Accelerating Materials Discovery: Automated Identification of Prospects from X-Ray Diffraction Data in Fast Screening Experiments
ADVANCED INTELLIGENT SYSTEMS(2024)
摘要
New materials are frequently synthesized and optimized with the explicit intention to improve their properties to meet the ever-increasing societal requirements for high-performance and energy-efficient electronics, new battery concepts, better recyclability, and low-energy manufacturing processes. This often involves exploring vast combinations of stoichiometries and compositions, a process made more efficient by high-throughput robotic platforms. Nonetheless, subsequent analytical methods are essential to screen the numerous samples and identify promising material candidates. X-ray diffraction is a commonly used analysis method available in most laboratories which gives insight into the crystalline structure and reveals the presence of phases in a powder sample. Herein, a method for automating the analysis of XRD patterns, which uses a neural network model to classify samples into nondiffracting, single-phase, and multi-phase structures, is presented. To train neural networks for identifying materials with compositions not matching known crystallographic structures, a synthetic data generation approach is developed. The application of the neural networks on high-entropy oxides experimental data is demonstrated, where materials frequently deviate from anticipated structures. Our approach, not limited to these materials, seamlessly integrates into high-throughput data analysis pipelines, either filtering acquired patterns or serving as a standalone method for automated material exploration workflows. A neural network is used for automatic classification of X-ray diffraction scans and categorizes single-phase, multi-phase, and amorphous samples. The network is trained based on simulated patterns that are generated from a cif-file; no further modifications are necessary to use this system. Hence, this system can be used for rapid screening of vast composition spaces.image (c) 2023 WILEY-VCH GmbH
更多查看译文
关键词
deep learning,fast screening,high throughputs,X-ray diffraction
AI 理解论文
溯源树
样例
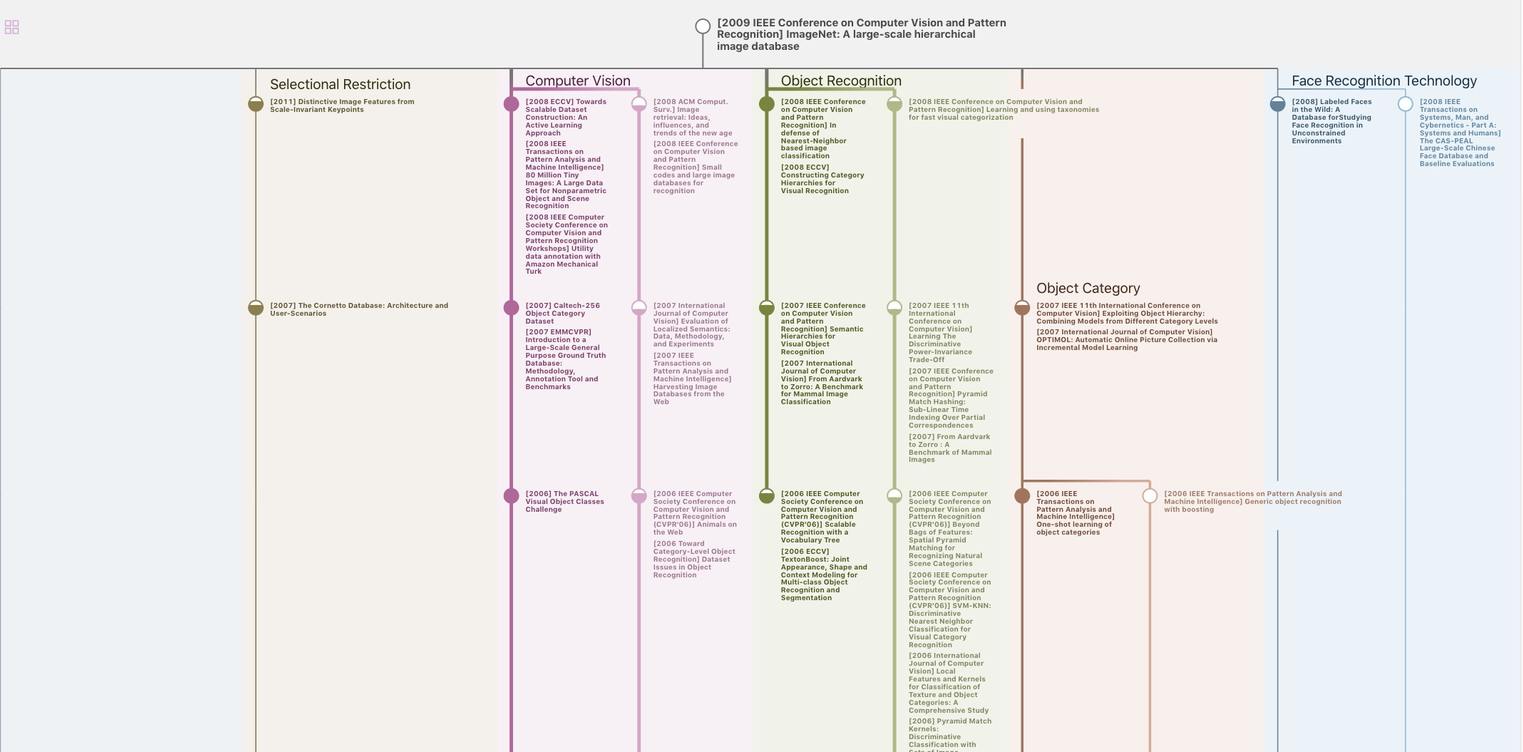
生成溯源树,研究论文发展脉络
Chat Paper
正在生成论文摘要