Multi-Branch Spectral Channel Attention Network for Breast Cancer Histopathology Image Classification
ELECTRONICS(2024)
摘要
Deep-learning-based breast cancer image diagnosis is currently a prominent and growingly popular area of research. Existing convolutional-neural-network-related methods mainly capture breast cancer image features based on spatial domain characteristics for classification. However, according to digital signal processing theory, texture images usually contain repeated patterns and structures, which appear as intense energy at specific frequencies in the frequency domain. Motivated by this, we make an attempt to explore a breast cancer histopathology classification application in the frequency domain and further propose a novel multi-branch spectral channel attention network, i.e., the MbsCANet. It expands the interaction of frequency domain attention mechanisms from a multi-branch perspective via combining the lowest frequency features with selected high frequency information from two-dimensional discrete cosine transform, thus preventing the loss of phase information and gaining richer context information for classification. We thoroughly evaluate and analyze the MbsCANet on the publicly accessible BreakHis breast cancer histopathology dataset. It respectively achieves the optimal image-level and patient-level classification results of 99.01% and 98.87%, averagely outperforming the spatial-domain-dominated models by a large margin, and visualization results also demonstrate the effectiveness of the MbsCANet for this medical image application.
更多查看译文
关键词
convolutional neural network,channel attention,frequency domain,breast cancer,histopathology image classification
AI 理解论文
溯源树
样例
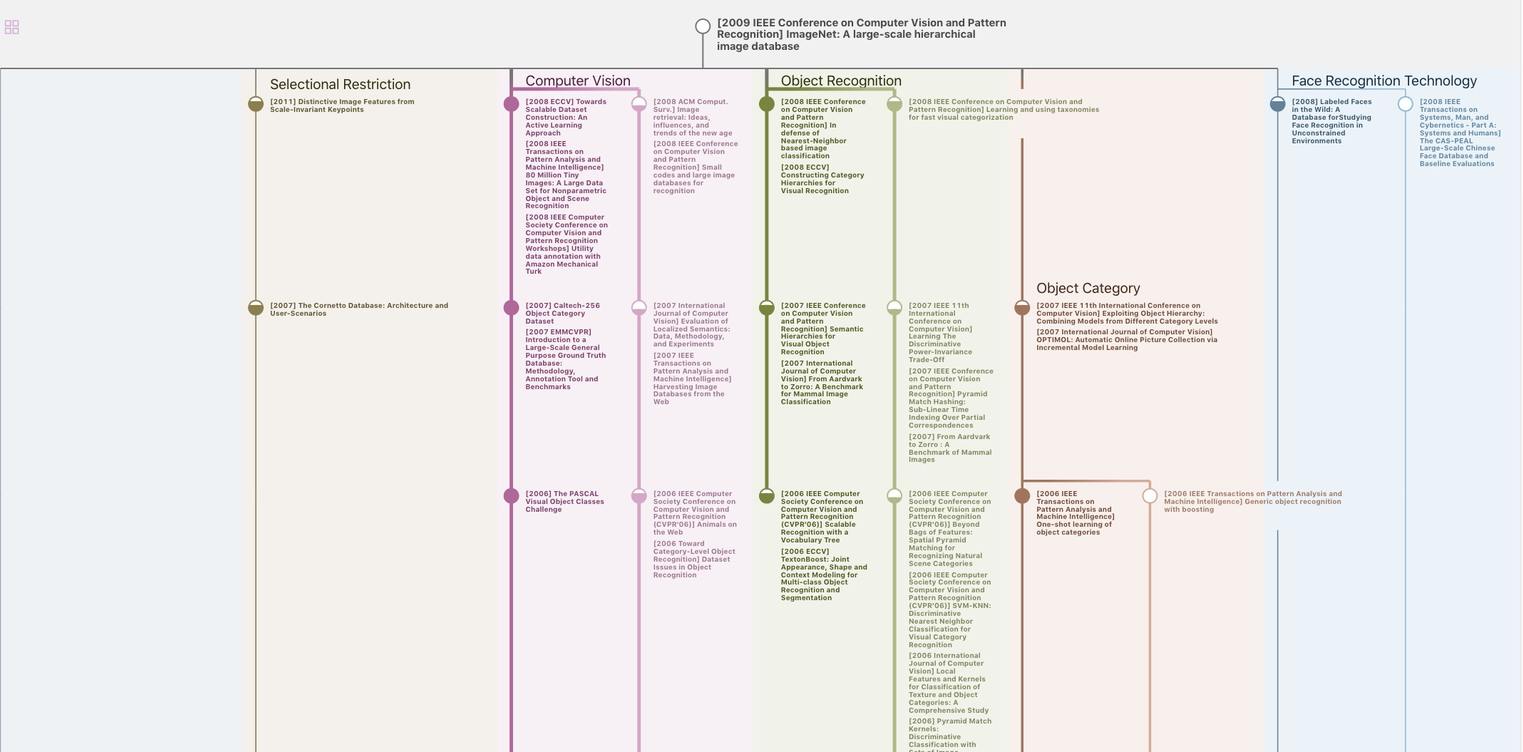
生成溯源树,研究论文发展脉络
Chat Paper
正在生成论文摘要