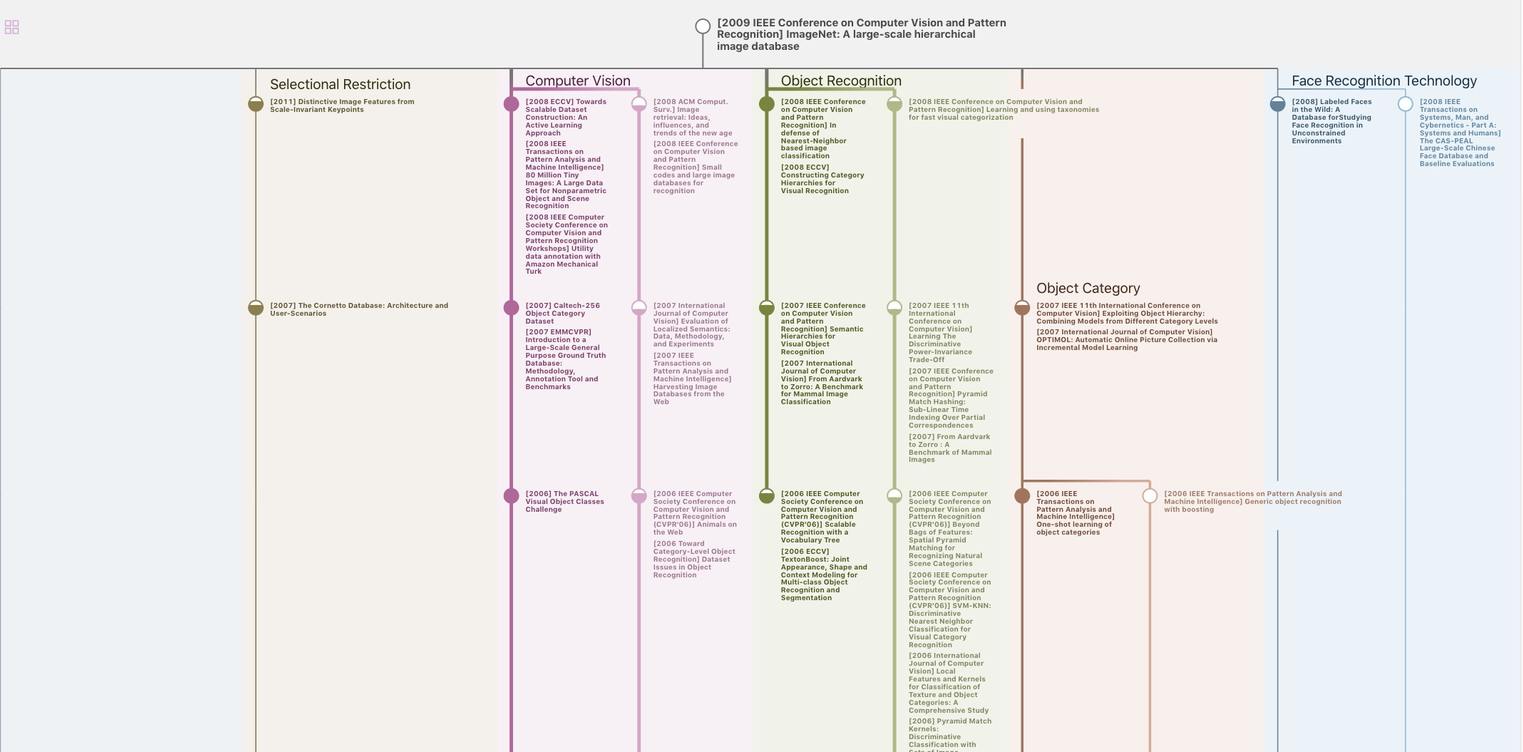
生成溯源树,研究论文发展脉络
Chat Paper
正在生成论文摘要