A Siamese CNN-BiLSTM-based method for unbalance few-shot fault diagnosis of rolling bearings
MEASUREMENT & CONTROL(2023)
摘要
Small and imbalanced fault samples have a profound impact on the diagnostic performance of a model in the process of locating and quantifying the rolling bearing damage of aeroengines in practice. Therefore, a Siamese Convolutional Neural Network-Bidirectional Long Short-Term Memory (CNN-BiLSTM) model was proposed in this paper. Random selection and cross combination methods were used to augment and balance sample sizes at first. Then, two weight-sharing CNN-BiLSTM models were used for adaptive extraction and distance measurement of weak fault features. Finally, the fault classification was performed based on feature distance. Model performance was verified using simulated fault test data of rolling bearings. The results showed that the Siamese CNN-BiLSTM model could achieve an accuracy of up to 96.0% for quantitative diagnosis and 98.0% for location diagnosis. This model was also capable of solving the imbalanced classification of samples and made it possible to transfer between different rotating speeds and working conditions.
更多查看译文
关键词
Rolling bearing,Siamese CNN-BiLSTM model,small and imbalanced samples,fault diagnosis
AI 理解论文
溯源树
样例
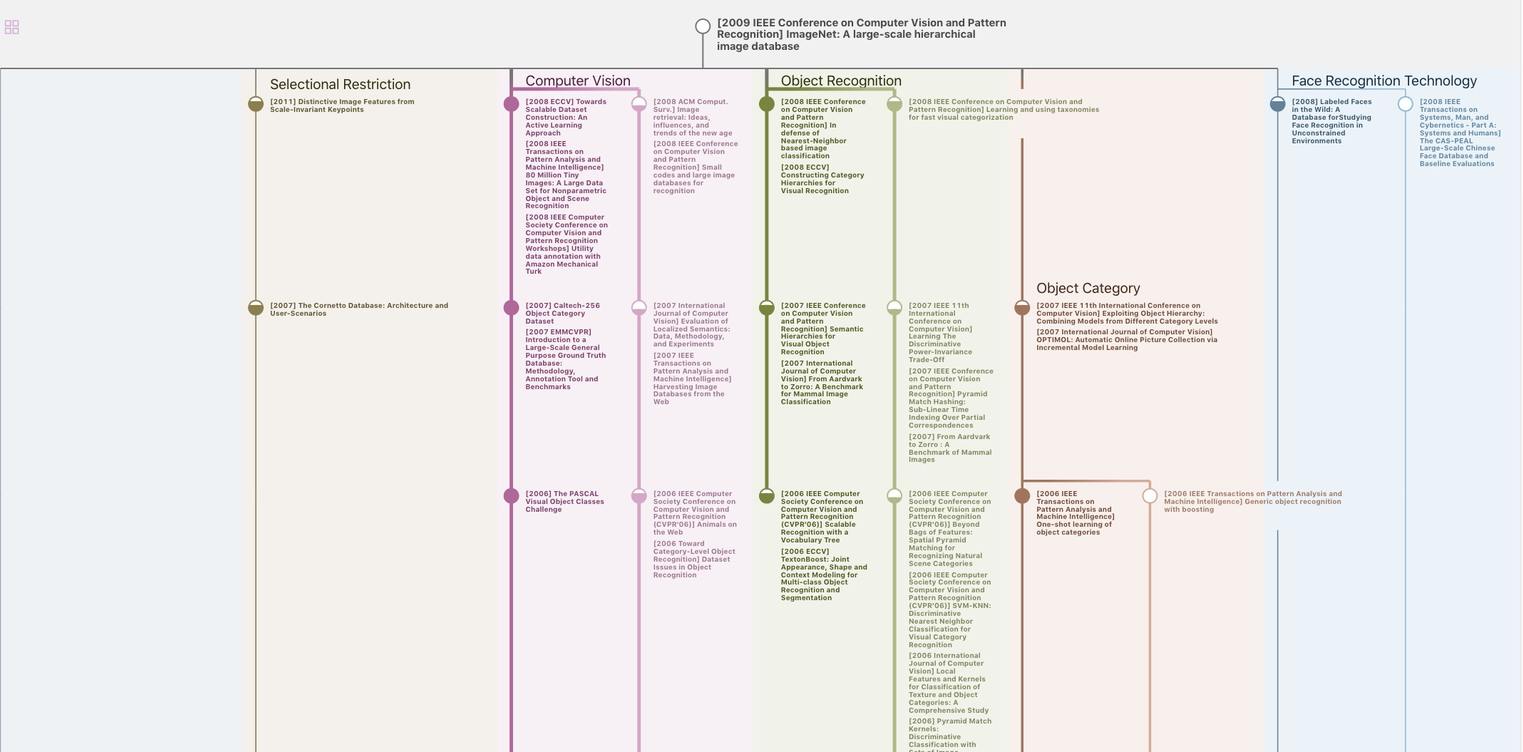
生成溯源树,研究论文发展脉络
Chat Paper
正在生成论文摘要