Optimized operation scheme of flash-memory-based neural network online training with ultra-high endurance
JOURNAL OF SEMICONDUCTORS(2024)
摘要
With the rapid development of machine learning, the demand for high-efficient computing becomes more and more urgent. To break the bottleneck of the traditional Von Neumann architecture, computing-in-memory (CIM) has attracted increasing attention in recent years. In this work, to provide a feasible CIM solution for the large-scale neural networks (NN) requiring continuous weight updating in online training, a flash-based computing-in-memory with high endurance (10(9) cycles) and ultra-fast programming speed is investigated. On the one hand, the proposed programming scheme of channel hot electron injection (CHEI) and hot hole injection (HHI) demonstrate high linearity, symmetric potentiation, and a depression process, which help to improve the training speed and accuracy. On the other hand, the low-damage programming scheme and memory window (MW) optimizations can suppress cell degradation effectively with improved computing accuracy. Even after 10(9) cycles, the leakage current (I-off) of cells remains sub-10pA, ensuring the large-scale computing ability of memory. Further characterizations are done on read disturb to demonstrate its robust reliabilities. By processing CIFAR-10 tasks, it is evident that similar to 90% accuracy can be achieved after 10(9) cycles in both ResNet50 and VGG16 NN. Our results suggest that flash-based CIM has great potential to overcome the limitations of traditional Von Neumann architectures and enable high-performance NN online training, which pave the way for further development of artificial intelligence (AI) accelerators.
更多查看译文
关键词
NOR flash memory,computing-in-memory,endurance,neural network,online training
AI 理解论文
溯源树
样例
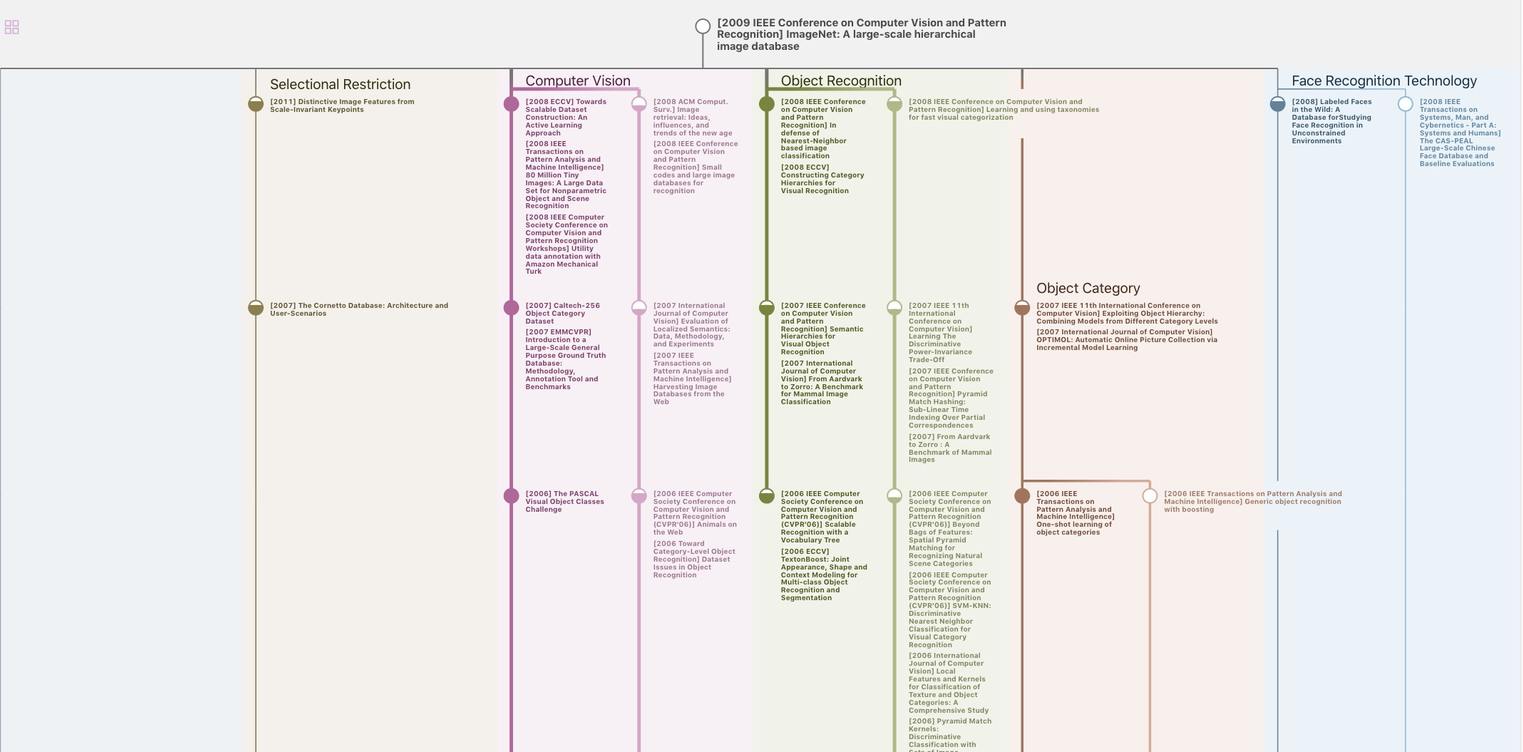
生成溯源树,研究论文发展脉络
Chat Paper
正在生成论文摘要