EEUR-Net: End-to-End Optimization of Under-Sampling and Reconstruction Network for 3D Magnetic Resonance Imaging
ELECTRONICS(2024)
摘要
It is time-consuming to acquire complete data by fully phase encoding in two orthogonal directions along with one frequency encoding direction. Under-sampling in the 3D k-space is promising in accelerating such 3D MRI process. Although 3D under-sampling can be conducted according to predefined probability density, the density-based method is not optimal. Because of the large amount of 3D data and computational cost, it is challenging to perform data-driven and learning-based 3D under-sampling and subsequent 3D reconstruction. To tackle this challenge, this paper proposes a deep neural network called EEUR-Net, realized by optimizing specific under-sampling patterns for the fully sampled 3D k-space data. Innovatively, our under-sampling algorithm employs an end-to-end deep learning approach to optimize phase encoding patterns and uses a 3D U-Net for image reconstruction of under-sampled data. Through end-to-end training, we obtain an optimized 3D under-sampling pattern, which significantly enhances the quality of the reconstructed image under the same acceleration factor. A series of experiments on a knee MRI dataset demonstrate that, in comparison to standard random uniform, radial, Poisson and equispaced Cartesian under-sampling schemes, our end-to-end learned under-sampling pattern considerably improves the reconstruction quality of under-sampled MRI images.
更多查看译文
关键词
deep learning,3D MRI,k-space under-sampling,image reconstruction,3D U-Net
AI 理解论文
溯源树
样例
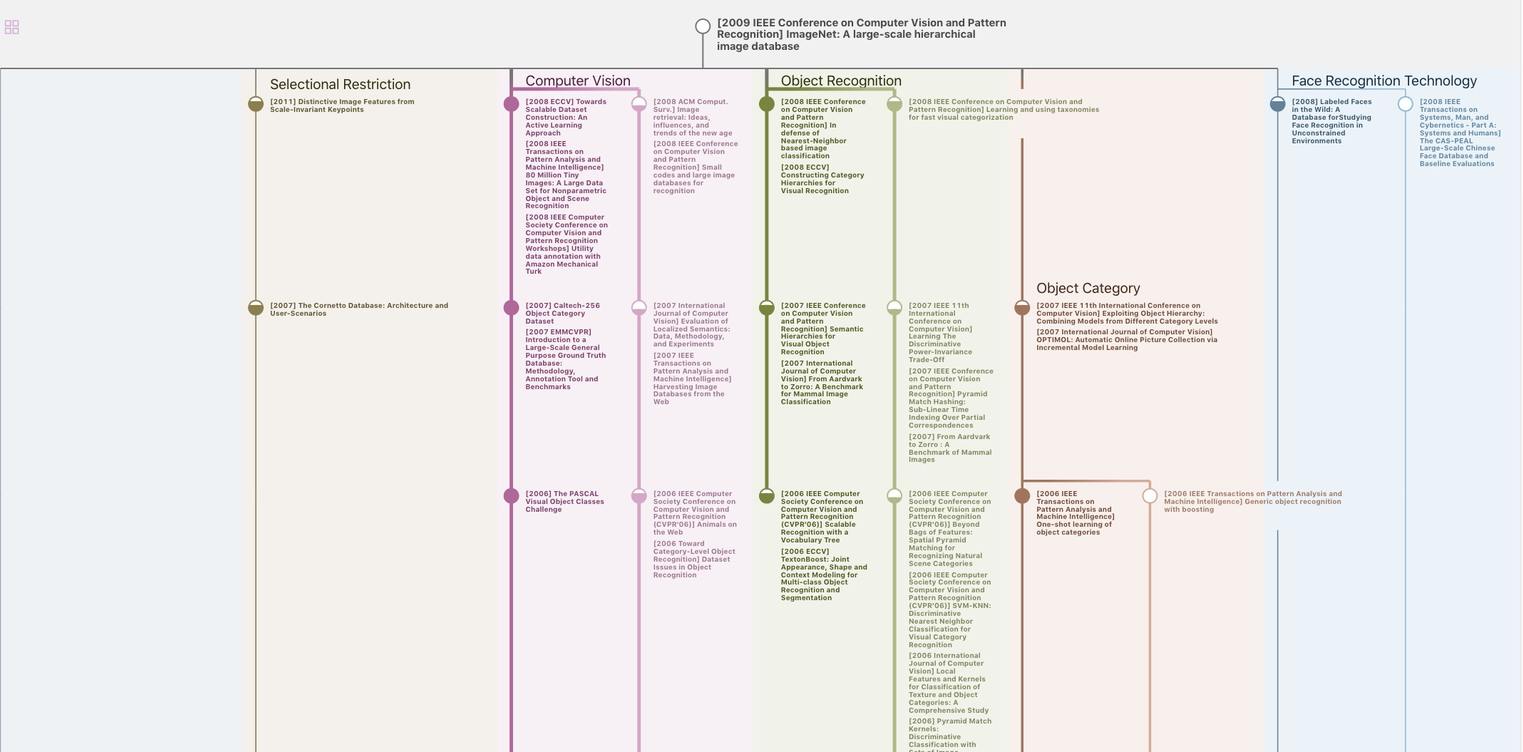
生成溯源树,研究论文发展脉络
Chat Paper
正在生成论文摘要