A survey on model-based reinforcement learning
SCIENCE CHINA-INFORMATION SCIENCES(2024)
摘要
Reinforcement learning (RL) interacts with the environment to solve sequential decision-making problems via a trial-and-error approach. Errors are always undesirable in real-world applications, even though RL excels at playing complex video games that permit several trial-and-error attempts. To improve sample efficiency and thus reduce errors, model-based reinforcement learning (MBRL) is believed to be a promising direction, as it constructs environment models in which trial-and-errors can occur without incurring actual costs. In this survey, we investigate MBRL with a particular focus on the recent advancements in deep RL. There is a generalization error between the learned model of a non-tabular environment and the actual environment. Consequently, it is crucial to analyze the disparity between policy training in the environment model and that in the actual environment, guiding algorithm design for improved model learning, model utilization, and policy training. In addition, we discuss the recent developments of model-based techniques in other forms of RL, such as offline RL, goal-conditioned RL, multi-agent RL, and meta-RL. Furthermore, we discuss the applicability and benefits of MBRL for real-world tasks. Finally, this survey concludes with a discussion of the promising future development prospects for MBRL. We believe that MBRL has great unrealized potential and benefits in real-world applications, and we hope this survey will encourage additional research on MBRL.
更多查看译文
关键词
reinforcement learning,model-based reinforcement learning,planning,model learning,model learning with reduced error,model usage
AI 理解论文
溯源树
样例
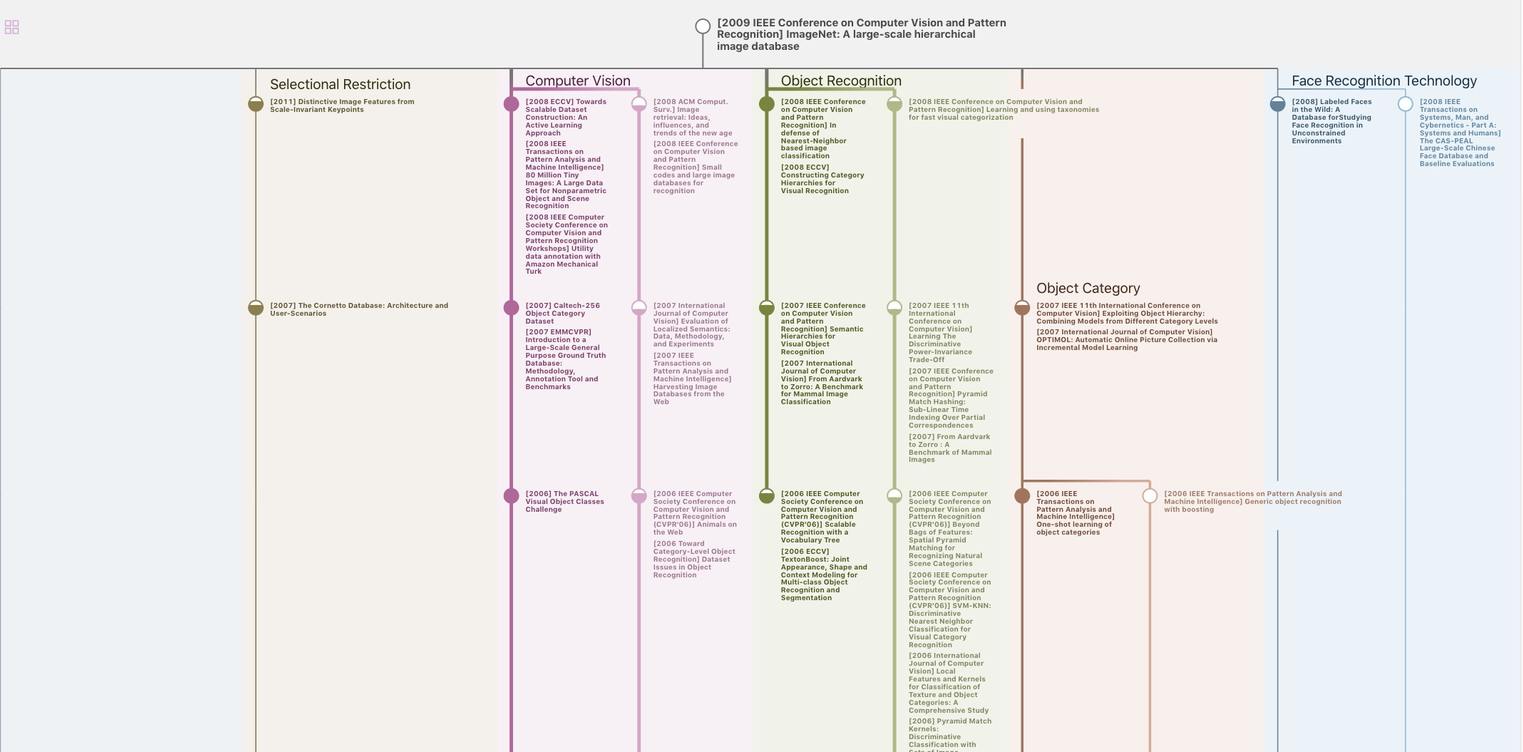
生成溯源树,研究论文发展脉络
Chat Paper
正在生成论文摘要