Estimation of the Concentration of XCO2 from Thermal Infrared Satellite Data Based on Ensemble Learning
ATMOSPHERE(2024)
摘要
Global temperatures are continuing to rise as atmospheric carbon dioxide (CO2) concentrations increase, and climate warming has become a major challenge to global sustainable development. The Cross-Track Infrared Sounder (CrIS) instrument is a Fourier transform spectrometer with 0.625 cm(-1) spectral resolution covering a 15 mu m CO2-absorbing band, providing a way of monitoring CO2 with on a large scale twice a day. This paper proposes a method to predict the concentration of column-averaged CO2 (XCO2) from thermal infrared satellite data using ensemble learning to avoid the iterative computations of radiative transfer models, which are necessary for optimization estimation (OE). The training data set is constructed with CrIS satellite data, European Centre for Medium-Range Weather Forecasts (ECMWF) Reanalysis v5 (ERA5) meteorological parameters, and ground-based observations. The training set was processed using two methods: correlation significance analysis (abbreviated as CSA) and principal component analysis (PCA). Extreme Gradient Boosters (XGBoost), Extreme Random Trees (ERT), and Gradient Boost Regression Tree (GBRT) are used for training and learning to develop the new retrieval model. The results showed that the R-2 of XCO2 prediction built from the PCA dataset was bigger than that from the CSA dataset. These three learning models were verified by validation sets, and the ERT model showed the best agreement between model predictions and the truth (R-2 = 0.9006, RMSE = 0.7994 ppmv, MAE = 0.5804 ppmv). The ERT model was finally selected to estimate the concentrations of XCO2. The deviation of XCO2 predictions of 12 TCCON sites in 2019 was within +/- 1 ppm. The monthly averages of XCO2 concentrations in close agreement with TCCON ground observations were grouped into four regions: Asia (R-2 = 0.9671, RMSE = 0.7072 ppmv), Europe (R-2 = 0.9703, RMSE = 0.8733 ppmv), North America (R-2 = 0.9800, RMSE = 0.6187 ppmv), and Oceania (R-2 = 0.9558, RMSE = 0.4614 ppmv).
更多查看译文
关键词
CrIS,XCO2,PCA,ensemble learning,extremely randomized trees
AI 理解论文
溯源树
样例
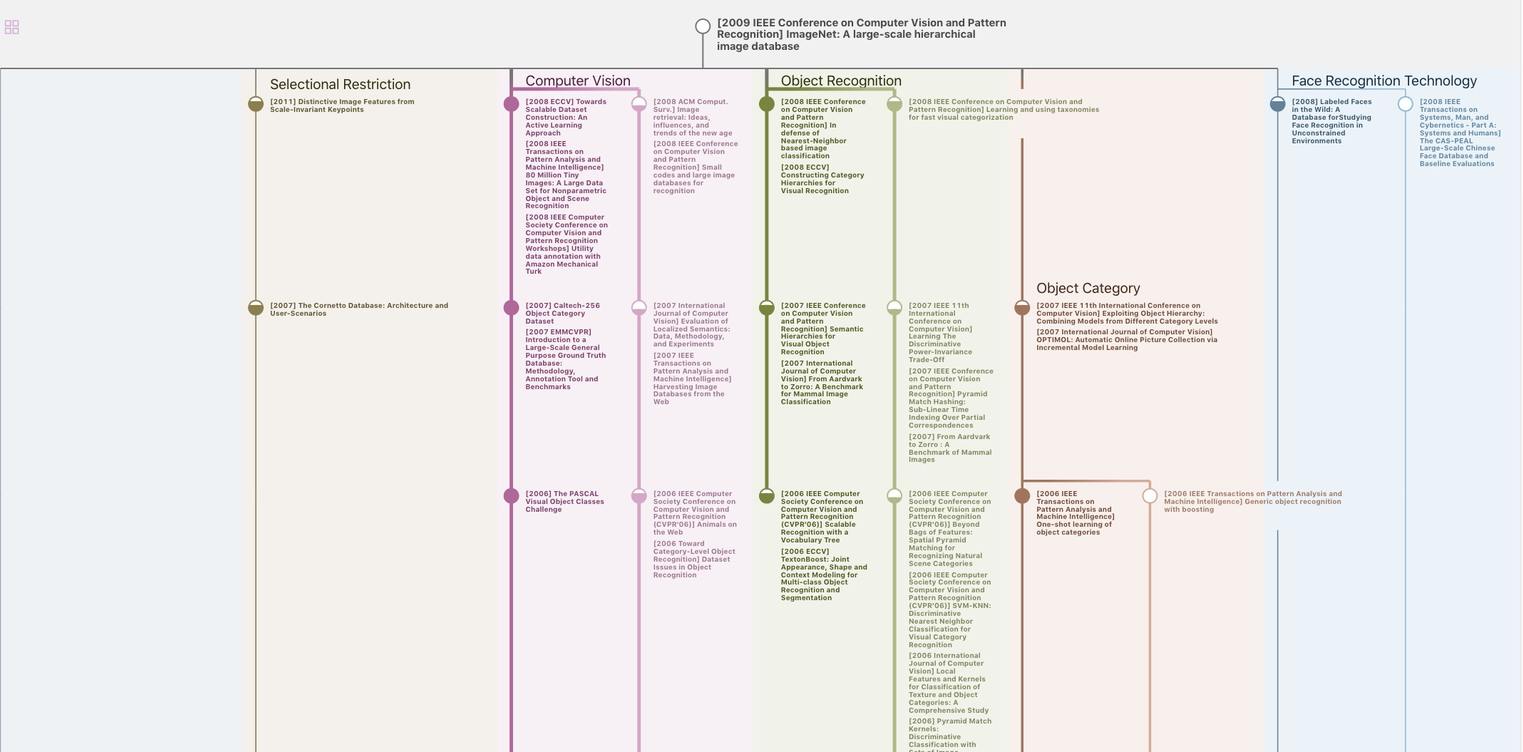
生成溯源树,研究论文发展脉络
Chat Paper
正在生成论文摘要