D2S2BoT: Dual-Dimension Spectral-Spatial Bottleneck Transformer for Hyperspectral Image Classification
IEEE JOURNAL OF SELECTED TOPICS IN APPLIED EARTH OBSERVATIONS AND REMOTE SENSING(2024)
摘要
Hyperspectral image (HSI) classification has become a popular research topic in recent years, and transformer-based networks have demonstrated superior performance by analyzing global semantic features. However, using transformers for pixel-level HSI classification has two limitations: ineffective capture of spatial-spectral correlations and inadequate exploitation of local features. To address these challenges, we propose a dual-dimension self-attention (D(2)SA) mechanism that fully exploits HIS's high spectral-spatial correlation by using two separate branches to model the global dependence of features from the spectral and spatial dimensions. Additionally, we develop a multilayer residual convolution module that extracts local features and introduces shallow-deep feature interactions to obtain more discriminative representations. Based on these components, we propose a dual-dimension spectral-spatial bottleneck transformer (D(2)S(2)BoT) framework for HSI classification that simultaneously models the local interactions and global dependencies of HSI pixels to achieve high-precision classification. By virtue of the D(2)SA mechanism, the introduced (DSBOT)-S-2-B-2 framework can produce competitive classification results with a limited number of training samples on three well-known datasets, which we hope will provide a strong baseline for future research on transformers in the field of HSI.
更多查看译文
关键词
Convolutional neural network (CNN),dual-dimension self-attention (D(2)SA) mechanism,hyperspectral image (HSI) classification,remote sensing,transformer
AI 理解论文
溯源树
样例
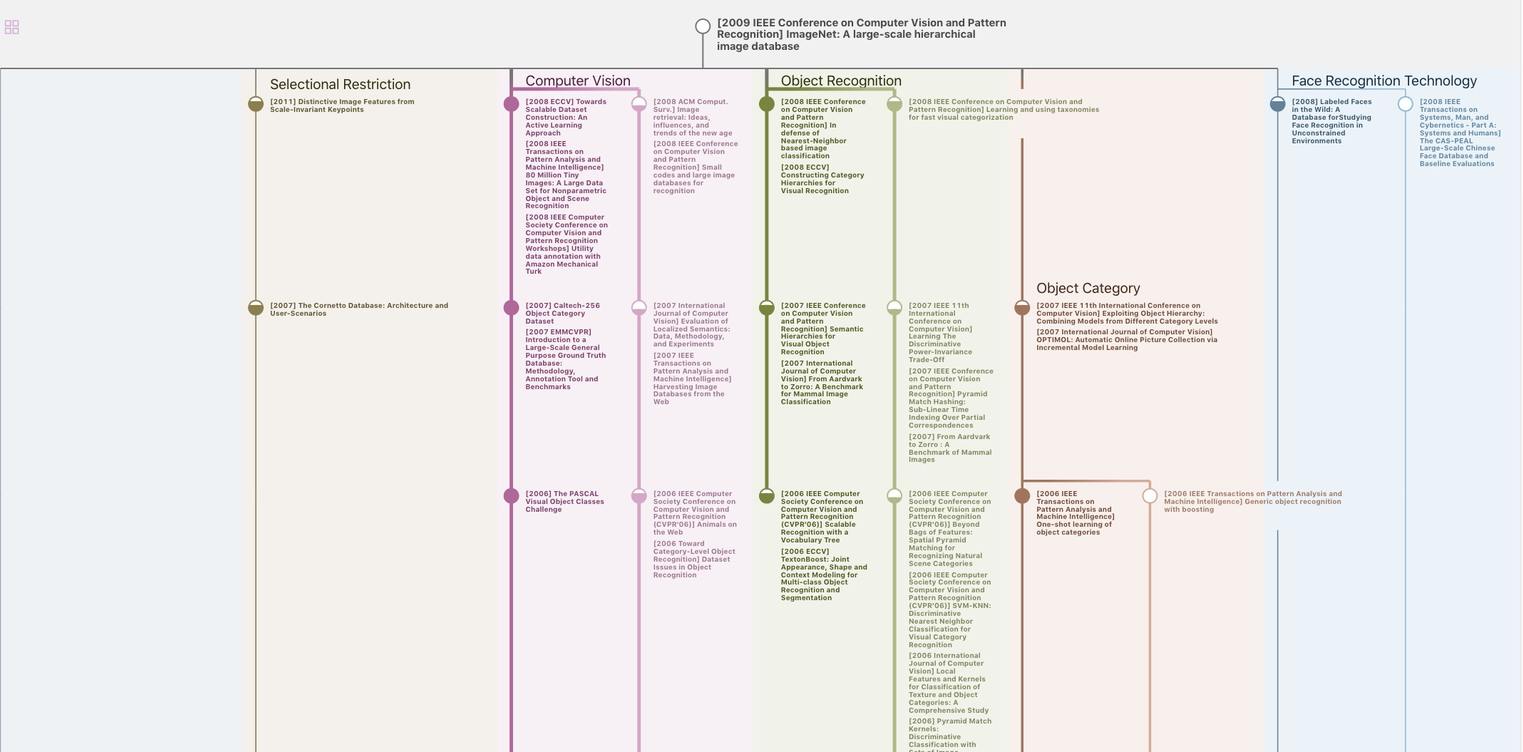
生成溯源树,研究论文发展脉络
Chat Paper
正在生成论文摘要