Prototypical Network with Residual Attention for Modulation Classification of Wireless Communication Signals
ELECTRONICS(2023)
摘要
Automatic modulation classification (AMC) based on data-driven deep learning (DL) can achieve excellent classification performance. However, in the field of electronic countermeasures, it is difficult to extract salient features from wireless communication signals under scarce samples. Aiming at the problem of modulation classification under scarce samples, this paper proposes a few-shot learning method using prototypical network (PN) with residual attention (RA), namely PNRA, to achieve the AMC. Firstly, the RA is utilized to extract the feature vector of wireless communication signals. Subsequently, the feature vector is mapped to a new feature space. Finally, the PN is utilized to measure the Euclidean distance between the feature vector of the query point and each prototype in this space, determining the type of the signals. In comparison to mainstream few-shot learning (FSL) methods, the proposed PNRA can achieve effective and robust AMC under the data-hungry condition.
更多查看译文
关键词
automatic modulation classification,few-shot learning,residual attention,prototypical network
AI 理解论文
溯源树
样例
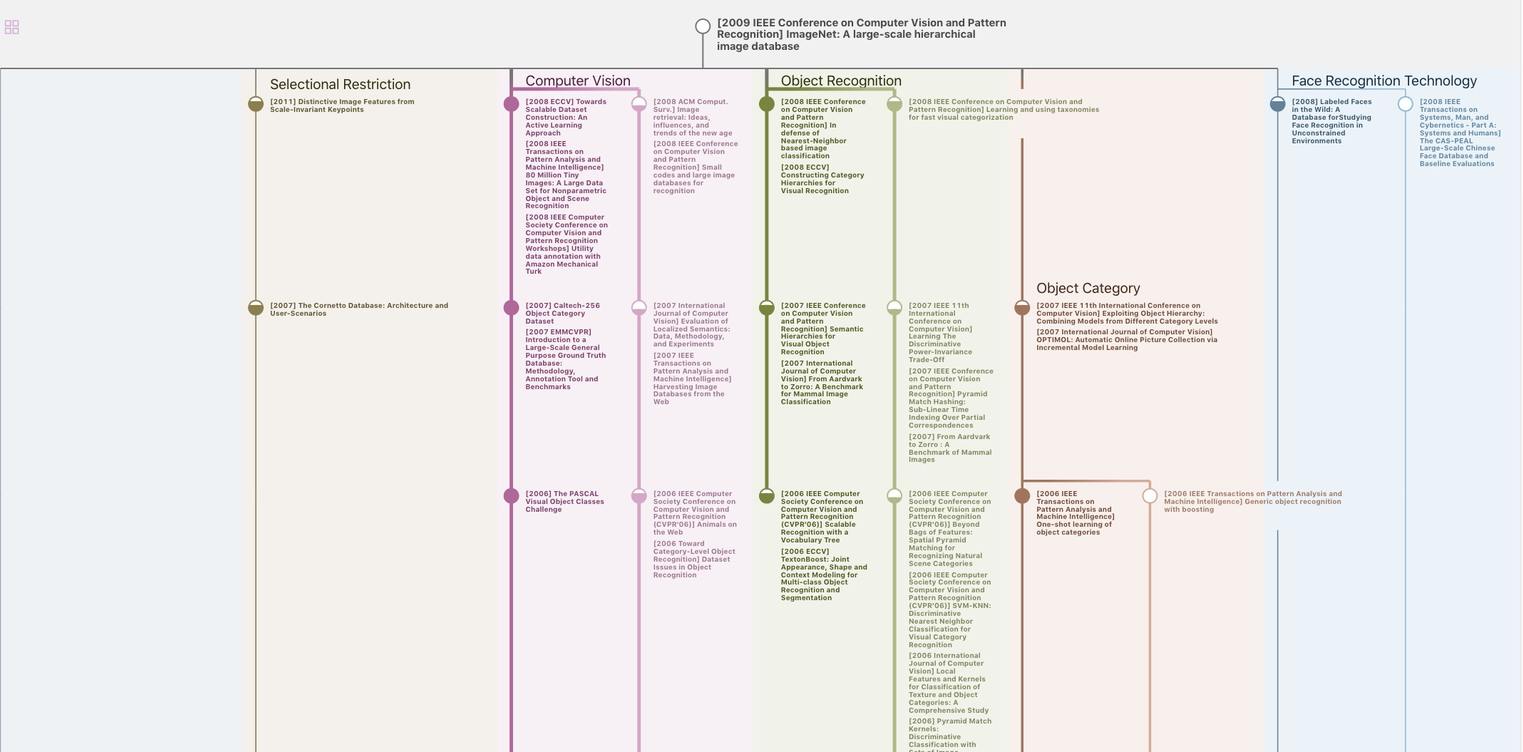
生成溯源树,研究论文发展脉络
Chat Paper
正在生成论文摘要