Causal-mechanical explanations in biology: Applying automated assessment for personalized learning in the science classroom
JOURNAL OF RESEARCH IN SCIENCE TEACHING(2024)
摘要
One of the core practices of science is constructing scientific explanations. However, numerous studies have shown that constructing scientific explanations poses significant challenges to students. Proper assessment of scientific explanations is costly and time-consuming, and teachers often do not have a clear definition of the educational goals for formulating scientific explanations. Consequently, teachers struggle to support their students in this process. It is hoped that recent advances in machine learning (ML) and its application to educational technologies can assist teachers and learners in analyzing student responses and providing automated formative feedback according to well-defined pedagogical criteria. In this study, we present a method to automate the entire assessment-feedback process. First, we developed a causal-mechanical (CM)-based grading rubric and applied it to student responses to two open-ended items. Second, we used unsupervised ML tools to identify patterns in student responses. Those patterns enable the definition of "meta-categories" of explanation types and the design of personalized feedback adapted to each category. Third, we designed an in-class intervention with personalized formative feedback that matches the response patterns. We used natural language processing and ML algorithms to assess students' explanations and provide feedback. Findings from a controlled experiment demonstrated that a CM-based grading scheme can be used to identify meaningful patterns and inform the design of formative feedback that promotes student ability to construct explanations in biology. We discuss possible implications for automated assessment and personalized teaching and learning of scientific writing in K-12 science education.
更多查看译文
关键词
automated assessment,formative feedback,machine learning,personalized learning,scientific explanations
AI 理解论文
溯源树
样例
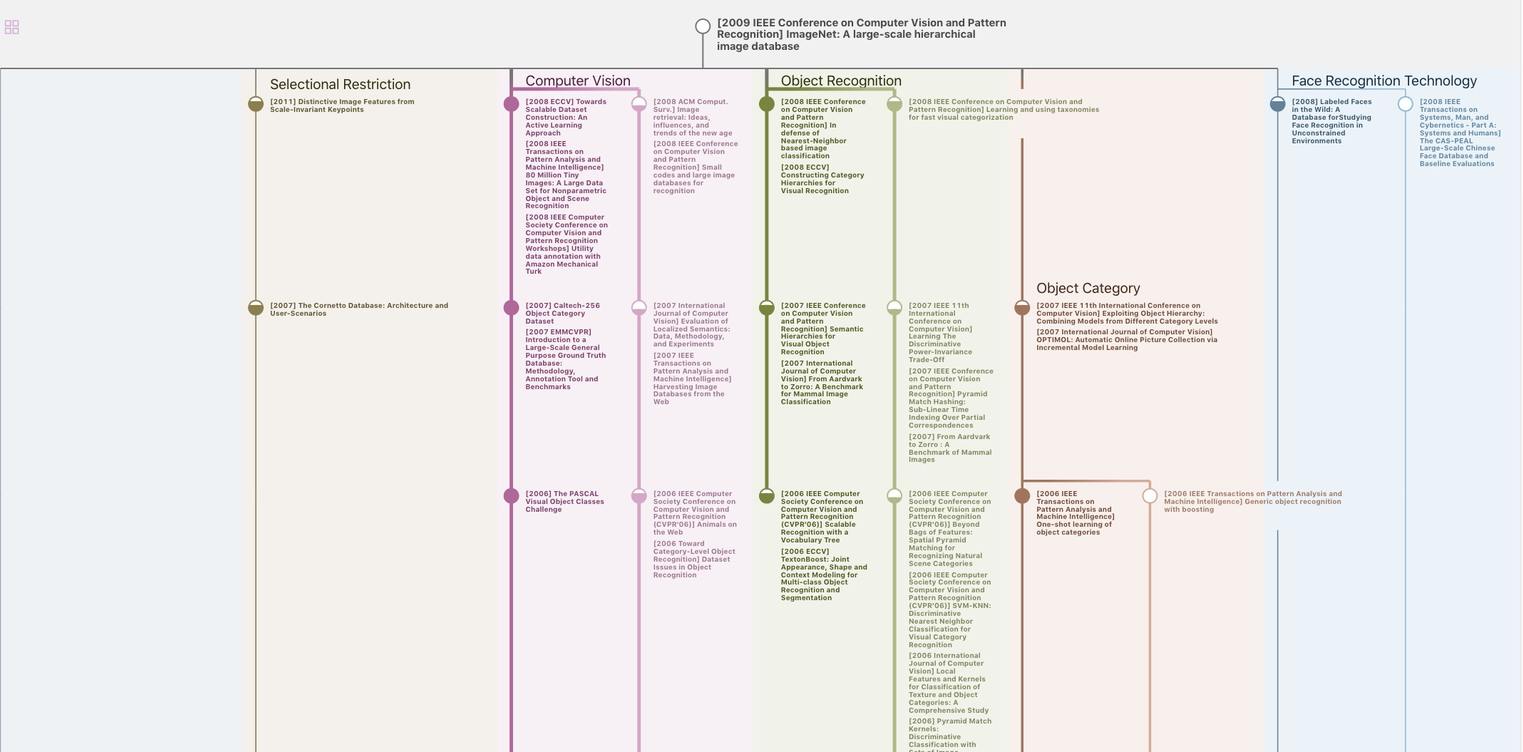
生成溯源树,研究论文发展脉络
Chat Paper
正在生成论文摘要