Feature selection and framework design toward data-driven predictive sustainability assessment and optimization for additive manufacturing
TRANSACTIONS OF THE CANADIAN SOCIETY FOR MECHANICAL ENGINEERING(2024)
摘要
Additive manufacturing (AM) is considered an innovative technology to fabricate goods with green characteristics. In com-parison to conventional manufacturing approaches, AM technologies have shown promising results in enhancing sustain-ability in production systems. Various research has been conducted to assess the environmental impacts of AM based on the well-known life cycle assessment (LCA) framework. However, this approach requires intensive domain knowledge to build the environmental impact model and interpret the findings. This knowledge barrier may cause delays and challenges in the selection of the optimal design and process parameters for additively manufactured parts. Such challenges can be particu-larly prevalent during the early product design and planning stages. As such, the research community demands an automated LCA tool to support AM toward elevated sustainability. To achieve this ambitious goal, this paper particularly investigates the fundamental question--"What are the key influential parameters that pose an impact on the environmental sustainability of AM?" Thus, this paper proposes a methodological framework for identifying the key influential parameters for AM. The framework was demonstrated by taking the fused filament fabrication process as a case study. Through instantiating various parts within the proposed framework and conducting LCA on over 200 AM instances, followed by correlation analysis, the key influential parameters were identified. Consequently, a data-driven predictive sustainability assessment and optimization framework was developed by integrating the identified influential features.
更多查看译文
关键词
additive manufacturing,environmental sustainability,life cycle assessment,machine learning,feature selection
AI 理解论文
溯源树
样例
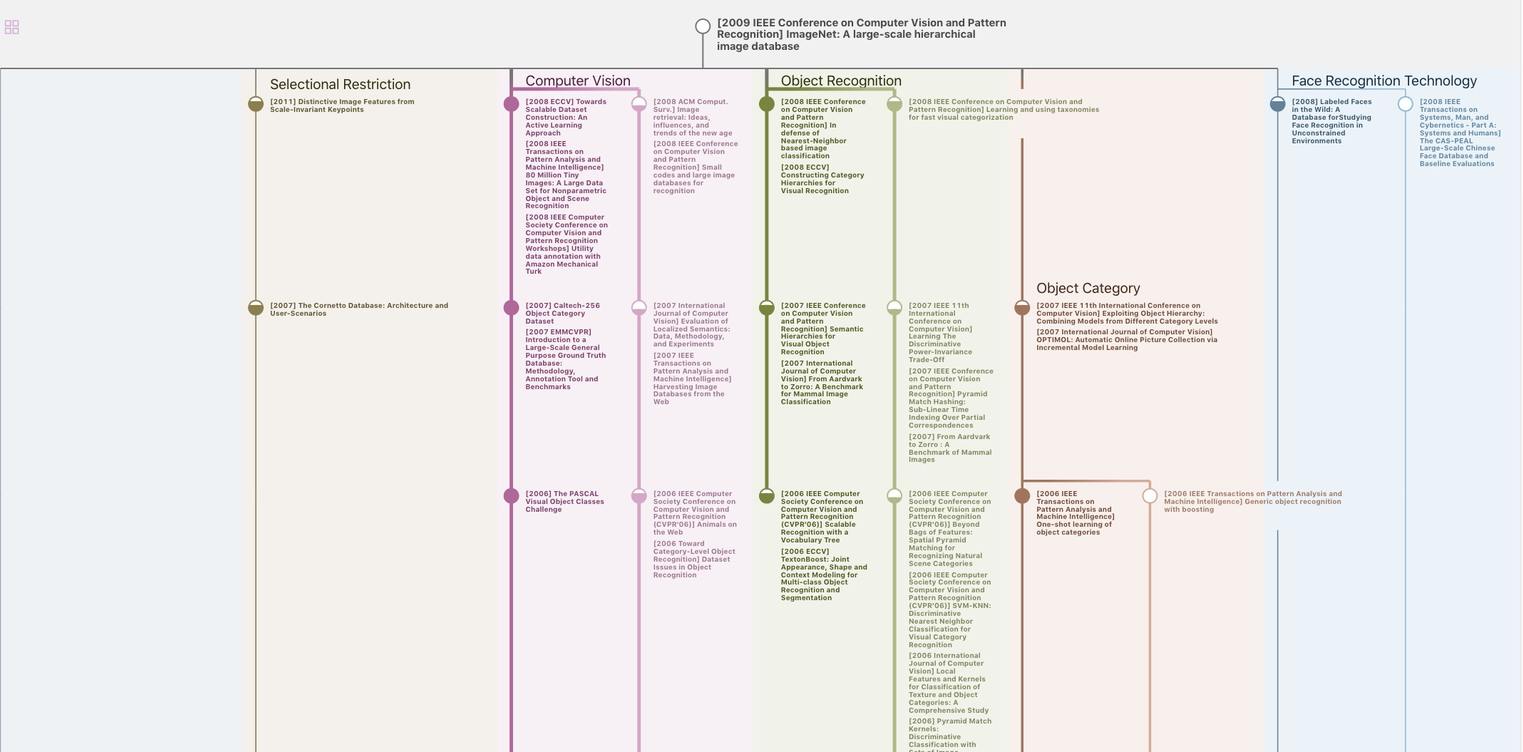
生成溯源树,研究论文发展脉络
Chat Paper
正在生成论文摘要