Statistical landslide susceptibility assessment using Bayesian logistic regression and Markov Chain Monte Carlo (MCMC) simulation with consideration of model class selection
GEORISK-ASSESSMENT AND MANAGEMENT OF RISK FOR ENGINEERED SYSTEMS AND GEOHAZARDS(2024)
摘要
Landslide susceptibility mapping (LSM) plays an essential role in landslide management and contributes to decision-makers and planners to formulate landslide prevention policies. It is often carried out by predicting possibility of landslide occurrence first from numerous landslides conditioning factors (LCFs), followed by partitioning areas with different landslide susceptibility levels. Numerous methods have been proposed for such a purpose, saying logistic regression (LR), deep learning methods, etc. Among these methods, LR is the most widely used in literature, which may be attributed to its good performance and easy-to-follow. However, few studies explore uncertainty and reliability of the LR in LSM. Furthermore, not all LCFs contribute significantly to the landslide occurrence, saying elevation, distance to roads, etc. How to objectively determine the most relevant LCFs is another issue that remains unsolved. This study proposes a Bayesian LR method for landslide susceptibility assessment (LSA), together with Markov Chain Monte Carlo (MCMC) simulation for parameter estimation. MCMC samples are used to determine the optimal model, and to quantify the uncertainty associated with the LSM. Real-life data from Shaanxi Province are used for illustration. Results show that the proposed method works reasonably well in determination of the optimal model and in uncertainty quantification in LSM.
更多查看译文
关键词
Geo-hazards mapping,Bayesian model class selection,uncertainty quantification,importance sampling,uncertainty propagation
AI 理解论文
溯源树
样例
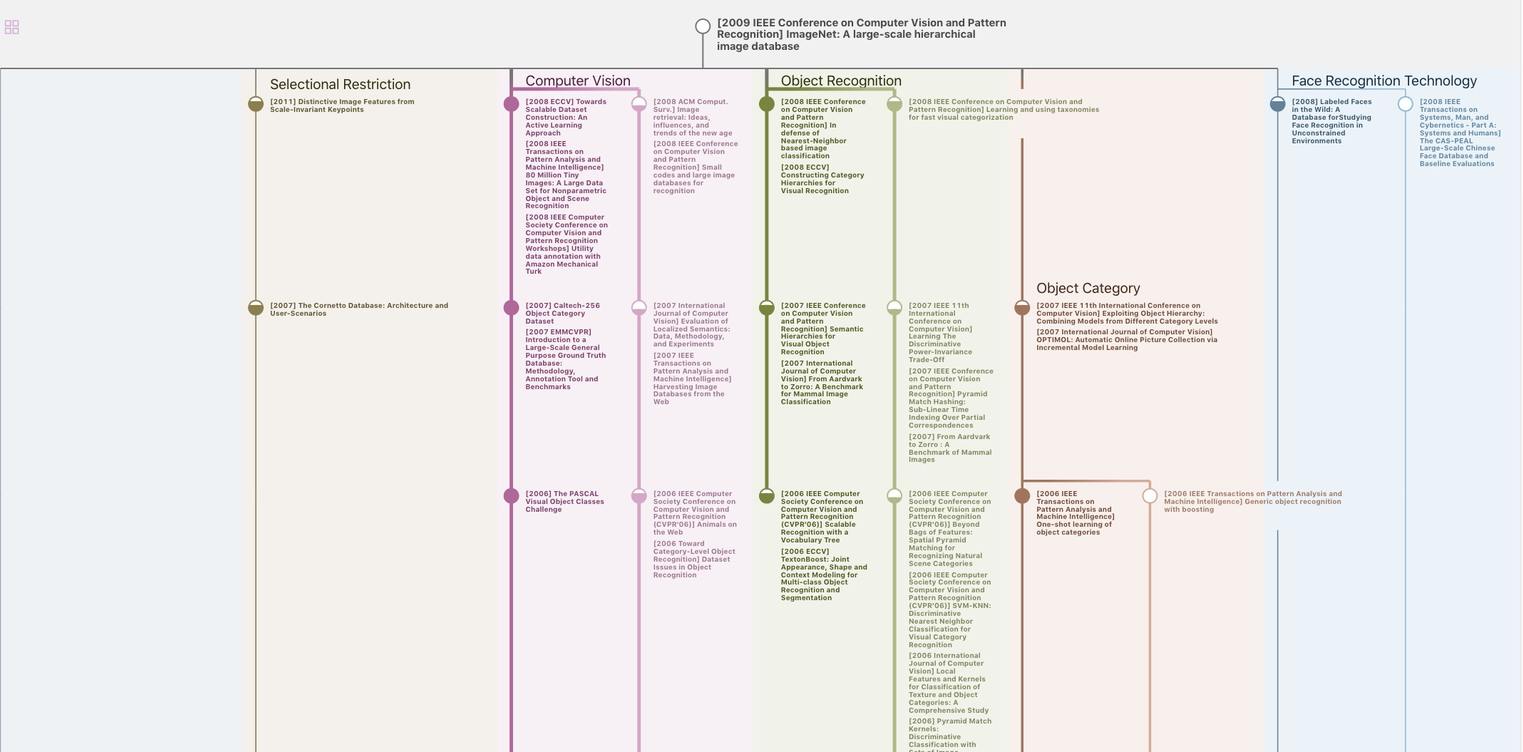
生成溯源树,研究论文发展脉络
Chat Paper
正在生成论文摘要