Learning Provably Stable Local Volt/Var Controllers for Efficient Network Operation
IEEE TRANSACTIONS ON POWER SYSTEMS(2024)
摘要
This paper develops a data-driven framework to synthesize local Volt/Var control strategies for distributed energy resources (DERs) in power distribution grids (DGs). Aiming to improve DG operational efficiency, as quantified by a generic optimal reactive power flow (ORPF) problem, we propose a two-stage approach. The first stage involves learning the manifold of optimal operating points determined by an ORPF instance. To synthesize local Volt/Var controllers, the learning task is partitioned into learning local surrogates (one per DER) of the optimal manifold with voltage input and reactive power output. Since these surrogates characterize efficient DG operating points, in the second stage, we develop local control schemes that steer the DG to these operating points. We identify the conditions on the surrogates and control parameters to ensure that the locally acting controllers collectively converge, in a global asymptotic sense, to a DG operating point agreeing with the local surrogates. We use neural networks to model the surrogates and enforce the identified conditions in the training phase. AC power flow simulations on the IEEE 37-bus network empirically bolster the theoretical stability guarantees obtained under linearized power flow assumptions. The tests further highlight the optimality improvement compared to prevalent benchmark methods.
更多查看译文
关键词
Distributed energy resources,global stability,local control,Volt/Var control
AI 理解论文
溯源树
样例
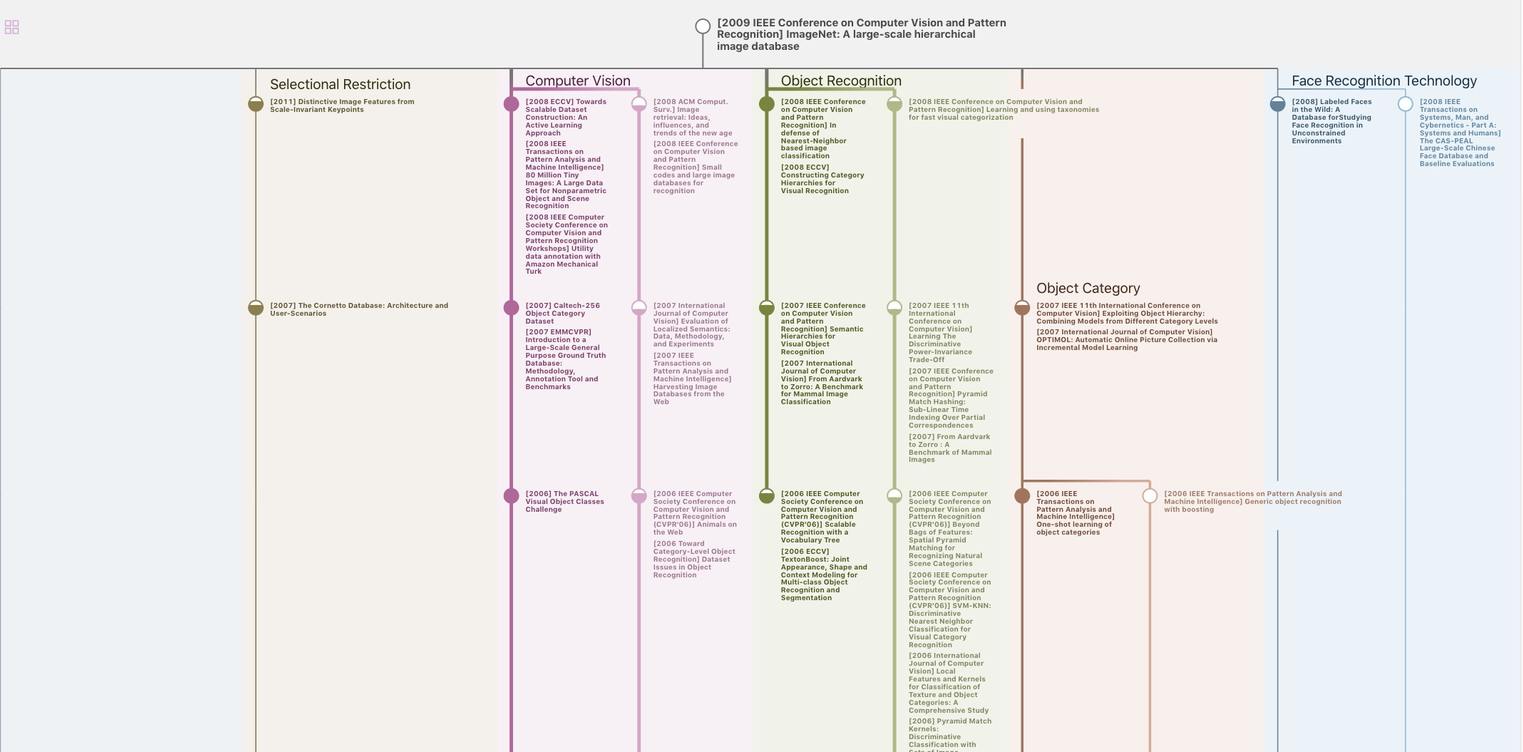
生成溯源树,研究论文发展脉络
Chat Paper
正在生成论文摘要