Multi-Level Prediction Framework of Driving Risk Based on the Matter-Element Extension Model
TRANSPORTATION RESEARCH RECORD(2024)
摘要
Surrogate safety measures (SSMs) can be used alone or in combination to assess vehicle conflicts. However, considering the complexity and uncertainty of the traffic environment, the road traffic factors that can be accounted for are still limited by combining two or more SSMs. This study proposes a driving risk prediction framework based on a modified matter-element extension model (MEEM), which integrates a set of carefully selected indicators at the macro-road-section level, the meso-vehicle-group level, and the micro-adjacent-vehicle level, to comprehensively reflect the factors affecting road traffic safety in temporal, spatial, and vehicular dimensions. To validate the proposed framework, lane-changing trajectories from the highD dataset are extracted to obtain the multi-level indicators and clustered risk levels. Different combinations of the multi-level indicators in different proportions are examined. The results show that the multi-level indicator combinations outperform those with micro-level indicators only, and optimal prediction performance is achieved when the ratio of meso- and micro-level indicators approaches 4:6. Furthermore, different time advances of the macro-level indicators are compared and analyzed considering flow propagation effect. It is found that an appropriate setting of the time advance in the calculation of macro-level indicators can help improve the accuracy of risk prediction. Finally, compared with the baseline algorithm random forest, MEEM generally shows better prediction performance at different prediction time, indicating that the multi-level indicators can be more effectively fused to assess risk using the proposed MEEM.
更多查看译文
关键词
safety,safety performance and analysis,crash prediction models,modeling and forecasting,transportation safety management systems
AI 理解论文
溯源树
样例
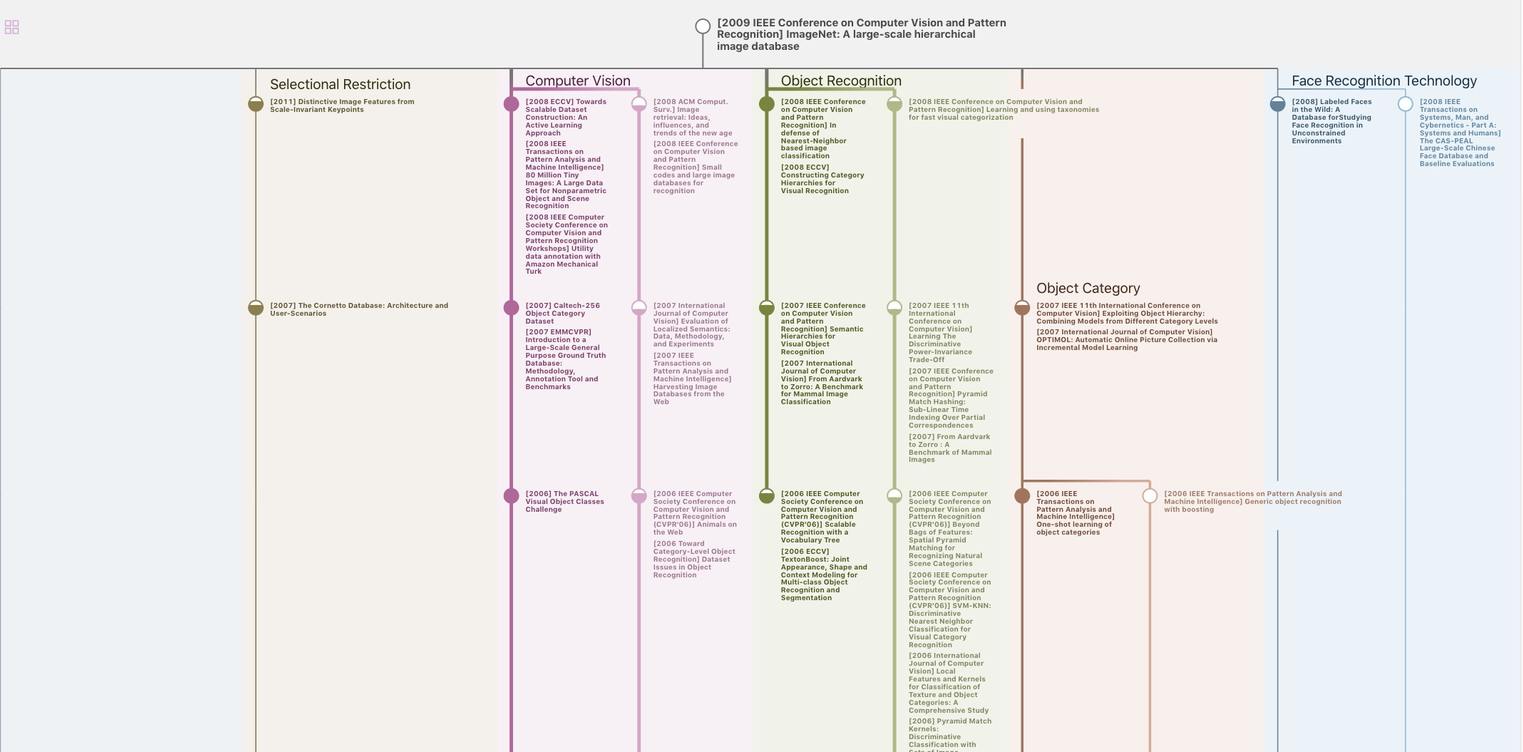
生成溯源树,研究论文发展脉络
Chat Paper
正在生成论文摘要